Data Catalog ROI - A Primer
A framework for computing data governance ROI

Introduction
Have you ever thought about the consequences of brushing your teeth for the first time on your 50th birthday? Doesn't seem like a good idea. Why? By the age of 30, your teeth would probably be in a serious state of decay already. Your dentist would tell you to replace them, and it would cost a fortune. Now, why would anyone do this when you can just put in the small effort of brushing your teeth every day and never have to face this kind of horror?
Funny enough, it goes the same way with data documentation. The more you wait, the more you lose. In response to the heightened complexity of the data infrastructure, people are turning to data catalogs to help make sense of the mess. The issue is, companies often end up leaving a lot of money on the table by implementing a catalog too late. Each year you don’t invest in data documentation contributes to building up your technical debt. When you finally decide to start, you’ve got the equivalent of the angry dentist telling you setting things right will cost a fortune.
How to avoid starting off with a huge technical debt? Implement a rigorous data documentation practice early on. This article highlights the exact breakdown of the consequences of waiting too long before implementing a data catalog.
The table below describes the savings made by a data team of 10 employees with a data discovery tool. For the rest of the article, we have calculated the yearly savings for a 10 employees data team. If your team is bigger/smaller, you can simply change the numbers in the excel ROI calculator to get the right numbers for you.
.png)
This article is divided into two parts:
1 - How much can a data catalog save your team every year?
2 - The cost of waiting too long to implement data discovery in your organization
Get the Data Catalog ROI calculator
I - How much can data discovery save your data team each year?
The first objective of this piece is to put a number on how much organizations save each year by investing in a data discovery solution. We’ll bring the focus on three areas of value generation that data catalogs particularly impact: Onboarding, Discovery and Infrastructure Costs. By looking at these, it makes it easier to calculate the time your employees save at each step of their workflows when there is a data catalog involved.
Assumptions/Hypothesis
This article gets into numbers: we have tried to avoid approximate ROI calculations. Here are the main assumptions we used to reach our conclusions. You can change the assumptions and obtain the numbers for your company here.
.png)
Three use cases: Onboarding, Discovery and Infrastructure
1 - Onboarding
What is it?
Data teams don’t escape the cycle of life. Every year, new people arrive and others leave. Inevitably, new hires go through an “onboarding phase” before they can be fully operational.
We deem a data person to be “operational” when she has a clear idea of the data projects and their evolution, the data owned by the company and where she can find it, etc.. It’s no secret that time = money, and the more time data people spend on the onboarding phase, the more money you spend. Data catalogs can help you reduce the onboarding of new joiners. Let’s see how.
Without a catalog
Here’s a small thought experiment about what an onboarding process looks like without a data catalog. It’s the first day of the new data analyst on your team. He’s immediately assigned a mentor who can get him running: take him through the current roadmap, the key peoples to contact, the tools used, key metrics to track, an overview of past achievements, etc. His mentor also explains how data is organized within the company and where relevant data can be found.
Usually, former employees left the company without documenting the knowledge that lived inside their heads (don’t be this person). The mentor has to go back and forth between the mentee and the former employee, trying to gather pieces of information, tie them together and get the ball rolling for the new joiner. This adds up a lot of time, making the onboarding process a tedious one. In total, this lengthy process takes about 4 weeks (if you’re lucky).
Based on our estimates, a data professional earns a daily salary of $600. Therefore, a four-week onboarding process costs approximately $12,000. Considering a 15% churn rate and a 15% growth rate for the data team, we estimate that there will be roughly three new onboardings per year for a 10-people data team. Annual onboarding costs without a catalog thus total $36,000.
With a catalog
Things look different once you have invested in a data management solution. The data catalog is a rich, constantly evolving knowledge base that contains all the information data people have inside their heads. The on-boarded person is thus fully independent and doesn't need a mentor to walk her through the company’s data assets. From column names definitions to metrics calculations, to project progress, the new joiner can learn everything simply by scrolling through the catalog interface.
The onboarding process takes approximately two full days, resulting in a cost of $1,200. Based on our assumption of three new onboardings per year for a ten-person data team, the annual onboarding costs with a catalog total $3,600. The table below illustrates the onboarding costs for a ten-person data team.
Savings on a yearly basis
You can change the assumptions and obtain the numbers for your company here.
.png)
2 - Data Discovery
Even more dreadful is the time and money you waste on daily, recurrent tasks such as data discovery. Employees are onboarded one time, but they engage in data discovery on a regular basis as a part of their job. Wasting time on data discovery matters thus has more important consequences.
What is it?
Data discovery is the process of finding and understanding the data you’re going to use for a data analysis project.
Without a catalog
Let’s look at how self-service analysis works without a data catalog. Let’s say you want to perform sentiment analysis on your product’s reviews so you can improve the product based on these feedbacks.
You err in the data warehouse, in quest for the needed data. Bad news: there are 20 datasets with the same name, and you have no clue which one is relevant. You end up finding some “close enough” data and using spreadsheet data from a past, outdated project. you have a few questions about missing values or column names in the dataset, but you have no idea who’s the owner of the dataset. Too bad, you’ll have to make approximations. You engage in data preparation steps, determine the needs for additional data, and repeat the same process to find the required data.
All this groping ends up taking a full day for a good data analyst. And a day cost $600, based on our assumptions. How does this change with a catalog?
With a catalog
Self-service analytics is taken to a whole new level when you have a data catalog. Instead of pinging colleagues from other teams to get semi-useful data, you only have to interact with the catalog interface. A good data catalog has powerful search capabilities, and guides employees towards the most popular & most used data assets in the company. In a few clicks, you can search the catalog and find popular data, trusted across the company. The whole process takes about one hour. That’s $75.
Again, numbers add up as soon as your data team grows. What do these numbers mean for a 10-people data team?
Savings on a yearly basis
You can change the assumptions and obtain the numbers for your company here.
.png)
Here, the yearly discovery time is calculated assuming that each data person gets involved in 20 data project per year. A “data project” refers to any analysis or reporting task that required data manipulation.
In the first case, you can start making sense of the data after a full day of work. In the latter case, an hour suffices to find, analyse & visualise the data for decision making. You save 600$-$75= $525 per employee per data project when using and maintaining a data catalog. Assuming (conservatively) that each employee gets in involved in 20 data projects each year yields, we conclude investing in data documentation saves $525*20 = $10,500 per employee per year. if you have 10 people on your data team, this brings the costs to $105,000.
3 - Infrastructure costs
What is it?
If you’re not frightened by the numbers above already, let us continue for a bit. A lack of well-documented data repository (aka. a data catalog) leads to a lot of redundant work. In fact, data people have no way of quickly knowing whether someone has already worked on a given task. As a consequence, data people often work on things that others have already done. This creates a lot of duplicates, ultimately leading to increased infrastructure costs.
Without a catalog
Now what happens when you don’t have a catalog? You end up creating assets that already exists because other people have already worked on a close-enough project. Sadly, it’s not free to store duplicates, and you usually end up paying a lot of storage costs. Without documentation, we estimate there are roughly 15% of duplicates and unused data. The issue is, 15% of your totally BigQuery bill is usually a lot.
With a catalog
An intelligent data catalog shall give you peace of mind on the matter. Duplicates are automatically detected, and you just need to press a button to delete them. Similarly, datasets that are dirty, unpopular and unused are automatically flagged, allowing you to dispose of the garbage rotting in your data warehouse or change its storage level. Finally, a catalog also allows you to spot the unoptimised and useless queries eating up a lot of storage, so that you can create new tables and point tables to them to eliminate the queries. Duplicates, stale data and unoptimized queries each represent roughly 5% of your data warehouse storage. Together, they make up 15% of your data warehouse, and thus of your storage bill. Cutting these through the use of a catalog cuts off 15% of your yearly storage bill.
Savings on a yearly basis
In this situation, the savings vary considerably according to the size of your organization. We’ll calculate the savings for a company with 500 employees. If you have a different employee count or number of tables, we recommend filling your details in this excel to get the accurate savings provided by a catalog.
.png)
With 500 employees, we assume you have roughly 5000 tables in your data warehouse. The average size of one table is 90GB, and the data warehouse charges you a yearly $0,24 per GB storage. Your yearly BigQuery bill thus usually reaches $108,000. If you have 15% of duplicates, stale data and useless queries due to lack of documentation, it costs you 0,15*$108,000 = $16,200 per year to store them.
With a data catalog, the duplicates are automatically removed. These 0% of duplicates cost you $0 per year. We’ve summarised these calculations in the table above.
II - The more you wait, the more it costs
A data catalog saves about $30,000/year/employee. This is why it already makes sense for a lot of organizations to pay hundreds of thousands for enterprise catalogs. Data catalogs would not have clients if this wasn’t the case.
The issue is, a lot of companies wait too long until implementing a data catalog and end up having to pay the cost of huge technical debt they’ve built up. This part is dedicated to evaluating the cost of this technical debt.
.png)
1. The cost of technical debt
Company that grow large cannot avoid investing in a data discovery solution. Tech giants, with hundreds of thousands of data assets, built internally their own tool to solve this problem. Airbnb built DataPortal, Uber DataBook, LinkedIn DataHub, Spotify Lexicon, WeWork Marquez, Shopify Artifact etc. Not one of these companies could do without a data discovery tool. Yet, the more you wait before implementing a tool, the more the initial investment in the tool will cost you.
The initial investment is the one-off documentation effort you make to get your data catalog running. Beyond the cost of software, a data catalog requires investment to train people & populate the catalog with metadata.
To illustrate the cost of technical debt, let’s do a simulation of what happens when you implement a data discovery tool at different growth stages your company. You can change the assumptions and obtain the numbers for your company here.
.png)
Now, don’t misread this. Even if the initial investment costs you $3 millions, it will be worth it and bring clear ROI. However, you would much rather pay $10 000 and benefit from the same ROI on the long term. Our advice: invest in data discovery EARLY.
Invest at T = 30 employees
Let’s say you implement a data catalog very early on, with only 30 employees in your organization. With 30 employees, you have two people on your data team and roughly 500 tables. The initial, one-off documentation effort is 15 min per table. This is fairly short because the two data analysts are extremely familiar with the data, and only need to put the knowledge living inside their head on paper.
In this case, it takes 1 hour to document 4 tables. It thus takes 125 hours to document all the data within you warehouse. From of salary assumptions in part 1), 125 hours cost you $9,375.
Invest at T = 500 employees
Now, let’s say you wait until you have 500 employees and a 25 people data team to implement a data catalog. At this stage, you have roughly 5000 tables to document. It now takes 45 minutes to document each table. Why? Early employees have left the company, making it difficult for you to gather the knowledge necessary for documentation.
Now, it takes you 3,750 hours to document all the data in your warehouse. This comes at a cost of $281,250.
Invest at T= 3000 employees
What happens when you wait until you have 3000 employees and 30,000 tables to document your data? Disclaimer: it doesn't look good.
At this stage, it takes 1.5 hours to document each table. It takes time to contact the tables owners who have left the company 5 years ago, there is no history of where anything comes from, the system is old, etc.. Documentation is now the biggest pain.
Your analytics team counts roughly 150 people. It now takes the team 45,000 hours to document all the data in the warehouse. This comes at a cost of $3,375,000.
Disclaimer 2: these numbers are not set in stone and will vary a lot according to your industry. The important stuff to realise is that the cost of waiting to implement a data catalog grows exponentially. Hence, the more you wait, the more you loose.
2. Hidden costs - missed opportunities
Waiting too long before using a metadata management tool also comes with a lot of hidden costs.
- Hiring & Turnover - No one likes to work with poorly documented data. People on your analytics team will find their workflows extremely frustrating. This will make it much harder for you to retain existing talents and to hire new ones. Just like some companies have a reputation for bad culture, yours will be reputed for its bad data. It’s hard to put a number on this kind of cost, but without discovery, data people spend at least 40% of their time rebuilding processes from scratch because SQL logic is unknown, or has been lost.
- No business adoption - Smart people working in your company are reluctant to use data because they don’t want to go through the hurdle of figuring out your swamp. Instead of taking the route of self-service and data democratization, you’re heading towards a place where people don’t want to use your data. Again, it’s hard to evaluate these costs, yet they should not be neglected.
Conclusion
Data catalogs have become vital components of any healthy data stack. Yet, these tools are not like other data infrastructure tools, in the sense that they don’t seem vital from the start. A lot of organizations know that they can’t get far in their business if they don’t invest in a strong storage and pipelining solution from the beginning. Just like people know that they have to buy shoes if they want to walk a long distance.
Documentation is never a priority, because you can survive without it for a long time. Just like people know they can survive for a while walking with a pebble in their shoe. Yet, lack of documentation becomes so painful over time that people ultimately have to invest in one. But when they do, they usually have accumulated such a high technical debt that the implementation costs are enormous. It’s just like taking the pebble out of your shoe after walking 100 miles. Your wound has become so big that you have to stop at the hospital for three days and rest before you can take up walking again.
The lesson is, don’t wait. You will have to implement a powerful documentation tool anyway. Make this investment early enough, to save you the pain of dealing with a huge technical debt and a monstrous bill.
Subscribe to the Castor Blog
About us
We write about all the processes involved when leveraging data assets: from the modern data stack to data teams composition, to data governance. Our blog covers the technical and the less technical aspects of creating tangible value from data.
At Castor, we are building a data documentation tool for the Notion, Figma, Slack generation. We designed our catalog software to be easy to use, delightful and friendly.
Want to check it out? Get a free demo.
You might also like
Get in Touch to Learn More
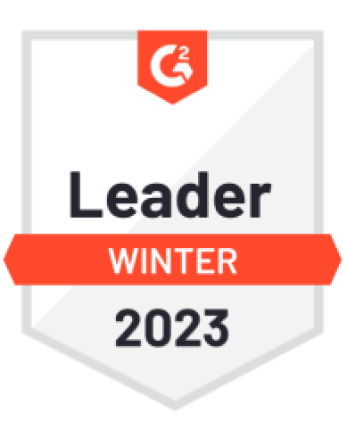
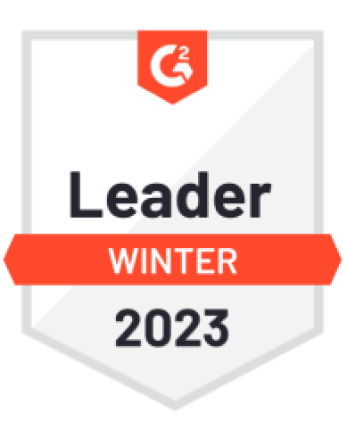

“[I like] The easy to use interface and the speed of finding the relevant assets that you're looking for in your database. I also really enjoy the score given to each table, [which] lets you prioritize the results of your queries by how often certain data is used.” - Michal P., Head of Data