What Is Data Governance Framework?
An Overview!
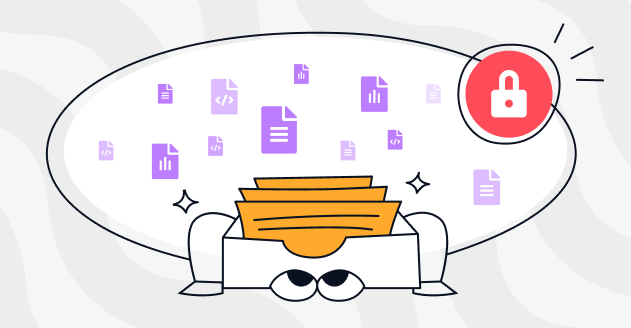
In our data-centric world, a robust "data governance framework" serves as the ship's captain, navigating through the vast sea of information. It's the critical connector linking various elements of data management, guaranteeing top-notch data quality, sturdy security, and smooth usability.
We're geared up to delve deep into this essential topic. We'll demystify its application with a wealth of real-world examples, user-friendly templates, and time-tested best practices. But before we explore the ins and outs of a Data Governance Framework, let's first get to grips with the concept of Data Governance. -
What Is Data Governance?
Data governance forms the strategic backbone of an organization, focusing on enhancing the quality, availability, integrity, security, and usability of its data. This strategy comes to life through a set of practices and principles, collectively creating a robust framework for data management.
The central objective of data governance is building trust in an organization's data assets. To achieve this, organizations lay down clear policies and standards. They outline specific roles and responsibilities.
They also define procedures and metrics for acquiring, maintaining, disposing of, and using data. All these efforts work in unison to cultivate a strong foundation of trust in the organization's data.
What Is Data Governance Framework?
A data governance framework weaves together processes, technologies, roles, rules, and metrics. It forms a holistic system, guiding an organization to handle data effectively, efficiently, and safely. The framework shapes how the organization collects, stores, manages, uses, and protects its data.
This framework isn't a one-size-fits-all solution. Instead, it morphs to accommodate the unique needs and structure of the organization. It considers the organization's goals, the kind of data it manages, and the regulatory landscape it operates within this
The ultimate objective of this customization is to transform data from mere information to valuable assets. It paves the way for insightful decision-making, fosters innovation, and provides a competitive edge.
Pillars of a Data Governance Framework
A comprehensive data governance framework is built on four main pillars.
Data stewardship is the management and oversight of an organization's data assets. Data stewards are responsible for maintaining data quality, enforcing data policies, and safeguarding data integrity. They act as custodians of data, ensuring that it is accurate, consistent, and usable. Their role includes defining data terms, setting up data quality metrics, and implementing data quality rules.
2. Data Quality
The second pillar, data quality, measures the fitness of data to fulfill its role in a particular context. If the data is accurate, reliable, and timely, we consider it of high quality.
An effective data governance framework embeds processes and data governance tools to uphold this quality. It includes methods for data validation and cleaning. It also incorporates practices for data enrichment.
Data privacy and security are crucial components of any data governance framework. This pillar ensures that sensitive data is protected. I also ensure that the organization complies with data protection regulations. It involves setting up security measures, defining data access and usage policies, and educating employees about data privacy and security practices.
The final cornerstone of a data governance framework is data architecture. It pertains to the blueprint and formation of data systems and procedures. It dictates the way data is gathered, stored, transformed, and retrieved.
A well-orchestrated data architecture makes sure that data courses through the organization without hiccups. It ascertains that data is within easy reach and comprehensible and that it's stored in a manner that maximizes its value, all while reducing associated costs and risks.
Why Does Your Organization Need a Data Governance Framework?
Data Governance helps with various Organizational and Business objectives -
Regulatory Compliance
Data regulation has become a significant concern for businesses worldwide, with hefty penalties for non-compliance. GDPR in Europe and CCPA in California, for instance, are comprehensive data protection laws that organizations must comply with. A robust data governance framework helps manage these regulatory requirements efficiently. It ensures that data is collected, stored, and processed in line with legal and ethical guidelines, mitigating the risk of non-compliance and subsequent penalties.
Improved Data Security
In a digital world where data breaches and cyber-attacks are frequent, data security has never been more critical. An effective data governance framework includes strategies for data privacy and security. It helps determine who has access to what data, under what circumstances, and how that data is protected from potential breaches. This not only protects the organization's data assets but also builds trust with customers, stakeholders, and regulators.
Decision-making and Business Intelligence
An often-overlooked benefit of data governance is its role in decision-making and business intelligence. By ensuring data is properly categorized, easily accessible, and trustworthy, a data governance framework lays the foundation for effective data analysis and reporting. Businesses can then derive meaningful insights from their data, leading to better decision-making and a competitive edge in the market.
Streamlined Data Management
Finally, a data governance framework streamlines data management by defining clear roles and responsibilities for data stewards, architects, and other stakeholders. Everyone knows who is responsible for what, which reduces confusion, enhances collaboration, and increases efficiency.
Data Governance Framework Examples
A successful data governance framework blends elements of data quality, data lifecycle management, data privacy, and security.
Take IBM, for example. They've built a sturdy framework with clear roles and responsibilities for managing data. They've integrated data quality metrics into their system and put privacy and security guidelines in place.
Microsoft provides another example. Their data governance framework champions data democratization while upholding strict data privacy and security standards. Through this model, Microsoft empowers its employees to utilize data and simultaneously ensures the data's protection.
Data Governance Framework Template
Here's a detailed outline of a potential data governance framework template:
1. Data Governance Goals and Objectives
This is where you articulate what you hope to achieve with your data governance program. This could range from improving data quality and consistency and ensuring regulatory compliance, to enhancing decision-making processes. Clearly defined objectives will guide the development of your data governance framework and provide a benchmark for assessing its success.
2. Roles and Responsibilities
Define the key stakeholders involved in data governance within your organization and outline their respective roles and responsibilities. This typically includes:
- Data Owners: These are typically senior executives who have overall responsibility for data quality and integrity in their respective business functions.
- Data Stewards: These individuals are responsible for enforcing data governance policies and practices. It helps in ensuring data quality and addressing data-related issues for enterprise data.
- Data Users: These are the individuals who use data in their daily work. They are responsible for adhering to data governance rules and practices.
3. Data Standards and Procedures
This section should detail the rules and processes for data collection, storage, access, and usage within the organization. This may include data naming conventions, data classification schemes, data storage rules, data access policies, and procedures for updating or modifying data.
4. Data Quality Metrics
Specify how you will measure and monitor data quality. This could include metrics such as accuracy, completeness, timeliness, relevance, and consistency. Regularly tracking these metrics will allow you to identify and address data quality issues promptly.
5. Data Security Measures
This section outlines the measures in place to protect data from unauthorized access, breaches, and misuse. This can include access control mechanisms, data encryption practices, and incident response procedures. It should also detail how you will comply with relevant data protection and privacy regulations.
While this template provides a good starting point, it's important to remember that each organization's data governance framework will be unique. Factors like organizational structure, industry regulations, the nature of the data, and the organization's specific data goals will shape the design of the framework. The key is to ensure that the framework is comprehensive, clear, and aligns with the organization's broader strategic objectives.
Best Practices in Building a Data Governance Framework
Establishing a data governance framework isn't a one-size-fits-all endeavor. Yet, there are some best practices that can guide you:
Develop Clear Goals and Objectives
Understand why your organization needs a data governance framework. What problems are you trying to solve? What benefits do you hope to gain? Having clear goals and objectives will help you stay focused and ensure that your framework is aligned with your business needs.
Involve All Relevant Stakeholders
Data governance Initiatives aren't just an IT issue—it affects all parts of the organization. Therefore, all relevant stakeholders should be involved in developing the framework. This includes not only IT professionals but also data users, managers, and executives.
Prioritize Data Quality
A data governance framework is only as good as the data it governs. Therefore, maintaining high-quality data should be a top priority. This includes implementing quality checks and balances, and continuously monitoring and improving data quality.
Build in Flexibility
The world of data is always changing. Your data governance framework should be able to adapt to these changes. This might mean incorporating new data sources, adjusting to new regulations, or accommodating changes in business strategy.
Educate and Train Staff
A data governance framework is only effective if people understand and adhere to it. Regular training and education sessions can help staff understand the importance of data governance and their role in it.
Conclusion
In conclusion, a data governance framework is a critical component in leveraging an organization's data assets effectively. Whether you're a small business or a large corporation, understanding the examples, templates, and best practices outlined here will help you create a robust and effective data governance strategy. Remember, good data governance isn't just about managing data—it's about empowering your organization to make better, data-driven decisions.
Subscribe to the Newsletter
About us
We write about all the processes involved when leveraging data assets: from the modern data stack to data teams composition, to data governance. Our blog covers the technical and the less technical aspects of creating tangible value from data.
At Castor, we are building a data documentation tool for the Notion, Figma, Slack generation.
Or data-wise for the Fivetran, Looker, Snowflake, DBT aficionados. We designed our catalog software to be easy to use, delightful and friendly.
Want to check it out? Reach out to us and we will show you a demo.
You might also like
Get in Touch to Learn More
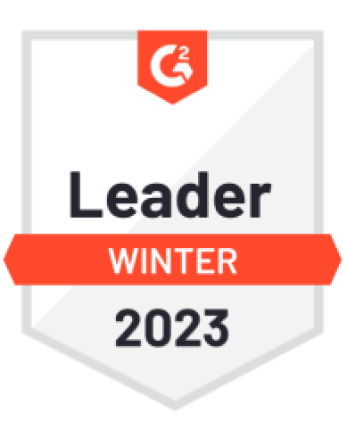
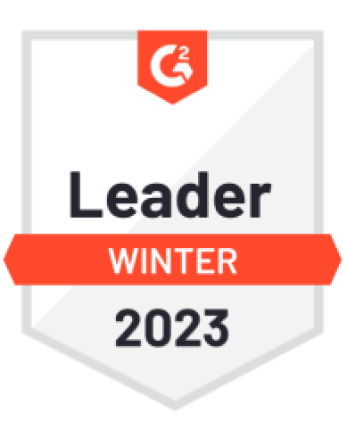

“[I like] The easy to use interface and the speed of finding the relevant assets that you're looking for in your database. I also really enjoy the score given to each table, [which] lets you prioritize the results of your queries by how often certain data is used.” - Michal P., Head of Data