How to use not equal in Snowflake?
Discover the secrets of using "not equal" in Snowflake with this comprehensive guide.
Snowflake is a powerful data warehouse platform that has gained popularity due to its flexibility and scalability. To fully utilize the capabilities of Snowflake, it is essential to understand various operators available in the query language, including the not equal operator. In this article, we will delve into the basics of Snowflake and explore the syntax, usage, and tips for effectively using the not equal operator.
The Importance of Not Equal Operator in Snowflake
Among the various operators available in Snowflake's query language, the not equal operator (!=) plays a crucial role in data analysis. It allows users to filter data based on inequality conditions, making it an invaluable tool for extracting specific subsets of information from large datasets.
When working with large datasets, it is often necessary to narrow down the focus of analysis to specific criteria. The not equal operator enables data analysts to identify and analyze data points that do not meet these criteria. By applying the not equal operator, analysts can filter out irrelevant or unwanted data, enabling them to focus on the data that matters the most for their analysis or reporting.
Role of Not Equal Operator in Data Analysis
The not equal operator is particularly useful in data analysis as it allows analysts to easily identify and exclude data points that do not meet specific conditions. For example, suppose a company wants to analyze customer feedback data but only wants to focus on negative feedback. By using the not equal operator, analysts can filter out all the feedback that is not negative, allowing them to concentrate solely on the information that is relevant to their analysis.
Furthermore, the not equal operator can be combined with other operators to create complex filtering conditions. For instance, analysts can use the not equal operator in conjunction with the greater than operator to filter out data points that are not only different but also greater than a certain value. This flexibility allows for precise and targeted data analysis, ensuring that only the most relevant information is considered.
How Not Equal Operator Enhances Data Filtering
The not equal operator works by comparing the values of two expressions and returning true if the values are not equal. In Snowflake, this operator can be used in conjunction with other operators, such as greater than (>), less than (<), or logical operators (AND, OR) to create complex filtering conditions.
For example, suppose a retail company wants to analyze sales data for products that are not equal to zero. By using the not equal operator, the company can easily filter out all the products with zero sales, allowing them to focus solely on the products that have generated revenue.
Additionally, the not equal operator can be used to filter data based on multiple conditions. Analysts can combine the not equal operator with logical operators like AND or OR to create more sophisticated filtering conditions. This allows for advanced data analysis, where analysts can specify complex criteria to extract the exact information they need.
In conclusion, the not equal operator in Snowflake's query language is a powerful tool for data analysis. It enables analysts to filter data based on inequality conditions, allowing them to focus on the most relevant information for their analysis. By combining the not equal operator with other operators, analysts can create complex filtering conditions, enhancing the precision and accuracy of their data analysis.
Syntax and Usage of Not Equal in Snowflake
Let's dive into the syntax and usage of the not equal operator in Snowflake. The not equal operator can be applied to various data types, including numeric, string, or date/time values.
Breaking Down the Not Equal Syntax
The syntax for using the not equal operator in Snowflake is straightforward:
column_name != value
In the above syntax, column_name represents the name of the column or expression being compared, and value represents the value to compare against.
Common Usage Scenarios for Not Equal Operator
The not equal operator can be used in a wide range of scenarios. Some common usage scenarios include:
- Filtering out outliers or anomalies in datasets
- Identifying missing or incomplete data
- Comparing values across different time periods or groups
- Segmenting data based on specific criteria
Keep in mind that the not equal operator can be combined with other operators or used in conjunction with aggregate functions for more advanced data analysis.
Tips for Using Not Equal Operator in Snowflake
While using the not equal operator in Snowflake, it's essential to be mindful of potential pitfalls and best practices to ensure accurate results and optimize performance.
Avoiding Common Mistakes with Not Equal Operator
One common mistake when using the not equal operator is dealing with null values. Keep in mind that comparing a value with a null value using the not equal operator will result in an unknown or null outcome. To handle null values, consider using the IS NOT NULL operator in combination with the not equal operator.
Best Practices for Using Not Equal in Snowflake
Here are some best practices to follow when using the not equal operator in Snowflake:
- Ensure consistent data types: Make sure the data types of the values being compared are compatible. Mismatched data types can lead to unexpected results.
- Optimize query performance: Indexing columns frequently used in not equal comparisons can significantly enhance query performance.
- Use parameterized queries: Utilize bind variables when working with dynamic values to prevent SQL injection attacks and enhance query reusability.
Troubleshooting Not Equal Operator Issues in Snowflake
Despite its simplicity, issues can arise when working with the not equal operator in Snowflake. Let's explore some common problems and their solutions.
Identifying Common Not Equal Operator Errors
One common error when using the not equal operator is mistyping the operator itself. Make sure to use the correct syntax, !=, instead of mistyping it as =! or <>.
Solutions for Not Equal Operator Issues
If you encounter issues while using the not equal operator, ensure that the columns being compared have the same data type. Additionally, double-check for any missing or incorrect values that might affect the comparison. Refer to the official Snowflake documentation or consult the Snowflake community for specific troubleshooting steps.
In conclusion, understanding and effectively using the not equal operator in Snowflake can greatly enhance your data analysis capabilities. By leveraging this powerful operator, you can filter and extract valuable insights from your data, ultimately empowering better decision-making within your organization.
Understanding the Basics of Snowflake
Overall, Snowflake's unique features and advanced functionalities make it a powerful and versatile data warehousing solution. Its scalability, separation of compute and storage, and zero-copy cloning capabilities provide organizations with the flexibility and efficiency they need to handle large datasets and drive data-driven insights.
CastorDoc is an AI assistant powered by a Data Catalog, leveraging metadata to provide accurate and nuanced answers to users. Our SQL Assistant streamlines query creation, accelerates debugging, and ensures your queries are impactful and enduring—no matter your skill level. Elevate your SQL game - Try CastorDoc today.
Get in Touch to Learn More
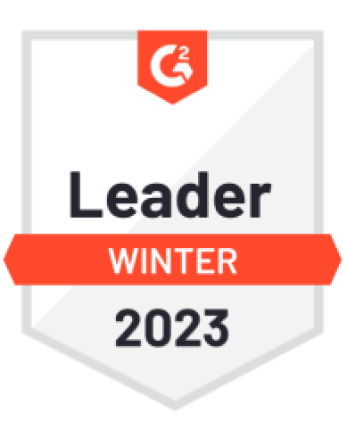
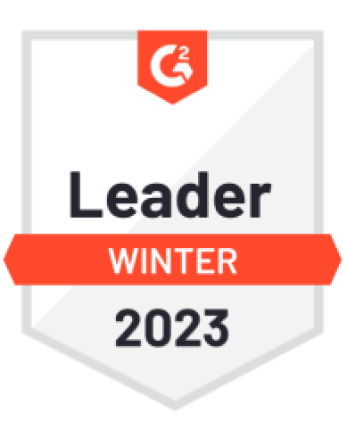

“[I like] The easy to use interface and the speed of finding the relevant assets that you're looking for in your database. I also really enjoy the score given to each table, [which] lets you prioritize the results of your queries by how often certain data is used.” - Michal P., Head of Data