How to Avoid Gaps in Data in Snowflake?
Data is the lifeblood of any business, and ensuring its accuracy and consistency is crucial for decision-making and analysis. In the world of Snowflake, a cloud-based data management and analytics platform, gaps in data can pose significant challenges. These gaps can hinder business intelligence efforts and lead to erroneous insights, ultimately impacting the bottom line. In this article, we will explore the importance of continuous data in Snowflake and discuss strategies to maintain data integrity, identify and address data gaps, ensure data quality, and establish a data governance framework.
Understanding the Importance of Continuous Data in Snowflake
Continuous data is fundamental to the success of any data-driven organization. It refers to the uninterrupted flow of data from various sources into Snowflake, ensuring that all pertinent information is available for analysis in real-time. By having access to up-to-date and complete data, businesses can make informed decisions and gain competitive advantages.
Continuous data in Snowflake enables organizations to monitor their operations and performance in real-time. For example, a retail company can track sales data from multiple stores, online platforms, and marketing campaigns simultaneously. This continuous flow of data allows them to identify trends, spot opportunities, and make data-driven decisions to optimize their business strategies.
Furthermore, continuous data in Snowflake facilitates proactive decision-making. With real-time data, organizations can respond swiftly to changes in the market, customer behavior, or internal operations. This agility gives them a competitive edge by enabling them to adapt quickly and seize opportunities as they arise.
The Role of Data Consistency in Business Intelligence
Data consistency is a critical aspect of business intelligence in Snowflake. It ensures that all data points are accurate, reliable, and synchronized across different systems and platforms. Consistent data guarantees that organizations can trust the insights and reports generated from Snowflake, allowing them to confidently base their decisions on the data at hand.
When data is consistent in Snowflake, it means that all data sources are aligned and synchronized. This alignment eliminates discrepancies and ensures that the information used for analysis is reliable and up-to-date. For example, in a multinational organization, data consistency in Snowflake ensures that sales figures from different regions are accurately consolidated, providing a comprehensive view of the company's performance.
Data consistency also plays a crucial role in data governance and compliance. By maintaining consistent data in Snowflake, organizations can ensure that they adhere to regulatory requirements and industry standards. This adherence is particularly important in industries such as finance and healthcare, where data accuracy and privacy are of utmost importance.
Why Gaps in Data Occur in Snowflake
Gaps in data can occur in Snowflake due to various reasons. Some common causes include technical issues, such as data extraction errors or failures in the data integration process. For example, a network outage or a software glitch during the data transfer can result in missing or incomplete data in Snowflake.
Additionally, human errors can also lead to data gaps in Snowflake. Incomplete or incorrect data entries, whether intentional or unintentional, can introduce gaps in the dataset. For instance, if a data analyst mistakenly enters the wrong sales figures for a specific period, it can create a gap in the data, leading to inaccurate analysis and decision-making.
Furthermore, gaps in data may arise from changes in the source systems or delays in data transmission. When organizations update their data infrastructure or migrate to new systems, there is a possibility of data gaps during the transition. Similarly, delays in data transmission from source systems to Snowflake can result in temporal gaps, where the data is not available in real-time.
It is crucial for organizations using Snowflake to have robust data monitoring and quality assurance processes in place. These processes can help identify and rectify data gaps promptly, ensuring the integrity and reliability of the data stored in Snowflake.
Strategies for Maintaining Data Integrity in Snowflake
To prevent and address data gaps in Snowflake, organizations must implement strategies that prioritize data integrity. These strategies involve regular data audits and utilizing Snowflake's built-in data validation tools.
Implementing Regular Data Audits
Regular data audits are essential for ensuring data integrity in Snowflake. By periodically examining the data pipelines, organizations can identify and rectify any gaps or discrepancies. Data audits involve verifying the accuracy and completeness of data, validating data transformations, and ensuring that all relevant data sources are connected and functioning properly.
During a data audit, organizations can also analyze the data quality metrics to gain insights into the overall health of their data. This analysis can include examining data completeness, consistency, and timeliness. By identifying any data gaps or inconsistencies, organizations can take corrective actions to maintain the integrity of their data.
Furthermore, data audits provide an opportunity for organizations to review their data governance policies and procedures. This includes assessing data access controls, data retention policies, and data privacy measures. By ensuring that these policies are in place and enforced, organizations can enhance data integrity and protect sensitive information.
Utilizing Snowflake's Built-In Data Validation Tools
Snowflake provides a range of built-in data validation tools that can help organizations validate their data, identify issues, and minimize the occurrence of data gaps. These tools, such as automatic data quality checks and data profiling capabilities, allow businesses to establish comprehensive data validation processes that ensure the consistency and accuracy of their data.
One of the key features of Snowflake's data validation tools is the ability to perform automated data quality checks. These checks can be configured to run at regular intervals or triggered by specific events, such as data ingestion or transformation processes. By setting up these checks, organizations can proactively identify and address any data gaps or anomalies, ensuring that the data remains accurate and reliable.
In addition to automated data quality checks, Snowflake also offers data profiling capabilities. Data profiling allows organizations to gain a deeper understanding of their data by analyzing its structure, content, and relationships. By profiling the data, organizations can identify any data anomalies or outliers that may indicate data gaps or inconsistencies. This information can then be used to take corrective actions and improve data integrity.
Furthermore, Snowflake's data validation tools can be integrated with other data management and governance solutions. This integration allows organizations to leverage additional capabilities, such as data lineage tracking, data cataloging, and data masking. By combining these tools, organizations can establish a comprehensive data integrity framework that covers all aspects of data management and governance.
In conclusion, maintaining data integrity in Snowflake requires a proactive approach that includes regular data audits and the utilization of Snowflake's built-in data validation tools. By implementing these strategies, organizations can ensure the consistency, accuracy, and reliability of their data, enabling them to make informed decisions and drive business success.
Techniques for Identifying and Addressing Data Gaps
Recognizing the signs of incomplete data and promptly addressing any gaps are crucial steps in maintaining data integrity in Snowflake. Organizations can adopt techniques and best practices to identify and rectify data gaps.
Recognizing Signs of Incomplete Data
There are several indicators that can help organizations identify potential data gaps in Snowflake. These include missing data points, incomplete records, unexpected variations or outliers in the data, and discrepancies between different data sources. By monitoring these signs, organizations can proactively identify data gaps and take necessary actions to resolve them.
Steps to Rectify Data Gaps in Snowflake
Once data gaps are identified, it is essential to take prompt action to rectify them in Snowflake. This may involve retrieving missing data from the source systems, correcting data errors or inconsistencies, and implementing data integration processes that minimize delays and interruptions. Additionally, organizations can leverage Snowflake's data loading capabilities to efficiently load and update missing or incomplete data in a timely manner.
Ensuring Data Quality in Snowflake
Data quality is paramount in Snowflake to maximize the value derived from data-driven insights and analytics. Poor data quality can lead to erroneous conclusions, unreliable forecasts, and flawed decision-making. Therefore, organizations must strive to maintain high data quality standards.
The Importance of Data Quality Management
Data quality management encompasses the processes and practices that organizations employ to ensure the accuracy, completeness, and consistency of their data. It involves establishing data quality standards, implementing data validation and cleansing procedures, and continuously monitoring data for any anomalies or gaps. By prioritizing data quality management, organizations can instill confidence in the accuracy and reliability of the data used for business intelligence in Snowflake.
Best Practices for Data Quality in Snowflake
To achieve and maintain data quality in Snowflake, organizations should adhere to best practices. These include establishing data governance policies, implementing data validation rules and constraints, conducting regular data quality assessments, and investing in data quality tools and technologies. By adopting these best practices, organizations can ensure that their data is accurate, reliable, and fit for purpose.
The Role of Data Governance in Avoiding Data Gaps
Data governance plays a pivotal role in preventing and mitigating data gaps in Snowflake. It provides a framework for establishing policies, procedures, and responsibilities related to the management and use of data within an organization.
Establishing a Data Governance Framework
To avoid data gaps, organizations should establish a robust data governance framework that defines clear roles, responsibilities, and accountability for data management. This framework should encompass data ownership, data quality standards, data lifecycle management, and data security measures. By having a well-defined data governance framework in place, organizations can prevent data gaps and ensure the accuracy and completeness of the data stored in Snowflake.
How Data Governance Helps Avoid Data Gaps in Snowflake
Data governance helps avoid data gaps in Snowflake by enforcing data quality standards, ensuring compliance with regulations and industry standards, and facilitating effective data stewardship. It enables organizations to have better control over their data assets, minimize the risk of data gaps, and promote data-driven decision-making across the organization.
In conclusion, gaps in data can have significant implications for organizations using Snowflake. By understanding the importance of continuous data, implementing strategies for maintaining data integrity, identifying and addressing data gaps, ensuring data quality, and establishing a data governance framework, organizations can effectively avoid gaps in data and harness the power of Snowflake for actionable insights and informed decision-making.
Get in Touch to Learn More
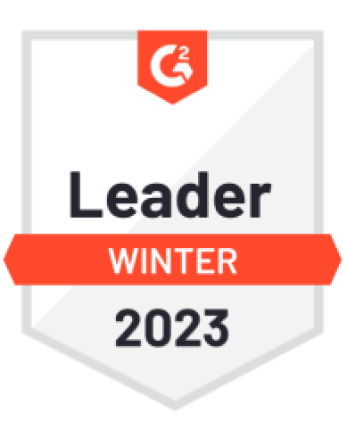
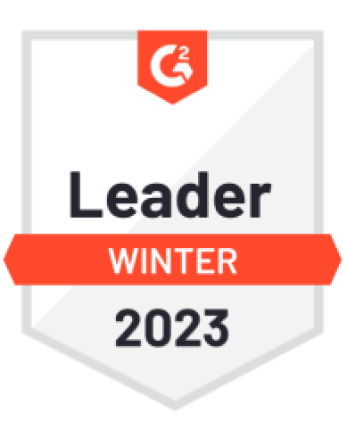

“[I like] The easy to use interface and the speed of finding the relevant assets that you're looking for in your database. I also really enjoy the score given to each table, [which] lets you prioritize the results of your queries by how often certain data is used.” - Michal P., Head of Data