Most common data analysis methods
Learn how to turn data into actionable insights no matter where it comes from.

Businesses now have access to a veritable mine of data. Every day, people use their smartphones, computers, IoT-enabled appliances, and other devices to create this data. Today, one of the most important factors in a company's success is its ability to assess and derive actionable meaning from data.
Although this post will focus on data analysis from a business perspective, the underlying scientific and statistical concepts necessary for doing the job properly will also be covered in detail.
Accenture predicts that by 2025, the world will have amassed 175 zettabytes of data (that's 21 zeroes!), yet businesses will still be unable to capitalize on this information fully. Only 32% of businesses are able to get economic value from their data now, according to that same report.
So, what is data analysis, and why is it so important for businesses? This article answers those questions, and we also take a look at some of the common methods for data analysis.
What is data analysis?
By cleaning, modifying, and processing raw data to obtain useful information, data analysis enables organizations to make educated decisions. It provides helpful insights, metrics, and statistics, generally shown in charts, tables, and graphs, that can be used to inform decision-making.
Every time we weigh the results of the past before making a decision, we perform a basic form of data analysis. In a nutshell, it’s the process of selecting a choice based on an examination of previous events.
Why is data analysis important?
Data analytics methods can be applied to any kind of data to gain understanding that can be put to good use. With the help of data analytics, previously hidden patterns and metrics can be uncovered. Afterwards, this data can be used to fine-tune operations and enhance a company’s overall performance.
But there are other reasons why data analytics is important for businesses. Here are the biggest benefits:
1. Reduce costs
Technology advancements like predictive analytics help companies see where they can make improvements by analyzing their data for patterns and trends. Spending less time and money on ineffective techniques is a long-term benefit. As an added bonus, you can plan for production and supply by thinking ahead to various circumstances, such as sales and demand.
2. Understand prospective and current customers better
By analyzing prospective customer data, companies can learn more about their demographics, purchasing power, and potential markets. This information is useful for many business decisions, including pricing, advertising, and inventory planning.
Furthermore, data analysis also allows you to understand your existing customers better, which in turn can improve their experiences with your company. For example, in a digital product business, data analysis can identify where users get stuck in their onboarding journey, which in turn could help the product team create a more seamless experience. Or, to take an example from a brick-and-mortar business, data analysis on retail store traffic volume could inform staffing decisions, and ensure customers don’t encounter super long lines. No matter the industry, data analysis can surface valuable insights to drive action.
3. Make informed decisions
Managers can profit from data analysis because it allows them to base their judgments on hard evidence rather than guesswork. Analytics can help inform where to put your money, spot potential for expansion, estimate future earnings, and deal with unforeseen challenges. With data analysis, you can glean useful data from every corner of your business and display insights to various stakeholders in a polished and engaging manner with the use of a dashboard or more sophisticated BI tooling.
Most common methods in data analysis
Data analysis allows analysts to transform a mountain of information into useful knowledge. Different methods of data analysis play a crucial role in translating research findings into actionable intelligence that may inform strategic business decisions. Data analysis is a key ability for creating corporate value since it may lead to increased income, enhanced marketing and operational performance, and strengthened customer relationships.
Data analysis methods are generally split into two broad categories:
1. Quantitative analysis
Quantitative data analysis involves working with numerical variables, such as statistics, percentages, calculations, measurements, and other numbers. Quantitative data analysis techniques often involve working with software to manipulate data and find insights that show the business value.
When to use quantitative methods
Quantitative methods can help business owners predict trends, decide how to use their resources, and run projects. Investing decisions are also made with the help of quantitative methods. To put it in an obvious way: you should use quantitative methods when you have quantitative data to work with, given the objective nature of it. However, quantitative analysis can often be paired with qualitative analysis to drive even more insight. Which leads me to my next point…
2. Qualitative analysis
Qualitative data is more subjective and usually describes things that can't be measured in numbers. In qualitative data analysis, a data analyst might use first-hand or participant observation, interviews, focus groups, or the review of documents and artifacts.
When to use qualitative analysis
Several business processes can benefit from qualitative data analysis. For example, techniques for analyzing qualitative data are often used as part of the process of making a product. Focus groups and interviews can be used to help inform prioritization of what new features a product might come out with in future updates.
Different quantitative analysis methods
Descriptive analysis
Descriptive analysis, which is also called "univariate analysis," helps you sum up and find patterns in data from the past. Analytical introspection always begins with a descriptive analysis, which seeks to answer the question, "what happened?" In order to accomplish this, it sorts, transforms, and analyzes raw data gathered from a variety of sources to provide actionable intelligence.
Descriptive analysis is crucial because it helps us communicate our findings clearly. This analysis will leave your data organized and ready to undertake further investigations. Still, it will not help businesses to forecast future events or answer questions like why something happened.
Inferential analysis
Inferential analysis is also called bivariate or multivariate analysis. It uses samples made from a complete set of data which is used to figure out how different factors affect each other so that conclusions can be drawn and predictions can be made. Most of the time, correlation, regression, and analysis of variance are used to draw conclusions from data.
Different qualitative analysis methods
Content analysis
Analyzing content across various formats (text, image, video, audio) can help you understand its context, meaning, and impact.
Whether the data comes from books, movies, or even real objects, it can be analyzed with content analysis. Research questions should be taken into account ahead of deciding to apply this analytical strategy.
Discourse analysis
Discourse analysis examines communication between individuals. It puts a premium on understanding the social setting in which the subjects interacted. The everyday context of the respondent is also taken into account in discourse analysis.
Using discourse analysis allows you to build a comprehensive and detailed understanding of your brand's ability to maintain relevance, stand out, and mean more to the people who matter to your organization within its competitive landscape.
Narrative analysis
Similar to discourse analysis, this technique is used to examine data collected through surveys, interviews, and in-field observations. But rather than relying solely on surveys or experiments, it makes use of people's own anecdotes and experiences to draw conclusions.
By analyzing narratives, we can learn more about the larger cultural forces at work in shaping stories and the ideas those stories reflect.
Grounded theory
To create hypotheses and test them in practice, qualitative researchers often use grounded theory analysis. Hypotheses and theories are developed via the systematic examination of qualitative information.
This approach reverses the usual order of qualitative data analysis procedures by working backwards, and developing ideas as a result of the collected information. Software developers, product marketers, managers, and others who work with data regularly employ grounded theory analysis in their work. When it comes to managing employees, this strategy might be useful as well.
Final thoughts
In data analytics, the focus is both on a company's end user and its own internal operations. Because of its usefulness in guiding decision-making and guiding the development of company strategies, business analytics has emerged as a critical component of any modern business's approach to the market.
Data analytics aids people and businesses in verifying their data in a world where reliance on information and statistics is growing. A collection of raw numbers can be converted into insightful, instructional insights that drive decision-making and careful management using a range of tools and methodologies.
Subscribe to the Castor Blog
You might also like
Get in Touch to Learn More
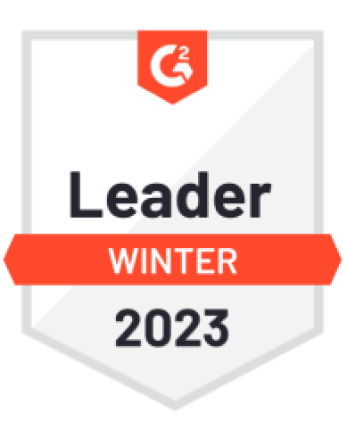
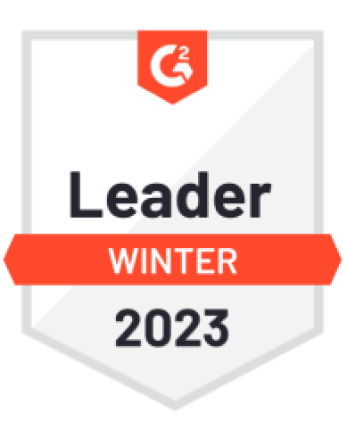

“[I like] The easy to use interface and the speed of finding the relevant assets that you're looking for in your database. I also really enjoy the score given to each table, [which] lets you prioritize the results of your queries by how often certain data is used.” - Michal P., Head of Data