Data Standardization, Explained
Data quality difficulties can be eliminated by uniform data standards. Our data standardization article explains everything you need to know.
.png)
An important aspect of effective workflows that contribute to the success of businesses is the maintenance of organized data in different systems. Data standardization across teams or across a whole firm can be a significant undertaking. When there are uniform data standards in place, everyone may use the same information without having to relearn a new format, and problems with data quality can be avoided altogether.
In order for businesses to obtain faster and more accurate insights, data standardization offloads all the intricate nuances of how data is acquired, standardized, and patched together. In this post, we'll discuss the significance of data standardization, the various approaches to standardizing data, and the consequences for businesses that don't standardize their data.
What does data standardization mean?
The goal of data standardization is to ensure uniformity in data structure and content across all data types. Data warehouses, lakes, cloud storage, and databases are just some of the common places where companies keep their data. However, problems might arise when data collected from several sources aren't standardized — such as when you combine, compare, and/or transform that data in producing dashboards and visualizations.
There are numerous reasons why it is essential to standardize data. For starters, it aids in the creation of a well-defined set of elements that serve as a complete catalog of your data. Whatever it is you're looking for, from insights to problems to address, a solid grasp of your data is an absolute must.
To get there, we need to standardize this information. Standardization includes sorting and transforming your raw, disparate data into a unified format so it can be usable no matter the source it came from.
In addition, it means using clear and consistent documentation. The metadata you create provides context for team members to reference as they use the data in analyses.
And there you have it: the foundation of your data standardization procedure. Standardization will enable more accurate analyses and internal consistency, which will drive more business impact.
Why is data standardization important?
The necessity of consistent data standards is evident in every corner of the world. As businesses acquire and keep ever more data, the importance of data governance has risen significantly in recent years.
Organizational data availability, usability, consistency, data integrity, and data security are all aspects of data governance. Data governance encompasses the processes, responsibilities, policies, standards, and measures required to manage data throughout its lifecycle and for the use of businesses. Users are given the ability to derive valuable business insights from data that is both secure and reliable, thanks to good data governance.
When an organization implements a data governance framework, data standardization is often key to data quality. It enables coworkers from different teams and departments to work together using data from all different sources while maintaining accuracy. Data processes and cooperation are simplified when data can be used across contexts, sources, and users to drive towards common insights.
Data standardization vs. data normalization
Two typical approaches to dealing with disparate data are data normalization and data standardization. While both strategies are used to consolidate data, they do so in distinct ways.
Data standardization, as previously discussed, is the process of ensuring that your data set is comparable to other data sets. It's crucial to any data-related endeavor, whether academic or commercial, and everyone who utilizes data ought to give it some thought before beginning to gather, clean, or analyze it.
On the other hand, data normalization employs a rigorous set of standards to create uniform, ordered data within the context of a database. When data is normalized, outliers that skew analysis are removed. Once the data has been cleaned up, it may be organized, analyzed, and interpreted with ease. In the absence of normalization, useful information is foregone.
Commonly used data standardization methods
Data standardization ensures uniformity and tidiness within a business’ data lake. Organizations can make better use of data and maximize storage efficiency thanks to data standardization and subsequent implementation of data standards.
There are a couple of data standardization methods that companies may use:
1. Data management platform
Standardization is the process by which raw, inconsistent data is sorted and transformed into a consistent, predictable, and unified format using a data management platform (DMP). Simply said, a DMP is a cloud-based data warehouse. When a company begins using a DMP, the platform starts gathering, organizing, and archiving all of the associated data. The information is then transformed into a more digestible format, complete with charts, graphs, and statistical highlights. As a result, businesses can take action based on the insights gained.
Collaborative research, large-scale analytics, and sharing of sophisticated tools and methodology are all made possible by this method. Analyzing consumer behavior, seeing trends, targeting, nurturing, and converting them all become simpler, faster, and cheaper with more consistent data. With a DMP, companies don’t have to worry about manually inputting data — unlike with using spreadsheets.
2. Spreadsheets
Perhaps one of the most basic ways to standardize company data is by using Microsoft Excel — or Google Sheets if you prefer. The main issue with using a digital spreadsheet is that you need to manually input your data into your spreadsheet before the program can even begin the process. However, once that’s done, all you have to do is type in the right formula, and the digital spreadsheet will do the rest.
Instead of showing how far each data point is from the mean on its own scale, z-scores report the number of standard deviations each point is from the mean. This adjustment is made during the data processing.
Benefits of standardizing data
Having data that isn't standardized would be a major headache that would cause unnecessary back-and-forths between departments, which equates to extra work for humans to convert. Data is more of a burden than an asset if nobody in the company understands it or can make sense of it.
Data standardization is an essential initial step if you are in the middle of a data migration or modernization project or if you have been tasked with cleaning up your customer database before launching a loyalty program or marketing push. A company's data can either serve as a catalyst for making meaningful connections with new customers and scaling to new heights, or it can be a burden that slows down growth and prevents them from moving forward.
With that said, here are some of the biggest benefits of data standardization:
1. It improves data accessibility
In order to maximize its usefulness, data should be made available to as many people as it needs to inside an organization. Businesses gain efficiency in decision-making and action-planning when information can be freely distributed among workers and between departments.
When data is standardized, it can be used without further scrutiny or reorganization to ensure that all parties involved are always on the same page.
2. It helps make more informed decisions
It's far simpler to read, organize, and analyze data that has been standardized. Data that is simple to understand will help you spot patterns and learn more about your customers, helping you make better choices. The ability to strategically direct the business’ investments and expenditures toward its desired outcomes is only possible when you have access to reliable data.
3. It saves resources
Standardized data ultimately improves productivity and potential. Relying on speculation rather than evidence might lead to undesirable outcomes, especially when it comes to large pools of data. The time that would be better spent making decisions and taking action is lost on manual data verification and correction. When a business’ data is standardized, its teams can rest easy knowing that the data will always be presented in the same way, no matter which technology was used to acquire it.
The Future of Data Standardization
Every day, new data is created at almost unfathomable rates and in a staggering variety of formats. Before your data lakes become data swamps, you need to gain control of your company’s data or risk drowning in a sea of incomprehensible data.
In order to draw meaningful inferences from data, companies need to compare it to other data sets, which is why standardized data is so important. Decision-making, system efficiency, and customer connection-building all benefit greatly from accessible and accurate data. When data is standardized, businesses can maximize their potential and benefit from increased gains.
Subscribe to the Castor blog
You might also like
Get in Touch to Learn More
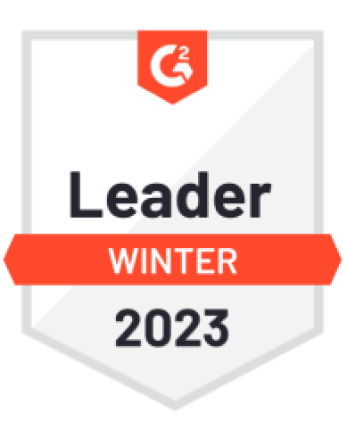
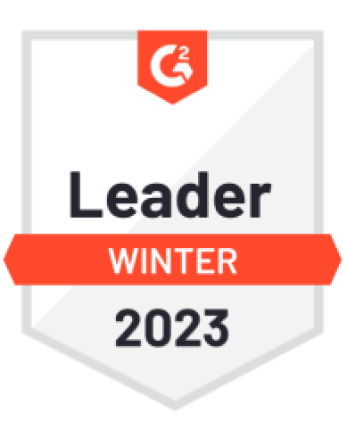

“[I like] The easy to use interface and the speed of finding the relevant assets that you're looking for in your database. I also really enjoy the score given to each table, [which] lets you prioritize the results of your queries by how often certain data is used.” - Michal P., Head of Data