What is Analytics Engineering?
The emergence of a new role at a crossroads between business and engineering
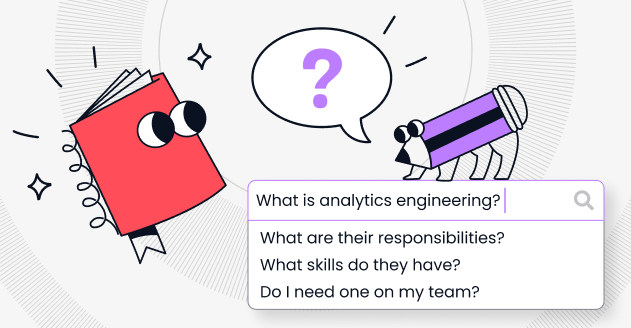
As you read the title, you probably thought: “Great, and another job hits the stack: what on earth is an Analytics Engineer now?”
You almost kept scrolling, but if you made it up to here, it’s probably because the weird juxtaposition of “analytics” next to “engineer” caught your attention. And rightly so: for many of us analysts are not exactly highly technical engineering roles and vice versa. But let’s face it: the world has jumped forward and today, Python, R and SQL are basic requirements to many jobs. The worlds of engineering and analytics are slowly merging to become one, and today we want to discuss the intersection of both areas: here sits the Analytics Engineer.
And yes, we agree with you, these days it seems that any excuse is a good one to create “new, essential tech jobs”, making it all so overwhelming to keep track of, but more seriously it also gives the impression that these roles are all very much Fugazi, Foogazi
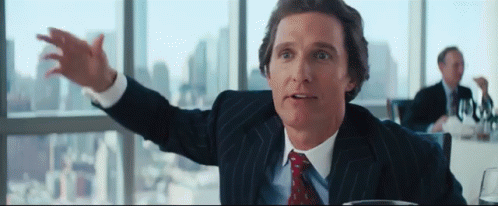
But this time, everything seems to indicate that the Analytics Engineer is here to stay.
Here is the deal:
Analytics has developed from a support to an advisory role, taking a driver seat in product innovations (setting and determining product KPIs, roadmaps, etc.). On the other hand, the data engineering role can, to a large extent, be automated.
Why does it matter?
Because whether you have a handful of data engineers or only a few data analysts with some coding skills on your team*,* when these worlds converge, you will need Analytics Engineers to act as the bridge between the producers and the consumers of your data.
In the following article, we’ll cover the rise of the Analytics Engineer, we’ll define the key responsibilities of Analytics Engineers and guide you in identifying your next Analytics Engineer. All of this to ultimately help you understand whether your organization needs one.
The rise of the Analytics Engineer: How did it all start?
To grasp why Analytics Engineers are here to stay, we must understand where it all started. Originally coined two years ago, by Michael Kaminsky, the term came from the ground-up, when data people experienced a shift in their job: they went from handling data engineer/scientist/analyst’s tasks to spending most of their time fixing, cleaning, and transforming data. And so, they (mainly members of the dbt community) created a terminology to describe this middle seat role: the Analytics Engineer. The real question becomes: How long ago and why did we experience this?
Three big changes in the data tooling stack are at the core of the development of the Analytics Engineer:
- The emergence of cloud-based data warehouses brought about a shift from the ETL (Extract, Transform, Load) to the ELT (Extract, Load, Transform) model. What does that mean? Not so long ago, if you wanted to get data into your warehouse, someone had to extract it by writing code to get the API, transform it, and finally load it into the data warehouse. But today, the reality is that modern cloud data warehouses (database services, hosted by public cloud, accessed remote) automate the Extract & Load steps so we can now do everything in SQL. This means ownership went from software engineers to land in the hands of less technical, business-savvy profiles handling simple SQL queries once it has arrived in the warehouse,
- Second, the growth of data pipeline services (eg. Fivetran or Stitch) means that data integration and extraction were almost automated. The work of a software engineer became less low-level intensive.
- Finally, the rise of user-friendly SQL-first BI tools (eg. Looker) enabled analysts to do more, focus on the creation of more complex pipelines all while saving time.
These shifts made access to data easier than ever. Every company now collects, tracks, and reports large amounts of data on everything but also on anything... This explosion of data comes with the cost of extracting the relevant data. To achieve this, companies must be sure to build the right data teams.
Today, the distinct jobs that existed in traditional data teams have converged: the line between the roles of data engineering, data science, and data analysis is blurry. But we cannot expect data scientists or analysts to be great working software engineers and vice-versa. Although this is exactly what we see happening…
Today, most data teams resemble something like this:
- Data analysts work with business and build reporting dashboards with Tableau, SQL tools, or modern BI tools. While data analysts should be focused on delivering results as quickly as possible, they tend to spend more time searching for the correct data and transforming it than delivering. Their time is also increasingly stretched by requests to build BI tools for each different area of the organization.
- Data engineers are the plumbers responsible for data modeling. They build customer data integrations, all while ensuring a smooth pipeline orchestration and maintenance of the data platform. They are great software engineers, but they are not trained to leverage data effectively nor to apply business science to engineering.
- Data scientists have an in-depth understanding of the “behind the scenes” of the algorithms, their quality, and optimization enabling them to model and infer statistics. But they spend a lot of their time cleaning and orchestrating the data to feed it into the machine learning models, which is far from being their priority.
The gaps mentioned above are exactly where Analytics Engineers walk in.
So… what is an Analytics Engineer?
An Analytics Engineer builds, transforms, tests and deploys models that support the queries of the rest of the data team. The objective of the job is to enable data products to talk to data consumers: the Analytics Engineer stands as the technical moderator of business and technical teams. It all comes down to this: Analytics Engineers allow their teams to navigate quickly the modern stack, all while leveraging the right data to answer their needs.
What responsibilities does an Analytics Engineer have?
At the intersection of business, data engineering and analytics, the Analytics Engineer travels back to SQL land to bring data models to life. In their day-to-day job Analytics Engineers will:
- Own and clean the datasets, building quality and performant data models to help other data team members work save time. Basically, they take control of data quality.
- Work closely with product managers, software engineers, and data teams to make sure analytics are seamlessly integrated with products across the organization.
- Validate and reshape datasets, transforming data so it is easily available for analysis. They also work on the design and development of CI/CD pipelines so that data teams can efficiently apply, test, and release analytics.
- Apply and communicate software engineering best practices in their code and to the analysts and data scientists.
- Create and maintain documentation around data to spread data literacy
- Act as facilitators between the business and technical teams: they can translate the strategic needs of the business into specific data models
Obviously, as the modern stack continues its development evolve, we are not done figuring out how to use all these new tools, who should use them, how we should report around them, etc. It only makes sense that the exact responsibilities of Analytics Engineers are still in the works. But the skillset required for such a role is straightforward.
All this is great, but so what skills should an Analytics Engineer have?
The next Analytics Engineer at your company may have a ton of software engineering skills or may very well be proficient in only one scripting language. What is important is that the Analytics Engineers bring these two worlds together. They won’t be the best software engineer out there, nor will they be the business go-to deal marker. And they really shouldn’t be: all the value they will add comes from their ability to blend technical and domain expertise in their daily tasks.
So how do you identify a good analytics engineer? Look at the skill sets.
Your Analytics Engineer should have:
- Experience working as part of a data team: they must be aware of the roles and responsibilities of data scientists, engineers, and analysts.
- SQL Wizard: Analytics Engineers should be fluent in SQL.
- Software Engineering Best practices: they will be responsible to use the best practices to ensure the whole team perform efficiently and has good quality standards.
- Great communication & interpersonal skills: they must be real team players to ensure a smooth communication between tech and business role. They will also guide, develop and mentor the data team around the stack, so demonstrating conciseness and clarity to express technical concepts is critical in this role.
- Written skills: they will be responsible for documenting learnings for the rest of the data team.
- Finally, the Analytics Engineer will most likely work on the tools presented in Figure XX. Therefore, working experience with cloud data warehouses is important and more generally speaking a solid understanding of data engineering tools and their ecosystems is undeniably a plus.

One interesting point was put forward: soft skills are almost more looked into than technical skills in this analyst role. Claire Carroll, a senior Analytics Engineer from dbt Fishtown Analytics developed that from her experience, the best Analytics Engineers she met were “people who do not come from the engineering background but instead from the operations/consulting/financial area”.
The skills needed to be a great Analytics Engineer spread across a wide spectrum requiring both technical expertise and solid business acumen. A certain level of experience calls for looking for a somewhat senior profile. At first sight, you may think it is impossible to find someone with such qualifications – but for an Analytics Engineer, the importance is to hire someone that will grow in their role, especially as Danielle Leong, Senior Analytics Engineer at Starry said, the role is more about “the mindset than the skillset”.
Do you really need an analytics engineer?
Since not long ago, companies experience that, thanks to data, the sky is the limit: their business impact is directly correlated to their ability to navigate the modern stack efficiently and make strategic use of the available data. This only stresses the importance of creating the right data team in place. The Analytics Engineer should be an integral part of that team because:
- First, remember that the Analytics Engineers saw birth naturally out of necessity. Data teams’ scope of work has only increased, and the amount of code they oversee is growing exponentially. So, whether you want it or not, the probability someone on your data team will unknowingly perform the tasks of an analytics engineer is high. Whether it will be your data engineer, scientist, or analyst, having them sit by idly performing tasks that are not theirs, is not be the best use of their time and will only decrease their satisfaction and will for personal development.
- Ultimately, the emergence of Analytics Engineers is about freeing data analysts and engineers to focus on strategic business decisions and to be more creative and specialized in building data processes. By ensuring the platform is scalable and well-maintained, they enable the rest of the team to save time and have only from a far, remote access to the dirty low-level coding work.
- On top of efficiency reasons, an analytics engineer is less expensive than a data engineer and can help shrink the size of your data team by an important factor. Not only will this save on your expenses, but it is also a lot easier to find people with SQL skills than with ML and big data engineering expertise on the market.
- At an organizational level, we see data science and analytics penetrating functional areas that were very low on tech just a while ago. Many of those areas couldn’t afford to have their decisions backed up by the expensive use of analytics. As data paves its way through the organizational structure, more and more companies undergo a structural reshuffling to place what used to be siloed data teams in business functions. Data teams are multiplying and moving in central parts of the organization, stressing the need for well-rounded (business and tech-savvy) profiles, such as Analytics Engineers.
Too often, we tend to see the world as binary: we think a career path is choosing between getting more specialized or going into management. But there are many different paths to seeing birth in the world of data, and Analytics Engineers are just one of the first. The importance of defining key data roles goes far beyond simply saying ‘oh I want someone to do something with my data' in an organization. Any stakeholder will want to understand the key roles his team players impersonate before building/joining a company. This relates directly to other topics we have already discussed as having the right data strategy, different tribes of data scientists and figuring out how to build a great data team.
Subscribe to the Castor Blog
About Us
We write about all the processes involved when leveraging data assets: from the modern data stack to data teams composition, to data governance. Our blog covers the technical and the less technical aspects of creating tangible value from data.
At Castor, we are building a data documentation tool for the Notion, Figma, Slack generation. We designed our catalog software to be easy to use, delightful and friendly.
Want to check it out? Give the CastorDoc software a test drive with our free 14-day demo.
You might also like
Get in Touch to Learn More
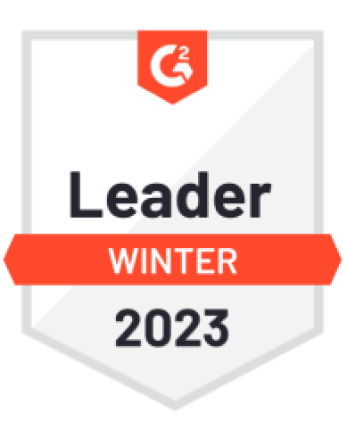
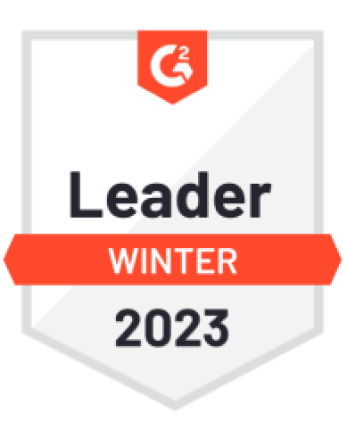

“[I like] The easy to use interface and the speed of finding the relevant assets that you're looking for in your database. I also really enjoy the score given to each table, [which] lets you prioritize the results of your queries by how often certain data is used.” - Michal P., Head of Data