How Generative AI is Changing the Way We Manage Data? - Get Ready for it
Maximizing the benefits of GenAI: have your technology, data & guidelines AI ready.
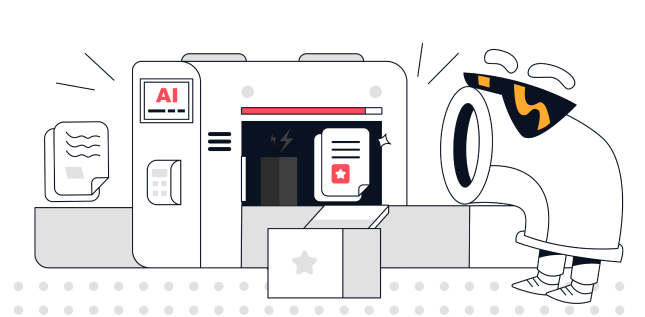
Introduction
Generative AI (GenAI) is changing how companies manage their data. It allows employees to access and analyze data easily. Workers can now find new insights in large data sets on their own. For example, Morgan Stanley uses GPT-4 to help financial advisors answer client questions accurately, building clients’ trust and bringing business value.
By 2026, 95% of workers will likely use AI routinely, including in data management roles. GenAI is forecasted to reduce manual data management costs by up to 20% annually.
However, businesses can be slow to adopt new technologies due to a fear of the unknown. A pattern observed in the past with the introduction of internet access and smartphones. When it comes to GenAI in data management, businesses worry about GenAI’s potential risks and high implementation costs.
Will businesses use AI to get more value from data management? Or will they fall behind competitors? This article explores the new capabilities generative AI brings to data management, the potential business value and risks involved. We further highlight how companies can prepare their technology, data, and guidelines to effectively and responsibly integrate generative AI into their data management practices.
I - What New Capabilities Does GenAI Bring to Data Management?
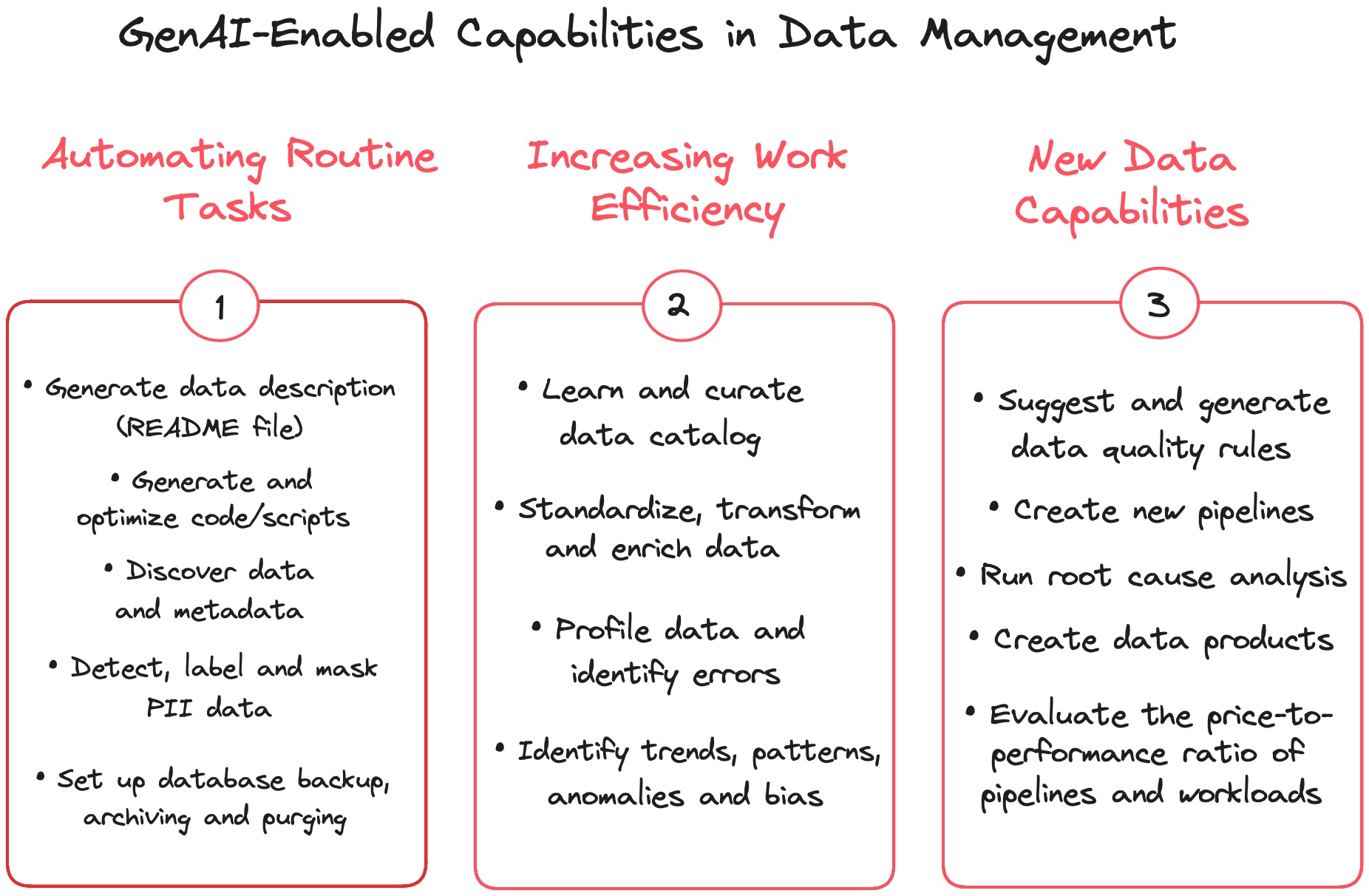
Generative AI provides companies with new ways for employees to work with data. These capabilities fall into three main categories:
1. Automating Routine Data Tasks:
People get overloaded with too much data and digital information daily. This backlog is called "digital debt." Data and governance teams dedicate substantial time to meticulously documenting data assets. However, this documentation process is tedious, time-consuming, and requires continuous updates to remain accurate. Automating these documentation tasks could significantly alleviate these teams' workload and improve efficiency. Generative AI can automate routine data management tasks such as describing data, finding sensitive info, setting up databases, and archiving. This allows companies to reduce employee overload and focus their efforts on more valuable work.
2. Enabling More Efficient Data Work:
In our recent article titled "The Self-Service Paradox: When Expanding Data Access Breeds Chaos" we discuss how company data exists across many scattered places - documents, processes, employee knowledge. This makes it hard to access. Generative AI acts as a bridge, letting employees rapidly self-serve and access the data and expertise they need. They don't have to constantly pester colleagues or data teams. With faster data access, employees can do tasks such as organizing data catalogs, standardizing inconsistent data, and finding errors more efficiently and accurately.
3. Unlocking New Data Capabilities:
CastorDoc's article on how AI shaking the world of data governance explores how generative AI introduces brand new data management features that go beyond what humans can do alone. Tech companies are now empowering businesses to analyze data in new ways their engineers couldn't before. New capabilities include suggesting data quality rules, automatically creating data pipelines, analyzing root causes, generating data products, and evaluating performance metrics. Best of all, anyone can use these AI capabilities conversationally without special programming skills.
While AI has been used in data management before, generative AI removes previous technical barriers with its conversational approach. The benefit is giving organizations an easier, more efficient way to fully utilize their data assets. However, these new capabilities also come with their share of potential risks.
II - What are the business value and potential risks?
Business Value of Generative AI
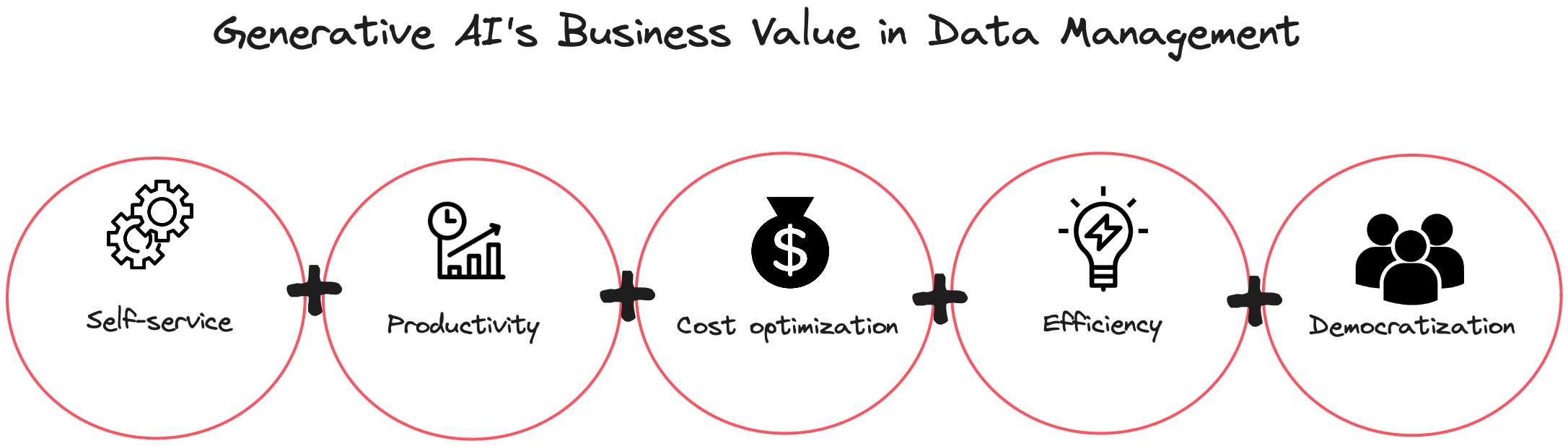
Companies are using Gen AI in all kinds of ways - from improving customer service to automating manual tasks. In data management, Gen AI unlocks value in 5 major ways:
- Self-Service Made Easy: Instead of complex commands, employees can ask questions in plain English or their language. Gen AI understands and enables them to operate autonomously, without any training.
- Productivity: By automating tedious boring, repetitive tasks, Gen AI frees workers up to focus on the important, strategic work.
- Cost-Cutting: GenAI can uncover insights from previously untapped dark data and optimizes costs associated with labor and time.
- Efficiency: In this blog post, CastorDoc highlights how with Gen AI, there's no need to wait ages for reports from data teams. Gen AI accelerates the time-to-insight, enabling workers to make prompt business decisions.
- Data Democratization: By 2025 natural language will be the main way we interact with data. Making data accessible to everyone, everywhere.
But There Are Some Risks...
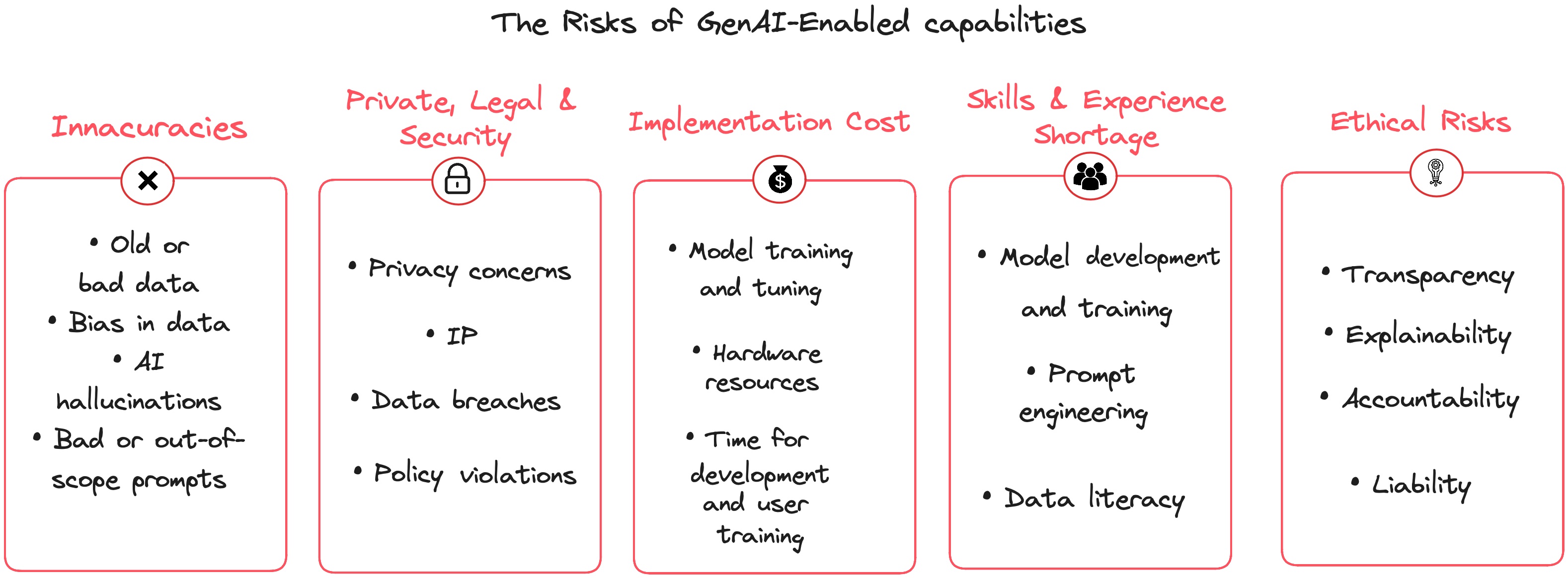
While Gen AI is a powerful tool to increase business value in data management, companies need to be mindful of a few potential risks:
- Accuracy Issues: Sometimes the results can be unreliable due to poor data quality or unclear instructions.
- Privacy & Security: Using proprietary data raises privacy concerns. Robust access controls are a must to maintain trust.
- Implementation Costs: Installing Gen AI isn't free - there are software, hardware, and training costs. But the long-term gains can justify the investment.
- Skills Shortage: There's a shortage of AI talent out there. Companies must upskill employees or recruit specialists to build sustainable capabilities.
- Ethical Pitfalls: Companies need to really understand how these AI models work to ensure transparency and ethical, accountable use in decision-making.
The potential is huge if you can navigate the risks wisely! Gen AI could revolutionize how businesses interact with and leverage our precious data assets.
III - How to Prepare for Generative AI in Data Management
1. Get Your Technology Ready
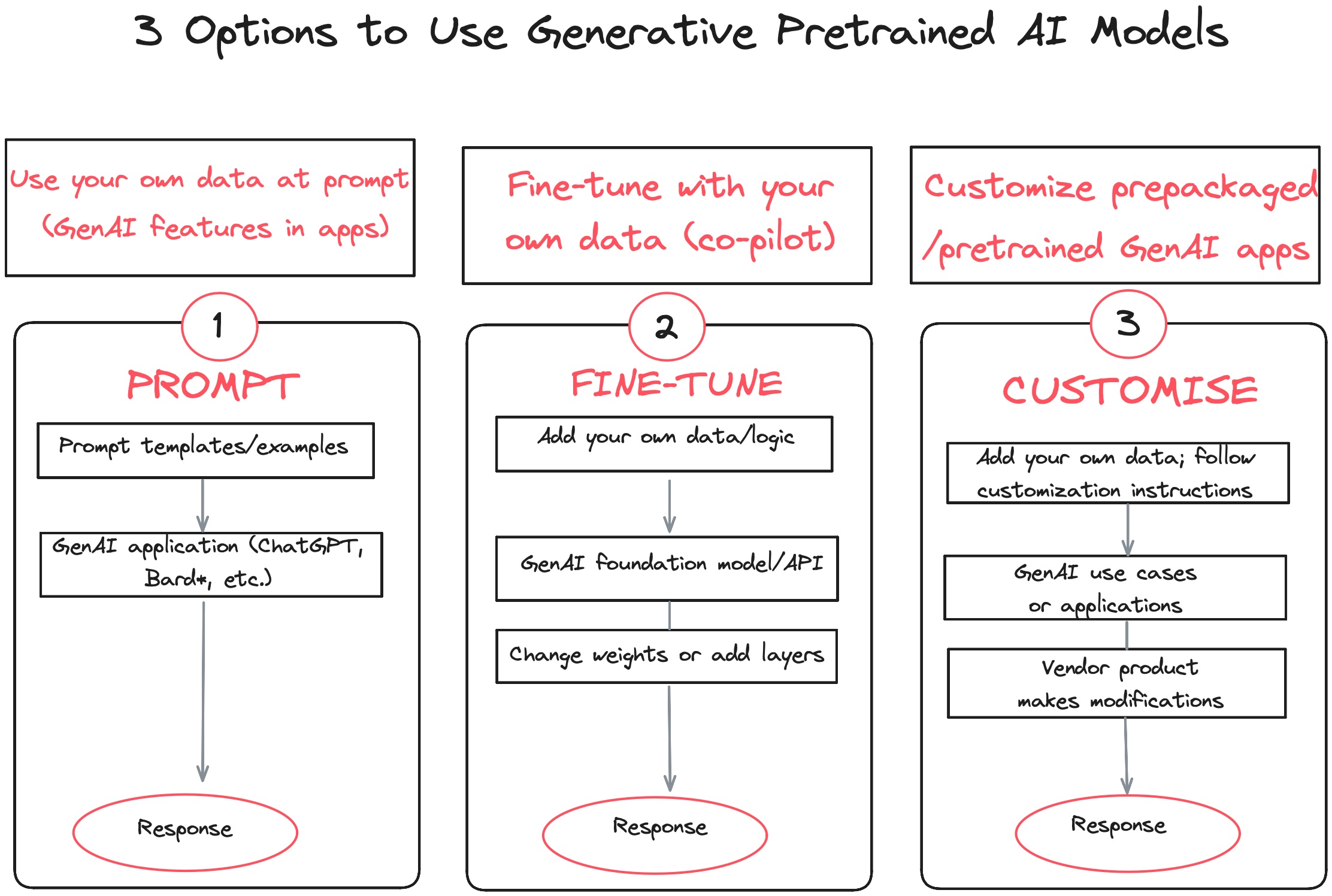
Companies have three main options to utilize generative AI models. They all require purchasing the necessary software and technologies:
Option 1: Prompt-Based Usage With Your Data (Like ChatGPT)
- You simply enter instructions or prompts, and the AI generates tailored responses for you.
- ✅ Pros: Very easy to start using with low costs and minimal skill requirements. Seamlessly integrates into existing workflows.
- ❌Cons: The AI model works like a "black box" - you can't see how it operates. There are limits on how much input you can provide. Potential risks of inaccurate outputs if using outdated data. Less control over security/privacy.
Option 2: Fine-Tune a Pre-Trained Model With Your Data
- Take an AI model that was initially trained on broad data, then further customize/fine-tune it using your company's specific data.
- ✅ Pros: Generates much more accurate results tailored to your business. Allows for longer input sizes. Better control over security with your private data. Lower risk of irrelevant outputs.
- ❌Cons: More expensive and time-consuming process to fine-tune the model. Requires skilled staff. May be overly specialized for your use case.
Option 3: Customize Pre-Packaged Generative AI Applications
- Tech vendors provide pre-built generative AI applications that you can further customize by adding your data and adjusting settings.
- ✅ Pros: Highly accurate results customized for your needs by incorporating your proprietary data during training. Maximum control.
- ❌Cons: Most expensive option. Longest implementation timeline due to customization work. Highest skill requirements for your team. Potential security concerns from customization process.
The three options represent trade-offs between ease of use, cost, customization, accuracy, security, and skills required. Organizations must evaluate their needs, data availability, budgets and internal capabilities when deciding which approach to take.
2. Get Your Data Ready for AI
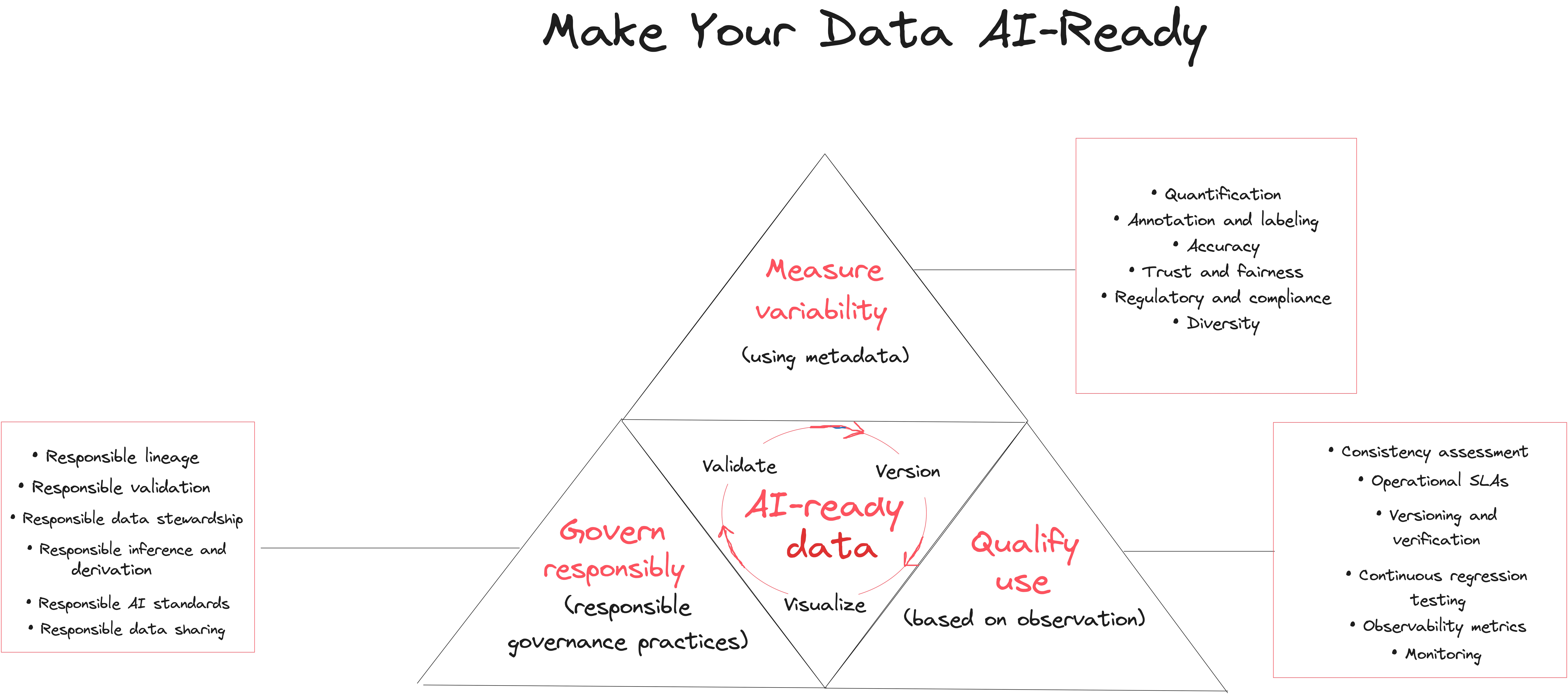
Getting your data ready to work with AI involves three main steps:
Step 1: Measure Data Variability
- Assess how well you understand your data using metadata
- Look at areas like data organization, accuracy, fairness, regulation compliance, diversity
- The more complete your metadata, the better positioned you'll be
Step 2: Qualify Your Data
- Evaluate if your data is suitable for specific AI use cases
- Perform consistency checks, set operational standards, data versioning
- Test continuously to ensure data quality and reliability over time
Step 3: Govern Data Responsibly
- Implement practices for ethical, compliant data usage
- Establish data lineage, validation, stewardship
- Follow responsible AI standards
- Enable data sharing while monitoring quality
Preparing data for AI is a continuous cycle - measure, qualify, govern. As data constantly changes, you need ongoing efforts. Using existing data management tools can help streamline this process. Some of these tools include metadata, lineage, quality, analytics, monitoring solutions. Ultimately, ongoing attention and adjustments are required for AI-ready data.
3. Prepare Robust AI Guidelines
Before integrating Generative AI, it's crucial to establish clear guidelines governing its usage. Robust AI guidelines act as guardrails, ensuring proper data handling and responsible AI practices. This mitigates risks, protects sensitive information, and promotes ethical AI usage.
Step 1: Update Data Policies
- Thoroughly review and update all existing data policies
- Address data sourcing, privacy protection, quality assessments
- Safeguard against risks like data breaches, inaccuracies
- Implement strict access controls and encryption protocols
- Assess reliability of data sources used by AI models
- Establish protocols for evaluating externally-sourced data
Step 2: Establish Comprehensive AI Usage Policies
- Develop holistic policies governing responsible AI usage
- Make data privacy and security top priorities
- Ensure legal compliance with data laws and regulations
- Define clear ethical guidelines for using AI outputs
- Establish user roles, responsibilities and accountability measures
- Maintain transparency through detailed documentation
- Implement channels for user feedback and complaints
Step 3: Implement Feedback Loops
- Solicit continuous feedback from AI users and engineers
- Quickly identify and resolve any issues or concerns
- Use this iterative feedback to consistently refine AI guidelines
- Enable ongoing improvement of AI implementation effectiveness
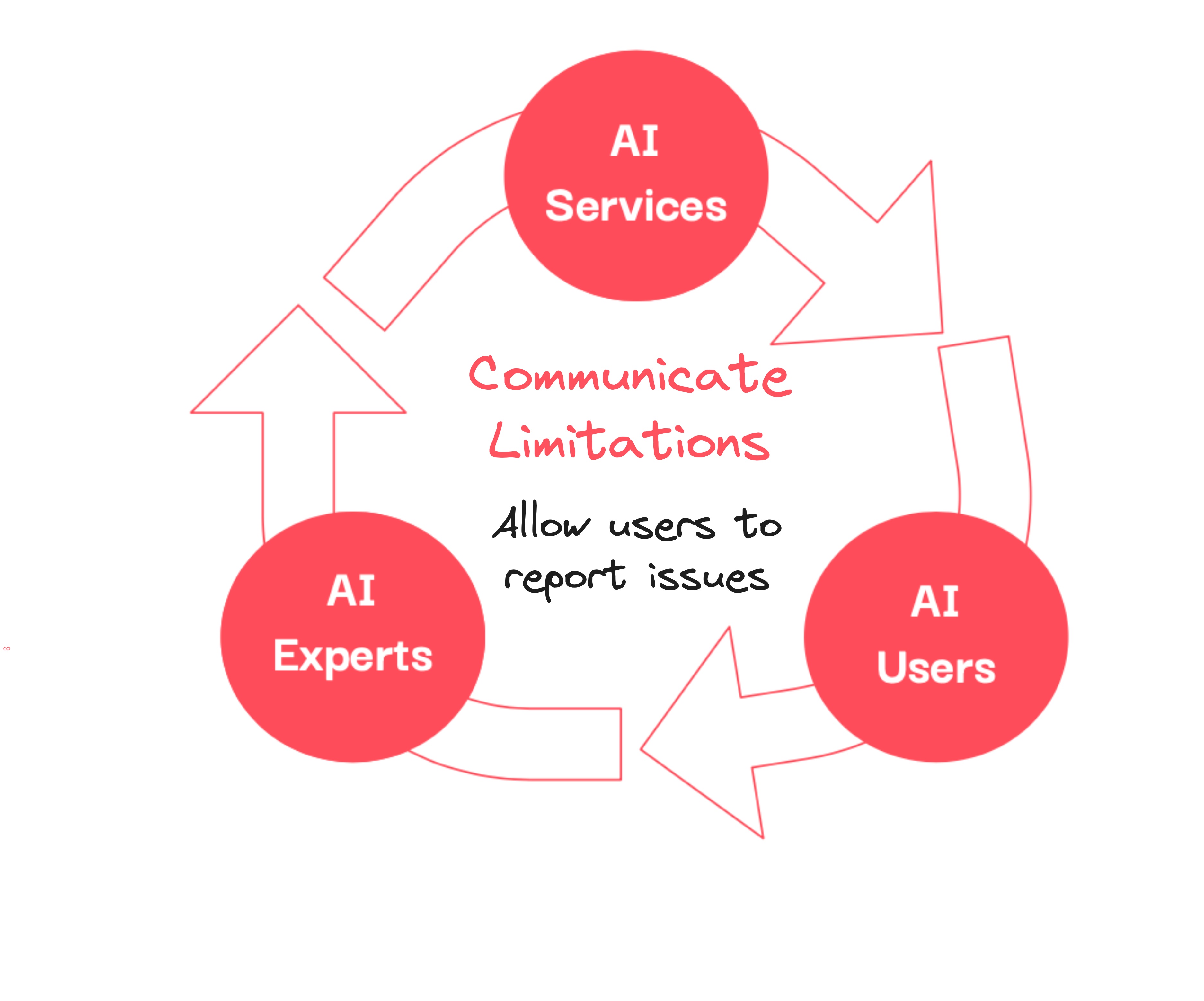
Step 4: Invest in Data & AI Literacy

- Provide comprehensive training on AI principles and data management
- Build upon existing data literacy as the foundation
- Offer workshops, seminars, educational resources
- Encourage cross-departmental collaboration on literacy efforts
- Empower employees with AI knowledge to maximize its value
Robust AI guidelines, combined with continuous feedback loops and organization-wide literacy programs, enable valuable AI adoption while responsibly minimizing risks long-term.
Conclusion
As GenAI becomes more common in workplaces, it can make tasks easier, save time, and bring new opportunities for businesses. But using it also comes with risks.
To get ready for using GenAI, businesses need to make sure they have the right technology and data ready. They also need to help their employees understand how GenAI works and what it means for their business.
Overall, using GenAI successfully means getting ready, staying flexible, and being responsible. By doing this, businesses can extract the most value out of GenAI in Data Management.
About Us
Ready to elevate your data preparation and harness the full power of AI in your business? Try CastorDoc today and experience a seamless integration of advanced governance, cataloging, and lineage capabilities with the convenience of a user-friendly AI co-pilot. Whether you're a data professional seeking control and visibility or a business user desiring accessible and understandable data, CastorDoc is your partner in unlocking the transformative outcomes of AI. Don't wait to transform your data governance—start your journey with CastorDoc today.
You might also like
Get in Touch to Learn More
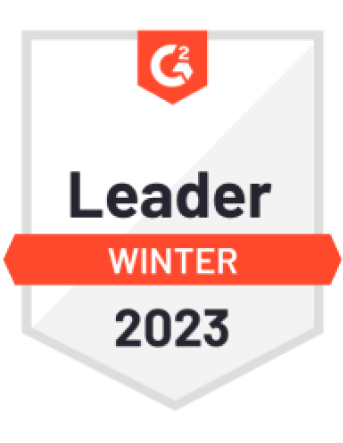
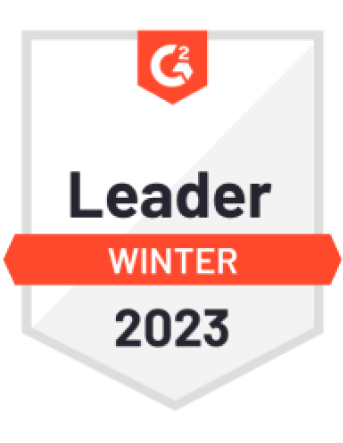
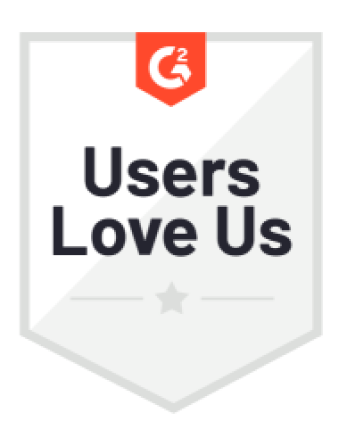
“[I like] The easy to use interface and the speed of finding the relevant assets that you're looking for in your database. I also really enjoy the score given to each table, [which] lets you prioritize the results of your queries by how often certain data is used.” - Michal P., Head of Data