Modern Data Catalogs are AI Augmented
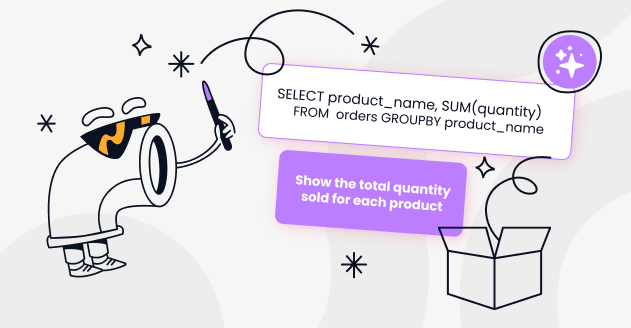
Artificial intelligence is revolutionizing the way we manage and utilize data, and one area where it is having a significant impact is in data catalogs. Modern machine learning augmented Data Catalogs are automating metadata discovery and profiling, which is improving the way we search for, discover, and trust our data.
The use of AI in data catalogs is particularly beneficial in three key areas: search, detect, and automation.
I - Machine Learning-Powered Search: How AI is Helping Data Teams Find and Trust Their Data
Data catalogs serve as a central repository for data assets within an organization, enabling data teams to search, discover, and share data assets with other stakeholders. As the volume of data continues to grow exponentially, the traditional approach of manual cataloging is no longer feasible, and organizations are turning to modern machine learning augmented Data Catalogs to automate metadata discovery and profiling.
Machine learning algorithms are particularly useful in automating search and discovery of data assets. AI-driven search and discovery of data assets is becoming increasingly important as organizations struggle to keep pace with the growing volume of data. Augmented data catalogs leverage machine learning algorithms to browse through thousands of possibilities and rank results based on various factors such as popularity, history, relationships, quality, and more.
With the help of AI, data teams can trust that they are finding the most relevant data and making informed decisions based on accurate and reliable information. Moreover, modern Data Catalogs provide AI-powered search and discovery of data assets, including recommendations. This is similar to how Google ranks results when a user types in a query. Augmented data catalogs rank the search results based on factors such as popularity, history, relationships, quality, etc. This helps users find the data they need faster and enables them to discover related data assets they may not have known existed.
Additionally, modern Data Catalogs provide AI-powered recommendations based on user behavior and interactions with the data. They learn from user preferences, feedback, and other data points, and then suggest relevant data assets to users. This not only helps data teams find the data they need but also enables them to discover related data assets they may not have known existed.
II - AI is Enhancing Data Governance and Security
In addition to AI-driven search and discovery, modern Data Catalogs are also leveraging AI to establish semantic relationships between data using knowledge graphs. A knowledge graph is a data structure that contains nodes, edges, and attributes, where nodes represent entities, edges represent relationships between entities, and attributes provide additional information about the entities.
With the help of AI, modern Data Catalogs can analyze and understand the relationships between different data assets, providing data teams with a better understanding of the data they are working with. This enables data teams to make more informed decisions about data usage, data sharing, and data governance.
Moreover, modern Data Catalogs provide data anomaly detection to identify sensitive PII information, flagging risky data assets, and outliers. Anomalies can arise due to a variety of reasons, including human error, system failure, or malicious intent. With the help of AI, modern Data Catalogs can detect anomalies and notify data teams, enabling them to take corrective action before any serious harm is done.
For example, modern Data Catalogs can analyze data assets and flag any data that contains sensitive information such as Personally Identifiable Information (PII), financial information, or health information. By detecting sensitive information, data teams can ensure that they are complying with data privacy regulations and protecting sensitive information from unauthorized access or use.
III - Automating Data Management: How AI is Streamlining Data Discovery, Tagging, and Collaboration
Perhaps the most significant impact that AI is having on data catalogs is in the area of automation. Machine learning augmented Data Catalogs enable the pervasive use of metadata not just for Data Governance but also to automate data integration, data preparation, data quality, and many other data management activities.
By automating data management activities, AI-powered Data Catalogs can accelerate time to insights by helping data teams automate most of the data discovery, tagging, propagation, and collaboration. This frees up data professionals to focus on more strategic initiatives such as data analysis, modeling, and visualization.
For example, modern Data Catalogs can use AI to automate data tagging, which involves attaching metadata to data assets to make them more easily discoverable and understandable. With the help of AI, modern Data Catalogs can automatically tag data assets based on their content, context, and usage, enabling data teams to find the data they need faster and with greater accuracy.
Moreover, modern Data Catalogs can automate data propagation, which involves updating metadata across multiple systems to ensure consistency and accuracy. With the help of AI, modern Data Catalogs can propagate metadata automatically, enabling data teams to maintain data quality and consistency across the organization.
If you want to know more about plug-and-play, collaborative, ML oriented data catalogs: check Castor.
Subscribe to the Castor Blog
You might also like
Get in Touch to Learn More
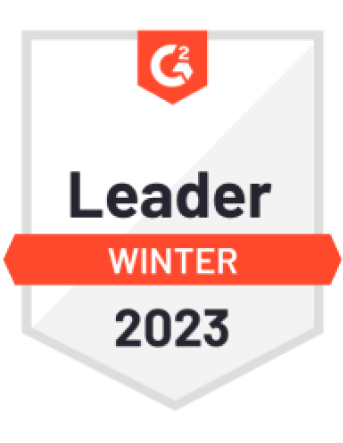
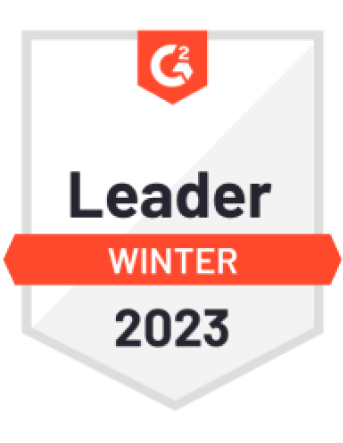
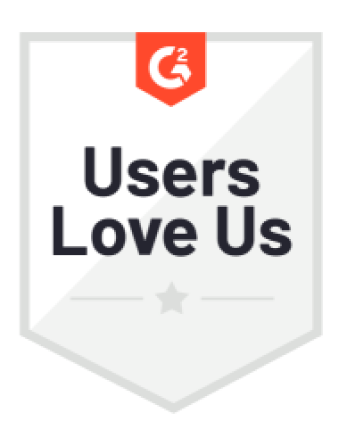
“[I like] The easy to use interface and the speed of finding the relevant assets that you're looking for in your database. I also really enjoy the score given to each table, [which] lets you prioritize the results of your queries by how often certain data is used.” - Michal P., Head of Data