Data Discovery: Unlocked
Talk Summary - Rent the Runway, Blossom, and Castor discuss data discovery.

Framing the data discovery issue
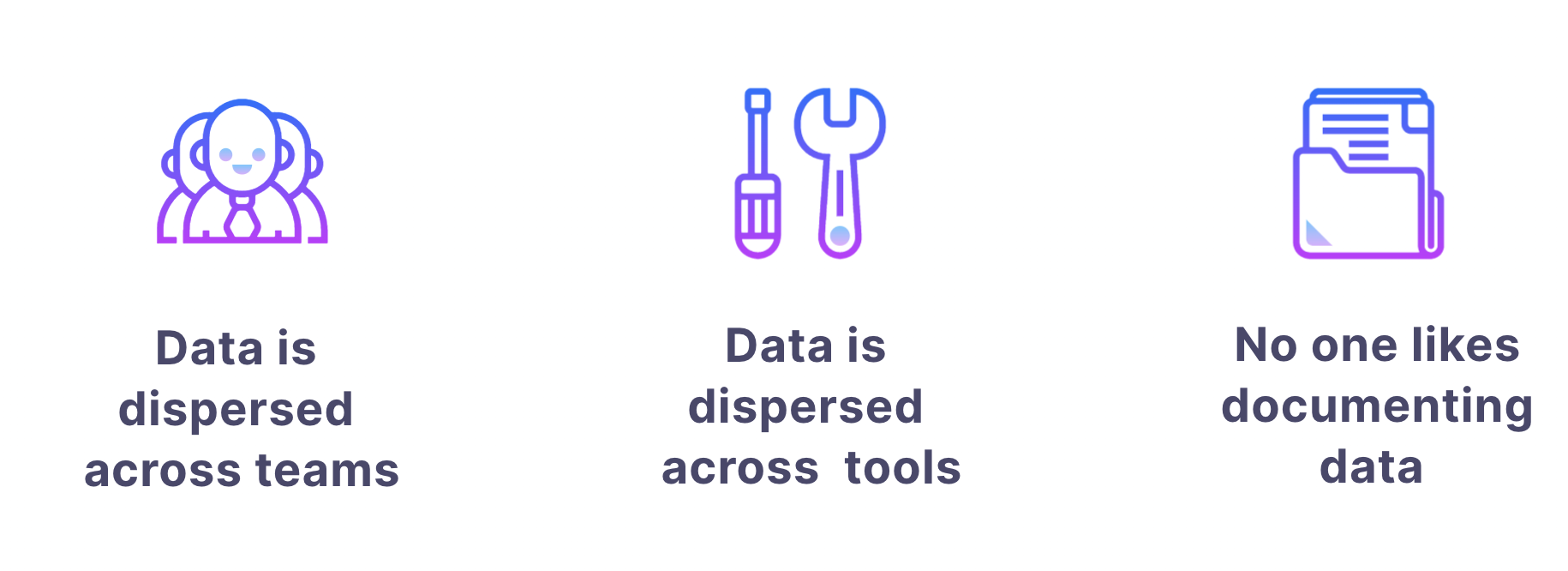
Data Discovery has really become a challenge in the past few years. This is for a few reasons:
- Data volumes have exploded: This is not news to you. In the last decade, most of the innovations in the data ecosystem were focused on producing more data. It worked. Unsurprisingly, data is now in everyone’s hands, and the number of people working with it has blown up in the past few years.
- As the modern data stack has grown, it has become siloed. The number of tools in people’s data stacks has multiplied. Within each tool, there is no transparency around who’s using the data, when the data is being updated, or how trustworthy the data is. This makes everyone’s jobs a lot harder.
- People hate documenting. And you can’t blame them; it’s not the most enjoyable activity. This is the icing on the cake, adding to the problem’s complexity.
Data is thus dispersed across people and departments, but also across tooling. This evolution has made data discovery one of the most complex challenges of our decade. The data discovery issue is affecting everyone; both the data team and stakeholders outside of it, who now rely on data for their daily analysis and operational decisions. This makes the challenge even more pressing to tackle.
We sat down with top data leaders to discuss proven strategies to tackle data discovery. I’ve slightly changed the talk structure in the transcribing process, for flow purposes. This article covers, in the following order:
I - How to identify a discovery issue when there is one.
II - How to choose the most adapted solution to your discovery problem.
a - The benefits of investing in a data discovery tool.
b- Choosing the right discovery tool for your organization.
III - How to ensure the success of your data discovery project.
A short introduction of our speakers:
- Lauren Haag leads Business Intelligence at Rent the Runway. Rent the Runway (RTR) is a US-based clothing rental company. Lauren spent most of her career in data and data adjacent areas, spending some time at some larger companies like Disney, Walmart, and DocuSign. She’s been at Rent the Runway leading the BI Team for a year and a half.
- Arnaud de Turckheim is the CPO of Castor, a data discovery tool. Before that, he was Head of Data at Payfit, an HR tech unicorn, where he built a 15+ people data team from scratch. Prior to this, Arnaud worked in data at Criteo, a retargeting ads business that pioneered product data management.
- Imran Ghory is a VC at a firm that specializes in early-stage investments called Blossom capital. Prior to venture capital, Imran used to run data teams. Imran was the voice behind all the pertinent questions in this talk.
Some context: Lauren, tell us a bit more about how the data team is structured and your function’s role within the company.
Lauren: Data has been a key component of Rent the Runway’s business model and culture for a really long time. We have a sizeable data team of about 40 people, covering many different areas. We have a team of ML engineers, a data engineering team, and several data science teams focusing on particular verticals. Then, we have our Business Intelligence team which I started a year and a half ago. The team started out with one analyst focused on the fulfillment center operations. We grew to support CX transportation, people inventory retention, and growth. The BI team now covers the full span of data at RTR. We have a very complex business model, leading to a lot of interesting data. We use a very common data stack: Snowflake, dbt, Prefect, Looker, and now Castor.
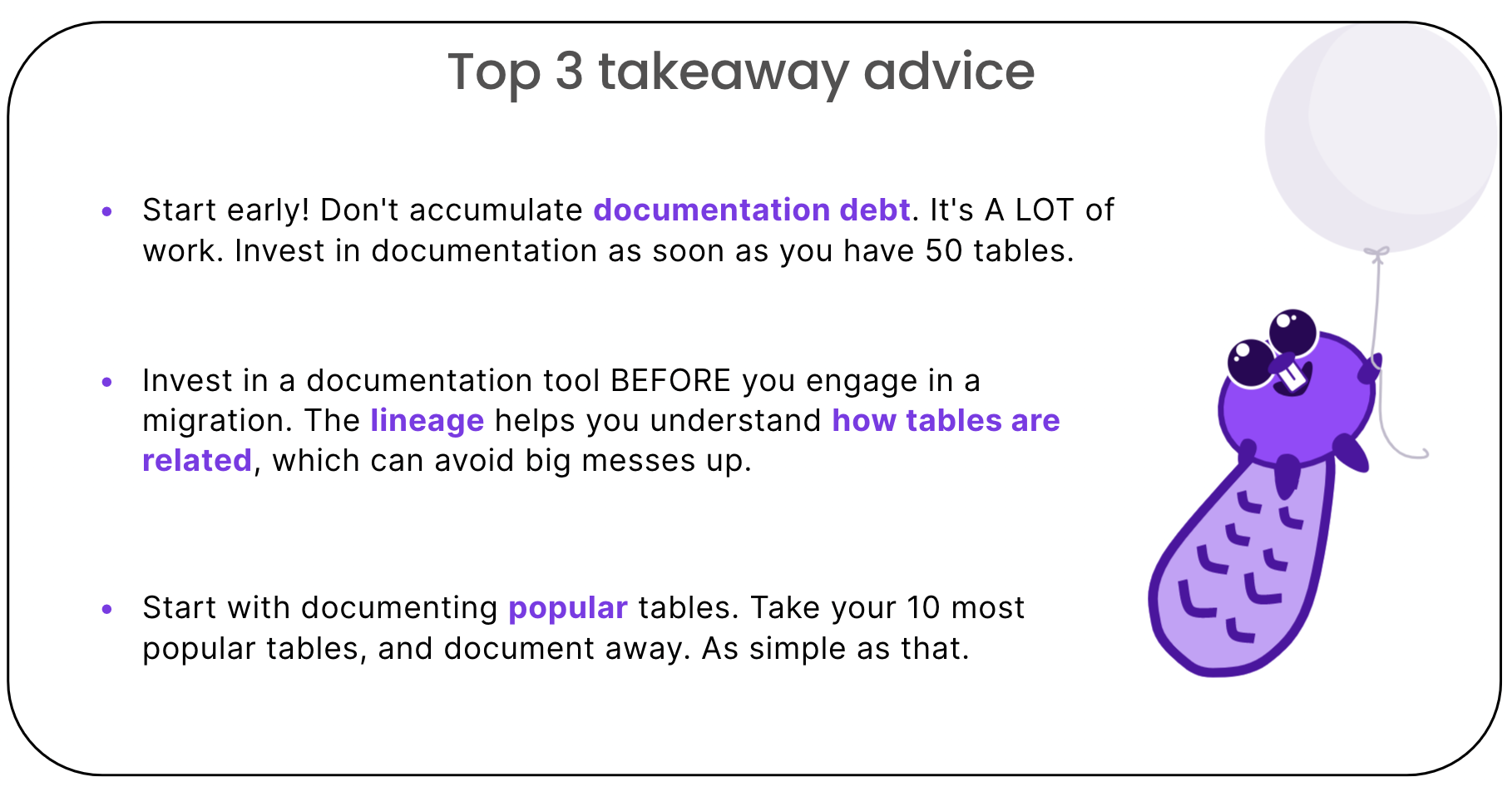
I - Identifying the data discovery issue
A lot of data leaders out there are thinking about data discovery, but often these goals aren’t at the top of the priority list. Can you share what sparked the data accessibility initiative at Rent the Runway and Payfit and what you were looking to solve?
Lauren: When I first joined the company we made everyone at RTR fill out a survey to see how they viewed data accessibility in the company, and we saw that the data team ranked the question “I can find the data I need” lower than we would have anticipated. We would hope that we were all 100%, across the board, we are the data team. But we were really struggling with it. It’s not so surprising regarding the level of complexity of our data, but it's not what we want. This was the wake-up moment, where we realized we had a discovery problem. We are a 15-year-old company, meaning we had 15 years of data documentation debt. We always knew the documentation was important, but the tooling we had in place just wasn't supporting the amount of documentation that we had we really needed to do. We were doing a little in dbt, a little in Confluence a little in Google Sheets. It was really spread out. We needed a way to bring everything together and weave it into people's actual day-to-day development.
Arnaud: At Payfit, the company was already 200 people strong when I joined as the first data hire. Thanks to Stitch, Fivetran, and dbt, we created 500 tables and 100 dbt models. Having tools that can create data so quickly brings about huge benefits, but it also comes to a cost which is that you can create a data mess super quickly. Although I was supervising the data team, other data users were getting involved in lookML, and they were struggling because of the number of tables that had been created. The company was barely 15 years old, yet it was quickly growing into a big mess. It was impossible to answer questions such as “In which table does this column appear? or other simple but useful questions of the sort. That’s when we realized we had a discovery problem.
II - Choosing the right solution
a) Investing in a data discovery tool
Arnaud, what are the challenges you’re now looking to help companies solve with Castor?
Arnaud: A lot of companies have documentation debt. the current solution around is to help data analysts find their way, even without documentation. Our mission is to get started with the first level of support, by enabling teams to document efficiently and build a source of truth containing all the existing documentation. This sets the stage for “social exploration”, meaning the ability for data users to check who’s used what and what they’ve done with it, through the data lineage feature for example. This allows users to understand how data flows and whether they can use a given data asset safely. From day one, Castor aims at improving the data experience, whether there is existing documentation or not, and then showing the way forward using documentation.
Arnaud, beyond tracking documentation, what benefits do you keep in mind when building the product to ensure Castor can be adopted across an organization? For example, how can data leaders think about the impact of things like improved collaboration or efficiency?
Arnaud: The other thing we are heavily focusing on is making people realize that they are part of an internal company or data community. They are not alone, they are not the only users. It is very rare that someone is the first user of a dataset or the first to ask a question about it. This is what makes it so important to leverage collaboration. Castor allows users to explore data sets, understanding which ones are the most used, who are the datasets’ owners, which queries have been performed, which sets have been merged and by whom, etc.. This is much more efficient than engaging in free text search.
b) Choosing the right data discovery tool
Lauren, could you describe the process of picking a data discovery tool? There are a lot of tools out there, so it is not always obvious.
Lauren: I think that what was really interesting about the process of trying to choose a tool here was looking at the data discovery landscape. There were a lot of enterprise tools trying to solve every problem out there. Yet, we had a very specific problem that these companies couldn’t solve in the specific way we needed. What I liked about Castor is that it was angled on the exact problem that we had: field documentation and the table/column lineage concept. So we decided to put our heads down to really document our most popular tables. We chose the tables which had a popularity of “4+” in castor and decided to emphasize the documentation effort on them.
Arnaud. A follow-up question for you - when you think about adoption across a company, how do you ensure a single product can address the needs of so many different types of users? How do you optimize for usability?
Arnaud: I think you can’t solve all user's needs, you need to pick the needs you wish to prioritize. As Lauren said, you need to pick the main use case that you wish to solve. Solving this particular use case will create assets within the tool that will help other users, covering different use cases. Our use case is discovery, not data quality, not data observability, or anything else. Discovery is just making sure that people can find and use autonomously the data they need to do their daily job. It is also a way to provide a smooth entry to business folks in your data stack.
Imran: Lauren, what were the strategies that were key to your progress in your data accessibility journey?
Lauren: Something which also really helped us was the kind of relationship we're able to develop with Castor, I think the ability to have a conversation with a company that like actually cares about data documentation in a very nerdy way was motivating. To me, it was like having the validation that documentation was important. Having folks talking about the way we were structuring dbt, I think that's opened up a lot of really helpful conversations within the data team, by just giving visibility to this metadata that we couldn't see before. So, again, on the strategy side, tracking progress is the most important. And then the other thing was just like having a tool that's helping you answer these questions you have, and filling in your pain points. When I started on the data discovery journey, I did not realize how important table lineage was going to be, and how much efficiency it would unlock for the team.
III - Ensuring the success of your data discovery project
Embarking on a data accessibility journey is much different than succeeding on one. Lauren, what elements did you identify as critical to motivate the team on, and what strategies were key to your progress?
Lauren: I realized going to people asking “can you document these tables” does not work. The key to making this work is to set targets. You have to show people their progress, setting the goal of a 95% documentation threshold. Otherwise, no one wants to spend their Friday afternoon documenting a table. Setting the target and the motivation in place, as well as showing the progress was really important to actually get us going because documentation is always the thing that goes to the bottom of the pile.
Arnaud, I’m sure many of Lauren’s considerations are familiar to you as a former Head of Data yourself. What are some of the common challenges for data leaders that you’ve encountered as you’ve built Castor, and what are your recommendations for solving them?
Arnaud: So I figured, as Lauren mentioned, if you want to increase coverage in documentation, you need to project manage it. You need someone who is internally pushing for data documentation, a sponsor. At Castor, we’ve realized people hate documenting. No one does that proactively and spontaneously. Simply because when you’re documenting a table, you’re not doing it for yourself. Of course! You already know the answer. yet, if everyone gives 5% of their time documenting, everyone ends up saving 20% of their time. Based on that it's super important to improve the discovery without documentation while pushing people to document. Where we help, I believe is by making sure that every piece of the documentation, that is added to Castor has the highest possible impact. We do this through column lineage description propagation. Basically once a column has been documented (= associated with a definition), the definition is propagated to all the columns bearing a similar name in downstream tables. This way, a single definition can be leveraged to document 20 other columns downstream. This feature is extremely popular because, again, people hate documenting so one minute that someone spent documenting, we need to make the most of it.
Lauren, what are some factors you’re weighing when thinking about broader data accessibility in the organization? How are you thinking about balancing accessibility vs support across different types of business stakeholders outside of the data team?
Lauren: We're using Looker as our main tool for making data more accessible across Rent the Runway. Looker is where we document descriptions that are stakeholder facing, that's where we expect people to learn how to self-service their data. That's our business-facing data, and democratization effort, so it's very nice that Castor also links into Looker. And we have that lineage through Looker because that is our business-facing UI. That said we do have some people across RTR who do use Snowflake. And we've been considering granting Castor access to finance, folks products etc. We actually don't want those teams to rely on Snowflake and would prefer they be able to self-service in Looker, with datasets that are more curated for their needs. So I haven't rushed to do that yet. So it's a delicate balance of who should have access.
About us
We write about all the processes involved when leveraging data assets: from the modern data stack to data teams composition, to data governance. Our blog covers the technical and the less technical aspects of creating tangible value from data.
At Castor, we are building a data documentation tool for the Notion, Figma, Slack generation. We designed our catalog software to be easy to use, delightful and friendly.
Want to check it out? Reach out to us and we will show you a demo.
Subscribe to the Newsletter
You might also like
Get in Touch to Learn More
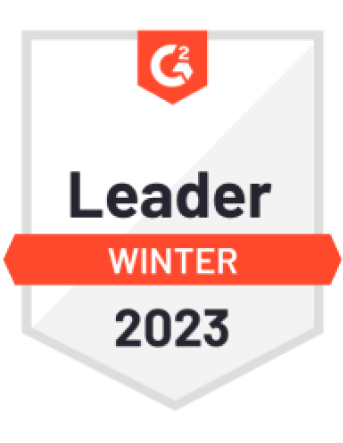
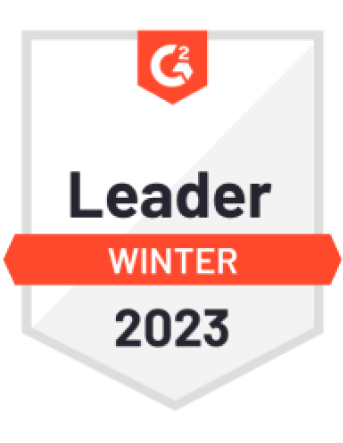
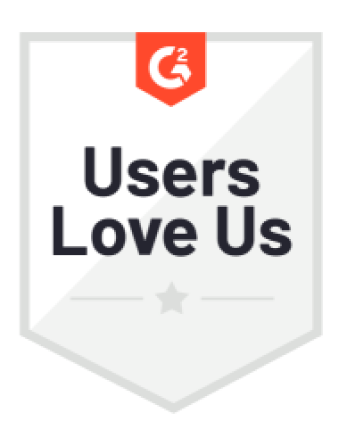
“[I like] The easy to use interface and the speed of finding the relevant assets that you're looking for in your database. I also really enjoy the score given to each table, [which] lets you prioritize the results of your queries by how often certain data is used.” - Michal P., Head of Data