How to Build Your Data Team
Overview of mid-market data team organization models

Peer reviewed by Kat Holmes – Data Director ITV
As businesses recognize the decisive power of data to achieve business goals, most are hoping to put data in the driver's seat of their business and product strategies. This entails putting together a strong data team which can effectively propagate its insights across different areas of the business. Unfortunately, this is no easy task.
To be truly data driven, companies need to build three capabilities: data strategy, data governance and data analytics.
.png)
Strategy: Data strategy is your organization's roadmap for using data to achieve its goals. It requires a clear understanding of the data needs inherent to the business strategy. Why are you collecting data? Are you trying to make money, save money, manage risk, deliver exceptional customer experience, all the above?
Governance: Data governance is a collection of processes, roles, policies, standards, and metrics that ensure the efficient use of information in enabling your organization to achieve its goals. A well-crafted data governance strategy ensures that data in your company is trusted, accurate and available.
Analytics: The term data analytics refers to the process of analyzing raw data to draw conclusions about the information they contain. Typically, those involved with data analytics in an organization are data engineers, data analysts and data scientists.
Ultimately, your ability to leverage data will depend on these three pillars. If you’re reading this and realizing that your organization possesses none of these, don’t worry. That’s why we’re here. A good place to start is to build a strong analytics team, one that is closely tied with the strategic goals of your business. It is the first pillar of your data organization, and the focus of the article.
When building a data analytics team, heads of data typically grapple with the following questions:
- How big should this team be?
- How many data engineers, data analysts, data scientists?
- How does the team interact with the rest of the organization?
- Which structure for the data team? Centralized or embedded?
They rightly do so; having a strong data team is not a luxury anymore, but essential to the very survival of a company today.
Let's start with the basics though.
Where are you in your data journey?
Before building a data team, it's important that you realize where you are in your "data journey", because this will directly affect the structure of your team. This part is thus dedicated to a simplified data maturity assessment. Beware, company size and data maturity are two different things. Your organization can be large but immature on a data level.
Data maturity is the journey towards seeing tangible value from your data assets. We propose a simple framework of data maturity assessment, in which you measure your ability to understand your past, know your present and predict your future. What do I mean by this?
Well, in most companies each department has its own set of KPIs that support the execution of the corporate strategy. It’s not enough just to define them, they must also be clearly tracked, and you must also have the ability to predict future outcomes against these KPIs. This ability rests on a clear knowledge of your present, which, in turn, builds on a strong understanding of the past. Do this, and you have found a simple way to assess your data maturity. For example, if you're unable to identify the revenue drivers for your company (your past), it means you need to work on your data maturity by bringing visibility to your business before you seek to predict future outcomes. We don't recommend skipping steps. It's like Maslow's hierarchy of needs, but for data.
.png)
Let's look at a couple of practical examples:
Marketing ROI. Define your ROI, across multiple channels, by using an identified attribution model. Then understand its evolution in the previous 12 months, and especially its drivers (identify performing channels, time of the year, product, ....). Then track on a daily/weekly/monthly basis its evolution thanks to a reporting tool you trust (present). Forecast your marketing budget based on these predictive models (future).
Customer Satisfaction. Define your customer satisfaction measure. Is it NPS, CSAT? Everyone in your company should share a common understanding of how it is computed. As with our previous example, compute its evolution in the previous 12 months, find its drivers (past). Then track daily the satisfaction of your customers with trusted dashboards. Identify action to take from today to increase it. Your understanding of the past and the present state of customers satisfaction will allow to predict churn efficiently (future)
Understanding your past and present is commonly referred to as performing descriptive analytics. Descriptive analytics helps an organization understand its performance by providing context to help key stakeholders interpret information. This context is usually in the form of data visualization, including graphs, dashboards, reports and charts. When you are analysing data to forecast the future, you’re engaging in predictive analytics. The idea with predictive analytics is to take historical data, feed it into a machine learning model that considers key patterns. Apply this model to current data, and hope that it will forecast the future. We’ll use the terms of descriptive and predictive analytics throughout the article to refer to understanding the past, present or predicting the future.
If you realize that your organization is not fully mature (ie. you don't have a clear understanding of your past and present), here are our recommendations for what should be the next steps of your data team.
Subscribe to the Castor Blog
Key players on a data analytics team
A data analytics team is usually composed of four core functions, which are detailed below.
- Data engineer: They are responsible for designing, building, and maintaining datasets that can be leveraged in data projects. As such, data engineers closely work with both data scientists and data analysts. We also include the new role of analytics engineer here, although, in practice, this role lies between analytics and engineering.
- Data scientist: They use advanced mathematics and statistics, and programming tools to build predictive models. The roles of data scientists and data analysts are pretty similar, but data scientists focuse more on predictive analytics than descriptive analytics.
- Data analyst: They use data to perform reporting and direct analysis. Whereas data scientists and engineers typically interact with data in its raw or unrefined states, analysts work with data that’s already been cleaned and transformed into more user-friendly formats.
- Business analyst/ops analyst: They help the organization improve its processes and systems. They focus on dashboarding, answer business questions and propose their interpretation. They are agile and straddle the line between IT and the business to help bridge the gap and improve efficiency. They frequently work with a specific business area such as marketing or finance, and their SQL literacy can range from basic dashboarding to advanced analysis.
- Head of data analytics: They provide strategic oversight to the data team. Their goal is to create an environment that allows all different parties to access the data they need painlessly, build the skills of the business to draw meaningful insights from the data, and ensure data governance. They also act as a bridge between the data team and the main business unit, acting both as a visionary and a technical lead.
How large should the team be?
Different companies will build data teams of different sizes, no one size fits all. We have studied the data team's structure of 300+ companies, with a 300-1000 employee range and derived the following insights:
- As a general rule, you should aim to have a total of 5-10% of data analysis savvy employees in your company. Some companies such as Amazon or Facebook are training a huge portion of their employees, but we have excluded them for our analysis.
- The first hires of a brand-new data team are often a data engineer and a data analyst. With just these two roles, organizations can already engage in some basic descriptive analytics. When building a larger team, think in terms of the skillset you need. A typical data project requires the following skills: database, software development, machine learning, visualization, collaboration, and communication skills. It is very rare to find individuals who possess all these skills. You should thus be aware of which skill each candidate brings to the table. Regardless of how many people you decide to hire, your team should ideally cover this skill set. Where you are in your data journey also impacts who you hire and at which stage. Generally, data analysts focus on understanding the past. That is, they take the data you have and try to understand the drivers of growth and other metrics. Business analysts/obs analysts are oriented towards the present (dashboarding). Finally, data scientists focus on predicting future outcomes. So, if you have trouble understanding your past, hire a data analyst instead ahead of a data scientist.
- What should ultimately guide the size of your data team is the number of business problem statements and the complexity of the most serious problems. Look at the size of your roadmap and establish how many people you need to complete your data projects within a reasonable amount of time. If you realize it would take more than a year for your data team to complete its projects, then it's probably time to expand the team. We also encourage you to look at your run vs build ratio. Members of your data team 'run' when they work on daily business operations, focusing on the present performance of the organization. They 'build' when they work on long-term projects, such as adding new features to the product. Your data team should be running 2/3 of the time and building 1/3 of the time. If your data team spends all its time focusing on day-to-day needs, you are jeopardising the future of your company, and it is probably time to expand the team.
- Finally, you might have to make some project-specific hirings. If you're a fintech conducting a project on fraud detection, or a company specialising in dispatching for logistics, you might want to hire someone who knows the specifics of your industry.
How does the data team integrate with the company?
There is no perfect structure for an analytics team, and your structure is likely to change many times. If your data team structure hasn’t changed for the past 2 years, then it’s likely to be a sub-optimal structure. Why? Because the data needs of your company are evolving rapidly, calling for an adaptation of your data team’s structure. Also keep in mind that the more static your organization, the harder the next change will be. For this reason, we don't prescribe a given structure, but rather present the most common models and how they can be suited to different types of businesses.
The very first step to take when structuring your data team is to find the data people that already exist in your organization. They might not be just the people with the term "data" in their title, but they could be any employee who's not afraid of data analysis or has SQL skills already, such as business analysts/ ops analysts. If you don’t take the time to locate pre-existing data people carefully, you are likely to end up with an unplanned data team structure, unlikely to fit your business needs.
Centralized model

The centralized model is the most straightforward structure to implement, and it is usually the first step for companies who aim to be data driven. There are, however, a few drawbacks to this model, which are referenced below. This structure usually leads to a centralized data "platform", where the data team has access to all the data, and services the whole organization in a variety of projects. All data engineers, analysts and scientists within this team are managed directly by the head of data. With this structure, the data team is reporting in a dotted line to data stakeholders based in business units, in a consultant/client-type relationship.
This flexible model is adaptable to the continuously evolving needs of a growing business. If you're at the beginning of your data journey, that is, you still struggle to have a clear vision of your past and present, this is the structure we recommend. The data team's first projects will seek to bring visibility to the business, ensuring all departments in your organization have KPIs and dashboards they can trust. This kind of structure is particularly good for analytics where reusability and data governance are important.
Advantages
✅ The data team can help with other teams' projects while working on its own agenda.
✅ The team can prioritise projects across the company.
✅ There are more opportunities for talent and skillset development in a centralized team. In fact, the data team works on a broader variety of projects, and data engineers, scientists and analysts can benefit from their peers's insights.
✅ The head of data has a centralized view of the company's strategy and can assign data people to projects that are the most suited to their capabilities.
✅ Encourages career growth, as data engineers, scientists and have clear perspectives of seniority roles.
Drawbacks
❌ High chance of disconnect between the data analytics team and other business units. In this model, data engineers and data scientists are not immersed in the day-to-day activities of other teams, making it difficult for them to identify the most relevant problems to tackle.
❌ Risk for the analytics group to be reduced to a "support" function, with other departments not taking their responsibilities.
❌ As the data team serves the rest of the business, other business units might feel like their needs are not properly addressed, or that the planning process is too bureaucratic and slow.
Decentralized / Embedded model
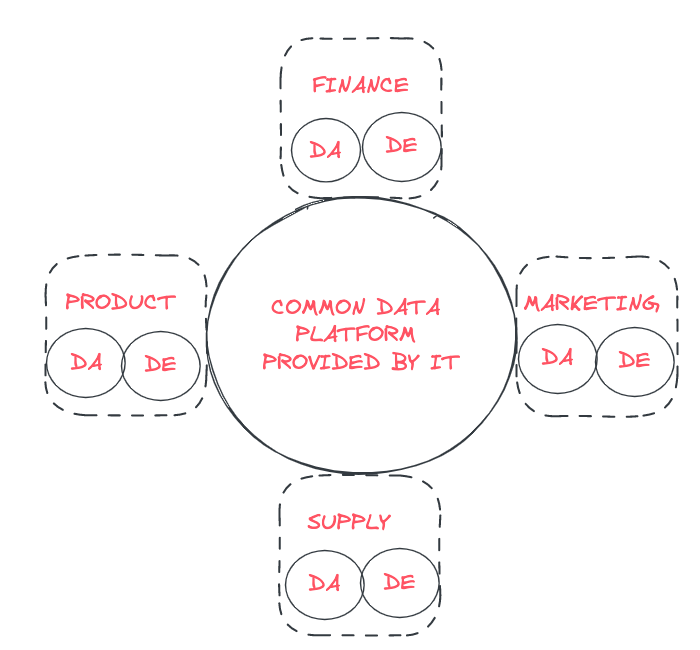
In a decentralized model, each department hires its "own" data people, with a centralized data platform. In this model, data analysts and scientists focus on the problems faced by their specific business unit, with little interaction with data people from other areas of the company. With this structure, data analysts report directly to the head of their respective business unit.
Advantages
✅ Embedded teams of data people are agile and responsive, because they are dedicated to their respective business functions and have good domain knowledge.
✅ Product managers can assign data tasks to the people most qualified to work on them.
✅ Business data teams don’t have to fight for resources to build their data project because the resources sit in the teams.
Drawbacks
❌ Lack of source of truth, duplication of data content
❌ Data people end up working on redundant issues due to a lack of communication between different teams.
❌ The creation of silos leads to productivity erosion since data people can't draw on their colleagues’ expertise as they do in the centralized model.
❌ This model makes it harder to optimally staff data people on different projects.
❌ Business managers, usually lacking technical backgrounds, will find it hard to manage data people and understand the quality of their work.
Federated model / Centre of excellence
A federated model is most suited to companies that have reached data maturity, have a clear data strategy and engage in predictive analytics.
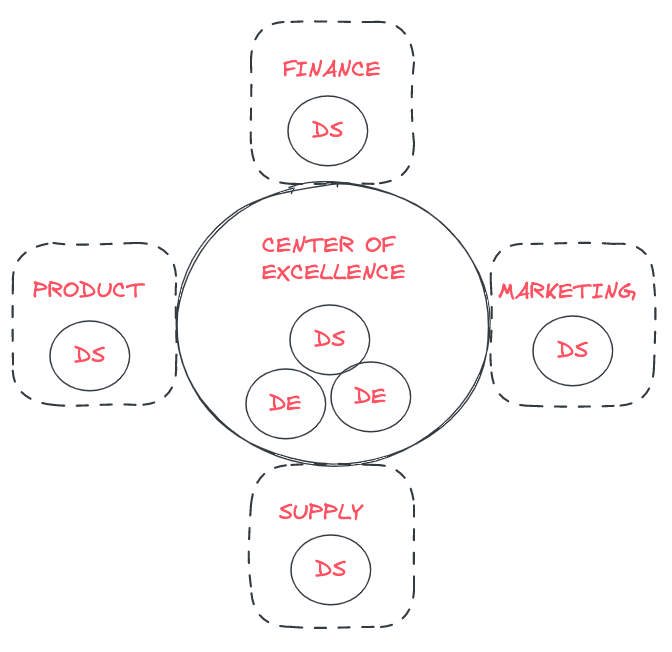
In the Centre of Excellence model (COE), data people are embedded in business units, but a centralized group that provides leadership, support and training remains. If data analysts and scientists are deployed across business departments, you would still have a data leader (or a core of data leaders according to company size) who prioritizes and supervises data projects. This ensures that the most beneficial data projects are tackled first.
This strategy is most suited to larger, enterprise-scale companies with a clear data roadmap. The centre of excellence model entails a larger data team, as you need data scientists both in the COE and in the different business branches. If you are a small or medium company, your needs might not require a data team of this size.
This approach retains the advantages of both the centralized and the embedded model. It is a more balanced structure in which the data team's actions are coordinated, but also keeps the data experts embedded in business units.
Again, it's extremely important that you know who your data people are. When building a centralized team at the beginning of your data journey, make sure you don't have business analysts/ops embedded in other departments. Otherwise, you will end up with an unwanted mixed model, creating complete chaos in your organization. When creating a COE, you need to ensure it's wanted and planned.
Advantages
✅ The Centre of Excellence model provides the advantages of both the centralized and the embedded models.
It still presents some drawbacks, though:
Drawbacks
❌ This model requires an additional layer of coordination and communication needed to ensure alignment between COEand business units.
❌ Not fit for purpose for small – medium sized organizations, so these companies can then hook it to the benefits that can come with this hub and spoke model.
Final words
Building a strong analytics team is a key pillar you need to build if your company is to become data-driven. The extent to which you will extract business value from data ultimately depends on the strength of this team, and how symbiotic it is with the rest of your business. There is no made-to-order advice for the size, composition and structure of your data team. That's why you need to understand the data maturity level of your organization, so that you can build a data team suited to your business' needs and aligned with your business strategy.
Subscribe to the Castor Blog
About us
We write about all the processes involved when leveraging data: from the modern data stack, to data teams composition, to data governance. Our blog covers the technical and the less technical aspects of creating tangible value from data.
CastorDoc is an AI assistant powered by a Data Catalog, leveraging metadata to provide accurate and nuanced answers to users.
Our platform integrates advanced governance, cataloging and lineage capabilities with a user-friendly data assistant, creating a powerful tool for enabling self-service analytics. Don’t wait to turn data into business decisions - Try CastorDoc today.
You might also like
Get in Touch to Learn More
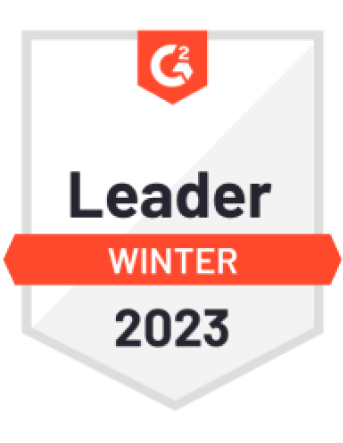
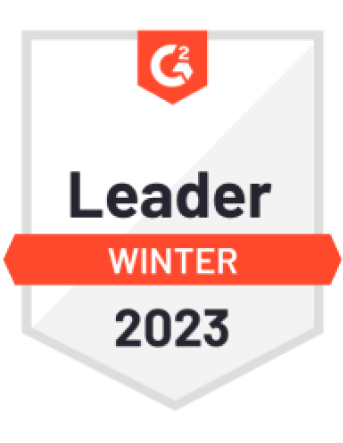
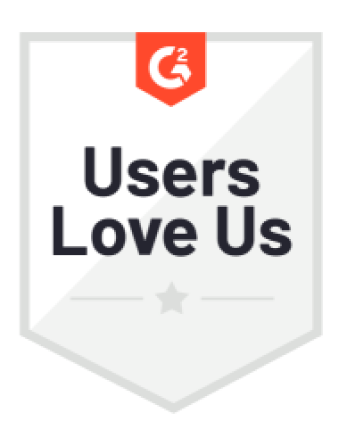
“[I like] The easy to use interface and the speed of finding the relevant assets that you're looking for in your database. I also really enjoy the score given to each table, [which] lets you prioritize the results of your queries by how often certain data is used.” - Michal P., Head of Data