What is a Recommendation Engine?
Recommendation Engines are sophisticated pieces of software that use data to try to understand your behavioral patterns and preferences. Learn more in this blog.

Ever wondered when Netflix unerringly cues up the film that fits your present mood? Or when Amazon almost instinctively suggests the precise product you've been looking for? It's not some sort of magic, but the work of highly efficient recommendation engines.
These are sophisticated pieces of software that understand your behavioral patterns and preferences. They then serve up suggestions you're likely to find interesting.Think of a recommendation engine as a virtual concierge. It knows your taste and preferences, takes them into account, and then offers suggestions that align with your interest.
For instance, research suggests that 80% of Netflix's viewed content comes from its recommendation engine. It's a testament to the influence and effectiveness of a well-oiled recommendation engine.
In this article, we'll discuss what is a recommendation system and how it's changing the way people interact with platforms like Amazon, Netflix, Spotify and more!
What Is a Recommendation Engine?
A recommendation engine is a specialized software that filters and presents items, be it movies, music, or online shopping products. It does so based on a user's behavior, preferences, and past interactions. Think of it as a virtual shopping assistant that knows your taste, offering suggestions you're most likely to enjoy or find useful.
Elements of a recommendation system:
User Generated Data
The core element of a recommendation engine is data, lots & lots of user generated data. It collects vast amounts of information ranging from explicit user inputs like ratings and reviews to implicit behaviors like clicks, page views, and time spent on a page.
Recommendation Algorithm
Once the data is collected, algorithms work their magic to sort and analyze this information. They create a model that can predict your future behavior or preferences with some good accuracy. From suggesting a show similar to somthing you binged watched recently to nudging you towards a pair of shoes that'll match the pants you just bought, the engine uses sophisticated mathematical models to make these predictions.
Real-Time Adaptability
One of the strongest points of a recommendation engine is its ability to adapt in real-time. As you interact more with the platform, the engine fine-tunes its recommendations, ensuring they become increasingly accurate over time.
Types of Recommendation Engines
Collaborative Filtering
- User-Based Similarity: In this filtering method, the engine identifies user profiles who are similar to the target user based on their preferences or behaviors. If User A and User B both like the same movie, it's likely they'll have other interests in common too.
- Item-Based Similarity: Alternatively, the algorithm can also identify items that are similar to each other. If a user likes Item X, the engine might recommend Item Y based on the number of users who liked both.
Content-Based Filtering
- Attribute Analysis: This approach relies on the characteristics of items and a profile of the user’s preferences. If you've shown an interest in mystery novels, for example, the algorithm will look for other books that fit the mystery genre.
- Metadata Use: The algorithm uses detailed metadata about each item—like a book’s author, its genre, or a movie's director—to make more targeted suggestions.
Hybrid Models
- Combined Approach: Hybrid recommendation models merge the strengths of both collaborative and content-based filtering. They might use collaborative filtering to find similar users and then use content-based filtering to recommend specific items to that user group.
- Increased Accuracy: The hybrid model often provides more accurate recommendations, as it's drawing on a broader set of data and techniques.
Context-Aware Models
- Time and Location: These engines take into account additional factors like the time of day or the geographic location of the user. For example, recommending more upbeat songs during workout hours or suggesting nearby restaurants.
- Behavioral Triggers: Some engines also consider short-term behaviors or events, like a sudden interest in baby products possibly indicating a new parent, to tailor recommendations even further.
How Does a Recommendation Engine Work?
The functionality of a recommendation system can be divided into four core stages -
Data Collection
The first phase in the functioning of a recommendation engine is data collection. It's an ongoing process that provides the raw materials for the engine to operate. User behavior data can include what pages someone visited, what they clicked on, or what items they purchased. Preferences might be taken from surveys or direct input, like liking or disliking a product.
Interactions refer to how users engage with the platform, whether they share content, leave a review, or even how long they spend looking at certain items. This data forms a complex tapestry that helps the system understand each user on an individual level.
Data Processing
Once the data is collected, the next step is data processing. This step is all about turning raw data into actionable insights. Data processing involves not just basic analytics but often complex machine learning algorithms that can recognize patterns and analyze user behavior.
The data might be segmented by user demographics, analyzed for seasonal trends, or subjected to a host of other analyses designed to contextualize it. All of these efforts are made to prepare the data for the important task of making recommendations.
Building a Recommendation Model
After the data is cleaned and processed, the next step is building the recommendation model. Recommendation model is like the brain of the engine. Algorithms sift through the processed data to find links between users and items. The type of algorithm used can vary widely depending on the application.
For example, an e-commerce site might use collaborative filtering to suggest items based on what similar users have liked. A news site might use content-based filtering to offer articles similar to those the user has read. These algorithms are fine-tuned to offer the most relevant and personalized suggestions for each individual user.
Continual Learning
The last step involves making sure that the recommendation model keeps on learning. As only it thrives on a continuous feedback loop. When a user interacts with a recommendation on any platform by ignoring it, clicking on it, or giving it a thumbs up, the engine takes note. This feedback helps the system to adjust its future recommendations, making them more accurate and relevant.
It's an ongoing cycle of learning and adjusting, aimed at continuously improving the quality of recommendations. This ability to learn from user feedback and adjust accordingly is one of the most exciting aspects of modern recommendation engines.
Benefits of Recommendation Engines
Recommendation system provide significant advantages to both users and businesses. Here are some of them -
Enhanced User Experience
By providing personalized suggestions, recommendation engines significantly improve the user experience. This personalization makes users feel valued, boosting user satisfaction and engagement.
Increased Conversion Rates
Recommendation engines encourage users to make purchases, leading to higher conversion rates. According to a report from McKinsey, Amazon's product recommendation engine is responsible for 35% of the company's sales (McKinsey).
Improved Customer Insights
The data collected and analyzed by recommendation engines can provide valuable insights into customer behavior. These insights allow businesses to make more targeted marketing strategies and product offerings.
Shaping Business Strategy
The in-depth understanding taken from recommendation engines can strongly shape business strategies. Enterprises can harness this knowledge to finetune their business strategies and products to align with customer predilections. This ultimately leads to fostering enhanced customer experience and great business outcomes.
Recommendation Engines Use Cases
Recommendation engines have become integral to many digital platforms. They're not only prevalent but also highly influential in shaping user experiences.
E-commerce Platforms
- Product Suggestions: One of the most common applications of recommendation engines is in e-commerce platforms where they suggest products to users. Example - Amazon's recommendation engine curates product suggestions that align with your purchasing behavior and browsing history.
- Personalization: They personalize the shopping experience by showing items that are closely related to the user's search history, previous purchases, or viewed items.
- Upsell and Cross-sell: They are adept at upselling and cross-selling by recommending complementary items or higher-value items in the same category.
Streaming Services
- Personalized Playlists: Streaming services like Netflix or Spotify use recommendation engines to create personalized playlists or suggest new shows and movies.
- Genre Matching: They can recognize the genres or types of shows a user typically watches and then suggest similar content.
- Time-Based Suggestions: Some advanced engines even consider what time you usually watch certain types of content and offer suggestions accordingly.
Social Media Platforms
- Friend Suggestions: On platforms like Instagram, these engines recommend new friends or groups based on mutual connections and shared interests.
- Content Curation: They also curate your feed by recommending posts, articles, or videos based on your past interactions.
- Ad Targeting: They can even influence the types of advertisements you see based on your behavior and engagement level.
News Websites
- Article Suggestions: News websites use recommendation engines to suggest articles that are similar to what the user has previously read.
- Topic Interests: If the engine notices a trend in the types of topics a user frequently reads, it will start suggesting articles of the same nature.
- Reading Time Optimization: Some engines can also predict the best time to suggest long-read articles based on a user's previous engagement times with similar content.
Conclusion
To sum up, recommendation engines have become an integral part of our online experiences. They make life easier for both consumers and businesses by providing more personalized, relevant options. As technology advances, expect these engines to become even more accurate and personalized.
You might also like
Get in Touch to Learn More
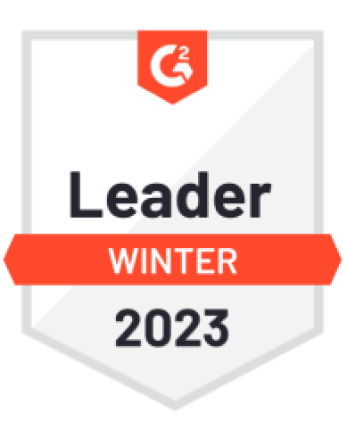
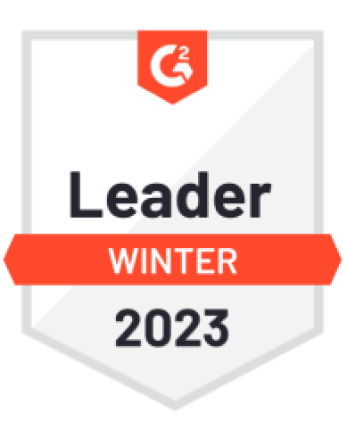

“[I like] The easy to use interface and the speed of finding the relevant assets that you're looking for in your database. I also really enjoy the score given to each table, [which] lets you prioritize the results of your queries by how often certain data is used.” - Michal P., Head of Data