Gartner Summit 2024 Highlights: Building Effective AI Strategies
3 Gartner Summit takeaways: practical steps for building effective AI strategies
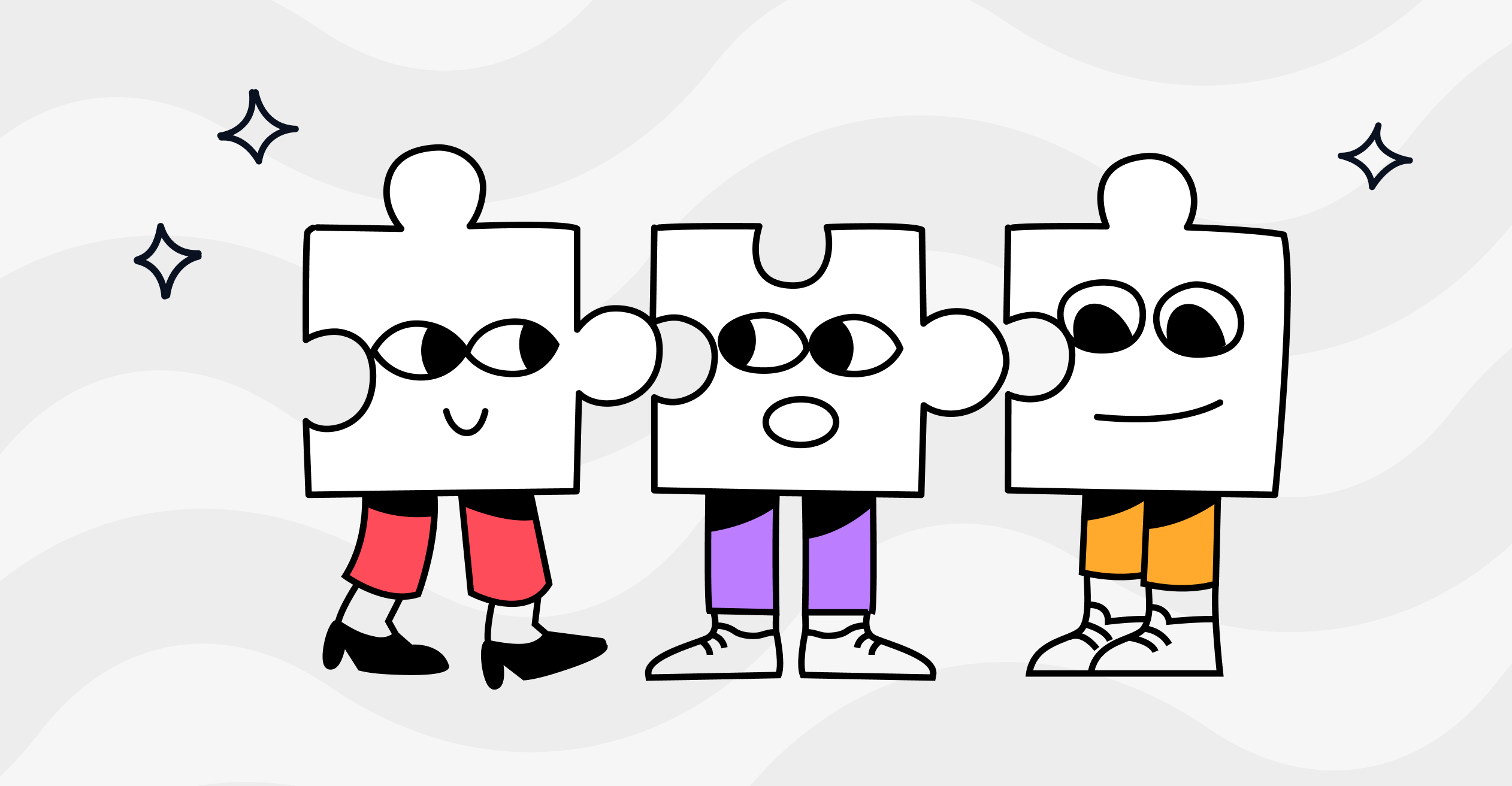
Introduction
What happens when over 5,000 analytics leaders, practitioners, and service providers converge at Disney World for a three-day summit? Something powerful.
As organizations embark on ambitious data & AI journeys - gatherings like these are pivotal. This was the case for this year’s edition of the Gartner Summit.
The Gartner Summit spans over three days and includes 200+ keynotes. While absorbing this wealth of knowledge is exhilarating, translating it into concrete actions can be daunting.
At CastorDoc, with our focus on enhancing our AI Assistant, we zeroed in on presentations that matched our mission and came away with valuable insights. Three talks that stood out for their direct relevance and practical advice:
- AI Readiness & Data Leadership - Debra Logan & Ehtisham Zaidi: This session broke down what makes for strong data leadership and the crucial steps to get AI-ready.
- Effective Strategies for Implementing Generative AI Across Enterprises - Arun Chandrasekaran: A guide through the complex but promising world of GenAI, from start to full-scale use.
- Understanding AI Regulations and Preparing for Compliance - Lydia Clougherty Jones: An insightful comparison of AI regulations around the world, key for staying compliant.
This article distills these three discussions, aiming to equip leaders with actionable insights for their AI strategies. We're sharing this knowledge in hopes it empowers you to fully leverage AI's potential—much as it has guided us in creating an AI assistant that truly democratizes data usage.
I - AI readiness and Great Data Leadership
“Organizations with advanced D&A maturity enjoy a 30% higher financial performance than their peers.” - Gartner
This year's opening keynote was led by Debra Logan and Ehtisham Zaidi, whose expertise lies in strategic topics within the Data and Analytics team, as well as in data management. The session addressed two topics: identifying the hallmarks of outstanding data leadership and mapping the route to AI readiness
The keynote began by revisiting the motivation for gathering over 5,000 analytics leaders, practitioners, service providers, and analysts at Disney World: Gartner reports that organizations with advanced D&A maturity enjoy a 30% higher financial performance than their peers. A good reminder that our efforts in this ecosystem are clearly driving substantial business value.
Having established this foundation, we turn to the next questions: What defines exceptional data leadership? And how can data leaders prepare their organizations for AI readiness?
A - Successful Data Leadership: 3% Strategy, 97% Execution
.png)
Debra Logan and Ehtisham Zaidi opened the discussion on what characterizes a truly effective D&A leader, highlighting the criticality of prioritizing execution over perfecting strategy.
They pointed out a common pitfall among leaders: the paralysis by analysis, where the research for the perfect strategy and data creates an endless strategizing look and impedes actions. Echoing Richard Wieselfors' perspective, they advocated for a pragmatic approach: successful data leaders should adopt a balance of “3% strategy and 97% execution.”
B- Getting your Data AI Ready
.png)
With a clear understanding of data leadership in place, the keynote shifted focus to the issue of AI readiness. The speakers offered some insights on the prerequisites for preparing your data landscape for serving AI initiatives.
- AI readiness stems from an iterative process where metadata plays a critical role in measuring, qualifying, and governing data.
- Metadata management is crucial for AI readiness, enabling the crafting of source lineage to mitigate bias, thus fostering trust.
- Data Observability also plays a key role in AI-readiness - and involves monitoring the health and reliability of data throughout its lifecycle.
- Finally, effective data governance liberates innovation, allowing for safe exploration and innovation within the data sphere.
With the stage set, let’s move on to the second part on how to scale GenAI across the enterprise.
II - Best Practices for Scaling GenAI Accross the Enterprise
“Through 2025, at least 30% of GenAI projects will be abandoned after proof of concept (POC) due to poor data quality, inadequate risk controls, escalating costs or unclear business value” - Gartner
Another talk we will remember was delivered by Arun Chandrasekaran a Gartner Expert specializing in GenAI. He summarized key points from his research paper, "10 Best Practices for Scaling Generative AI Across The Enterprise," into a focused 30-minute talk worth re-transcribing.
According to Chandrasekaran, the ultimate objective of AI is to "democratize access to knowledge and skills across the enterprise via user-friendly language interfaces"—a vision that resonates deeply with us at CastorDoc and has been a driving force behind the development of our AI assistant.
While AI carries transformative potential, it also comes with its share of complexities. It’s crucial to navigate the deployment of AI solutions with due diligence. Below are 10 actionable steps you can put in place to do so.
.png)
10 Strategies for GenAI Implementation:
- Prioritize Use Cases Continuously: Establishing a continuous prioritization process ensures alignment with the organization's AI ambitions and guards against distractions by the most appealing demos or vendor influence. Develop a framework to assess and track business value, rigorously testing each use case during the pilot phase and evaluating its benefits post-deployment.
- Decide Between Build vs. Buy: Understand whether GenAI will be an in-built feature within applications or if it involves embedding LLM APIs from cloud-based models into custom workflows. This choice significantly affects ownership costs, output quality, security, and privacy control.
- Conduct Pilot Tests for Scalability: Many GenAI initiatives fail due to the absence of pilot testing or ignoring pilot phase warnings. Pilots enable realistic environment simulations and organizational learning. Adopt an agile approach, creating a "model garden" sandbox for safe experimentation and model evaluation.
- Design a Composable Platform Architecture: Choose between a single-vendor AI platform or a custom-built GenAI platform. This decision influences market speed, vendor dependence, and scalability. Emphasize flexibility and minimize technical debt by decoupling models from the engineering and UX layers, allowing easy model updates.
- Emphasize Responsible AI: Highlight the importance of responsible AI to mitigate new risks related to compliance, reputation, and intellectual property. Establish and communicate clear principles across fairness, bias mitigation, ethics, risk management, privacy, sustainability, and compliance.
- Enhance Data and AI Literacy: Prepare every employee for direct GenAI usage by emphasizing AI literacy and addressing AI-related fears and misconceptions. Personalized training and open discussions about AI’s impact are crucial for broad deployment.
- Implement Strong Data Engineering Practices: Despite the general-purpose nature of GenAI models, their value is amplified when coupled with organizational data, emphasizing the need for high-quality data practices.
- Foster Human-Machine Collaboration: Optimize GenAI tools for human workflows to overcome mistrust and improve collaboration. Establish "human in the loop" processes and communities of practice for GenAI to share knowledge and enhance mutual collaboration.
- Apply FinOps Practices: Address the cost implications of scaling GenAI with FinOps principles to monitor and manage expenses actively. Utilize auditing tools and effective prompting techniques to control costs.
- Adopt a Product Mindset: Given GenAI's rapid evolution, adopting a product approach ensures continuous improvement and responsiveness to user feedback. This mindset is vital for adapting to changing technologies and user expectations.
Arun emphasized humility and open-mindedness as essential qualities for navigating the GenAI landscape, underlining the importance of evolving strategies based on ongoing feedback.
III - Navigating the new AI and GenAI regulatory dynamics
“Generative AI, which creates new outputs like text, images, and code from data patterns, faces significant regulatory scrutiny. This scrutiny is crucial as the outputs can range from innovative to controversial, such as deep fakes.” - Gartner.
The last session that caught our attention was around AI regulations. If this topic can sometimes be dreadful - Lydia Clougherty Jones, Sr. Research Director at Gartner, talked about it in a lively and intelligent manner.
Clougherty Jones compared the AI regulations of three pioneering locations in AI research: the United States, the European Union and China. The regulations vary among these three countries due to their unique fundamental principles. Since each country aims to protect its core values through its regulations, differences in these principles result in distinct regulations in each location.
We highly recommend watching the full session - but for the purpose of this article, here is a summary of the regulations for each location, and some key recommendations for compliance.
“By 2027, the productivity value of AI will be recognized as a primary economic indicator of national power” Gartner
A - United States Approach: Executive Order and Implementation
.png)
Key Regulations
The U.S. has adopted an Executive Order on AI, mandating enforceable actions to balance AI's benefits against its risks. This is a flexible approach allowing organizations to use GenAI but with the appropriate harm reduction. The idea of the regulation is to temper the high-risk GenAI use cases instead of forbidding them altogether, as in Europe. The goal of this approach is to help the US keep a competitive advantage in the realm of GenAI, while mitigating high-risk use cases.
Impact
The approach aims to ensure AI's responsible use within the government and the private sector, requiring transparency, safety testing, and mandatory disclosures. Agencies must appoint a chief AI officer to guide AI strategy, emphasizing the nation's leadership in ethical AI development and application.
Navigating the regulations
Below are the main elements that you should focus on to ensure you develop GenAI systems that are compliant with the US regulations.
- Appoint a Chief AI Officer: Essential for overseeing AI governance and ensuring compliance with U.S. regulations, especially for organizations working with federal agencies.
- Integrate Privacy Technologies: If your AI tools are used by government agencies, embed privacy-enhancing technologies to align with the executive order’s safety and privacy requirements.
- Mitigate High-Risk Use Cases: Identify and address high-risk AI applications within your organization, focusing on harm reduction in compliance with U.S. guidelines.
- Comply with Reporting Requirements: Adhere to safety testing and disclosure mandates for large-scale computing clusters and dual-use AI models, reporting as required to the federal government.
B - European Union Approach: The EU AI Act
.png)
Key Regulations
The EU AI Act introduces a risk-based regulatory framework for AI, categorizing applications by their potential risk to society. It imposes strict prohibitions on AI systems considered to pose an "unacceptable risk" to people's fundamental rights, including indiscriminate biometric surveillance in public spaces and AI-driven emotion tracking in the workplace. The Act mandates transparency and informed consent for users interacting with AI, such as chatbots or when exposed to deepfake content.
Impact
Short-term, the Act may challenge the competitiveness and development pace of AI within Europe due to its stringent requirements. However, long-term, compliance promises a global competitive advantage, setting a benchmark for responsible AI use that prioritizes social responsibility and human rights.
Navigating the Regulations
Here are the key components to prioritize for developing GenAI systems that adhere to the EU regulatory standard:
- Risk Classification: Evaluate and classify your AI applications based on the EU's risk framework, focusing on avoiding "unacceptable risk" categories.
- Transparency and Consent: Implement measures to inform users about the use of AI, such as chatbots and deepfakes, and ensure consent is obtained where necessary.
- Law Enforcement Exemptions: If applicable, understand and comply with exceptions for biometric systems used by law enforcement, ensuring adherence to specific conditions.
- Embrace Responsible AI: Align AI strategies with EU standards for responsible use, positioning your organization for long-term global advantages.
Dive deeper → Your Guide to the EU AI Act.
C - People’s Republic of China: Towards a Comprehensive Framework
.png)
Key Regulations
The regulatory landscape for AI in China adopts a targeted approach, addressing AI risks within specific areas such as algorithms, deep synthesis technologies, and Gen AI. This strategy has led to the identification and prohibition of certain AI uses, signaling a move towards more comprehensive regulation in the near future. This evolving framework indicates China's cautious yet progressive stance on managing AI's societal impacts, with an eye on both innovation and risk mitigation.
Impact
The regulatory framework aims to secure a safe digital environment while bolstering China's economic strength through AI. By enforcing ethical guidelines and data security measures, China seeks to ensure that AI contributes constructively to society and maintains high standards for technological ethics and user protection.
Navigating the Regulations
To align with China's AI regulations, here are a few elements you can focus on:
- Review Mechanisms: Conduct thorough reviews of algorithms and technological ethics, ensuring compliance with specific AI risk areas identified by China.
- Data Security and Ethics: Strengthen data protection and uphold ethical standards in content, particularly in protecting minors and promoting positive content.
- Transparency and Content Control: For deep synthesis AI, ensure clear content labeling and management, and maintain high standards of transparency and technical security.
Conclusion
As organizations get ready to test and deploy GenAI use cases - the Gartner Summit was a fertile ground for conversations focused around leveraging the technology effectively and responsibly.
This article distilled three key presentations that align with our direction at CastorDoc. Collectively, they chart a course for preparing your data for AI, expanding AI's role within your enterprise, and navigating the AI regulatory framework while maintaining compliance.
At CastorDoc, we've crafted an AI assistant underpinned by our Data Catalog, leveraging metadata for precise, context-aware responses.
Our platform merges sophisticated governance and cataloging with an intuitive data assistant, creating a powerful tool for enabling self-service analytics. Explore AI applications or to experience our AI assistant firsthand, our team is ready to exchange.
You might also like
Get in Touch to Learn More
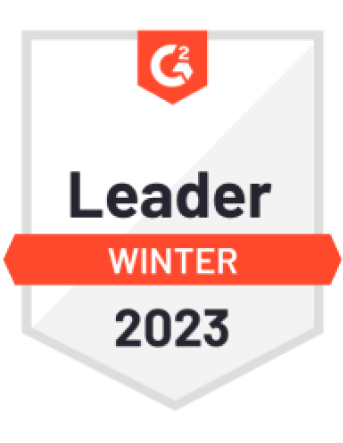
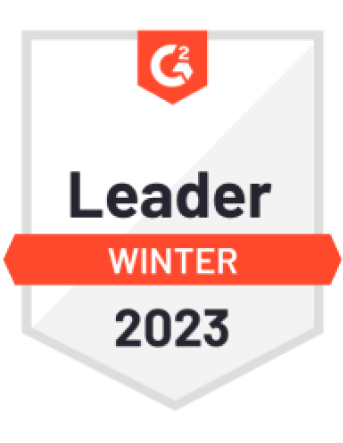
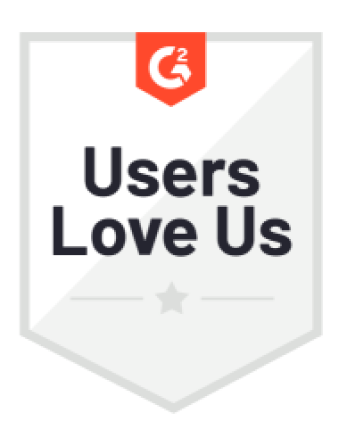
“[I like] The easy to use interface and the speed of finding the relevant assets that you're looking for in your database. I also really enjoy the score given to each table, [which] lets you prioritize the results of your queries by how often certain data is used.” - Michal P., Head of Data