Top 5 Data Quality Metrics You Should Track
Completeness, Accuracy, Consistency, Uniqueness, & Timeliness.

We're all aware that data is the engine driving our businesses. It's the tool we use for smarter decision-making, timely innovations, and staying a step ahead of the market. And we've all been in professional scenarios where data analytics opened the door to new opportunities.
But let's not sugarcoat it, if you're working with data you know this, poor data quality can be a disaster. It's the kind of thing that sends you down a maze of false leads and wasted resources. And it’s not just an inconvenience; we're talking about real financial setbacks. The annual financial hit to businesses from low-quality data can soar up to $15 million, according to a Gartner report.
In this article, we're zeroing in on the data quality metrics that you can't afford to ignore: Completeness, Accuracy, Consistency, Uniqueness, and Timeliness.
What Are Data Quality Metrics?
Data quality metrics are your way of evaluating just how good—or bad—your data is. These aren't just arbitrary numbers; they're the guidelines that tell you if your data is reliable enough to base key business decisions on. Whether you're allocating resources, shaping your next marketing strategy, or dealing with risk assessment, these metrics give you the intel you need.
And this isn't industry-specific; we're talking universal applicability here. From healthcare to finance, ed-tech to insurance, data quality metrics are the common denominator that sets the pros apart from the amateurs.
Top 5 Data Quality Metrics
1. Data Completeness
When it comes to data quality, "Completeness" is your first port of call. This metric essentially tells you if you've got all the pieces to your data puzzle. The "Data Completeness" metric is all about making sure that every expected data field is populated and that you're not missing any crucial bits of information.
Consider the following dimensions of data quality when evaluating "Completeness":
- Missing Values: Check whether every field in your records is filled out. Missing values can compromise the whole analysis.
- Partial Records: Sometimes, you'll encounter records that are half-complete. These partial records can skew your data and, consequently, any derived insights.
- Field-Level Completeness: Are all critical fields like email, phone number, and so forth present and populated? Missing crucial data fields can severely hamper operations like customer outreach or personalization.
To measure "Completeness," you can tally up the empty fields or partial records and express it as a percentage of the total records. Lower percentages mean you've got more complete data, which is where you want to be.
2. Data Accuracy
The data accuracy metric tells you how closely your data mirrors the actual state of affairs. Particularly in sectors like finance, there's no room for gray areas—your data is either spot-on or it's off, and the stakes are sky-high. You've got fines, reputation damage, and bottom-line hits waiting in the wings if your data is inaccurate.
Key facets to look at when evaluating "Accuracy" include:
- Exact Matches: Especially in finance, numbers have to align down to the last decimal. Any discrepancy can lead to significant errors and, frankly, a lot of headaches.
- Threshold Levels: Sometimes, a slight deviation from the actual value might be acceptable within defined limits. Know those limits.
- Logical Consistency: Your data should not just be accurate on a standalone basis; it should also be consistent with related data fields.
To measure "Accuracy," the go-to metric is generally the percentage of correct values relative to the total number of values. The closer you are to 100%, the better.
So, bottom line, if you're not keeping a close eye on "Accuracy," you're basically flying blind—and that's not a position any of us can afford to be in.
3. Data Consistency
"Consistency" in data quality management ensures that data is uniform across multiple systems or platforms. The key data consistency measures include:
- Contradiction Checks: Ensure that identical data fields across different systems align in both value and format.
- Uniform Reporting: Consistent data is essential for accurate cross-functional reporting.
- Standardized Representations: Adopt uniform terminologies for missing or unavailable data across all sources.
To measure "Consistency," perform reconciliation checks between different data repositories. Discrepancies, especially in crucial data fields like financial information, should be immediately corrected. Consistent data is essential for reliable analytics, reporting, and operational efficiency.
4. Data Uniqueness
"Uniqueness" in the data quality context focuses on minimizing or eliminating duplicate records. Key aspects to consider are:
- Duplicate Records: Identifying and removing duplicates is essential for clean data. For instance, having a customer listed twice in a mailing list can distort analytics.
- Process Efficiency: Duplicate records can bloat databases and complicate data processes, affecting efficiency.
To measure "Uniqueness," tally the number of duplicate entries and express it as a percentage of the total records. The goal is to bring this percentage as close to zero as possible. Ensuring data uniqueness is vital for accurate analytics and streamlined operations.
5. Data Timeliness
"Timeliness" measures how current the data in your database is. Here's what you should pay attention to:
- Data Age: More recent data is generally more accurate and relevant, particularly in fields like customer information that change over time.
- Error Propagation: Older data can introduce errors as it moves through various stages of your data pipeline, affecting downstream analytics and decisions.
To track "Timeliness," use timestamps on your data sources. Calculate the age of your data compared to the current date. Based on this, you can decide whether to reverify, update, or archive the data in question. Keeping your data timely ensures its relevance and accuracy, reducing the risk of error propagation.
How To Measure Data Quality Metrics
Measuring the quality of data through these five ways -
- Define Metrics and Benchmarks: Clearly outline which quality metrics are important for your business objectives. Set benchmarks that reflect what acceptable data quality looks like for each metric.
- Data Auditing: Data auditing helps you periodically examine random samples of data for discrepancies or anomalies that could indicate quality issues. Use automated auditing tools for large datasets.
- Use Validation Rules: Implement validation rules for data entry and processing to catch errors before they become ingrained in the system.
- Compare to Reference Sets: If possible, cross-reference your data against authoritative datasets to measure accuracy, completeness, or consistency.
- Monitor Continuously: Make data quality assessment a continuous process. Use dashboards and alerts to immediately flag potential issues, enabling real-time corrections.
Conclusion
By defining your key metrics, auditing your data, setting validation rules, cross-referencing with authoritative sets, and maintaining continuous data observability, you're setting the stage for data-driven success. Remember, high-quality data isn't just a goal to improve data quality once; it's a continuous process that requires commitment. Make that commitment, and you position your business to be more adaptable, compliant, and competitive.
Subscribe to the Newsletter
About us
We write about all the processes involved when leveraging data assets: from the modern data stack to data teams composition, to data governance. Our blog covers the technical and the less technical aspects of creating tangible value from data.
At Castor, we are building a data documentation tool for the Notion, Figma, Slack generation.
Or data-wise for the Fivetran, Looker, Snowflake, DBT aficionados. We designed our catalog software to be easy to use, delightful and friendly.
Want to check it out? Reach out to us and we will show you a demo.
You might also like
Get in Touch to Learn More
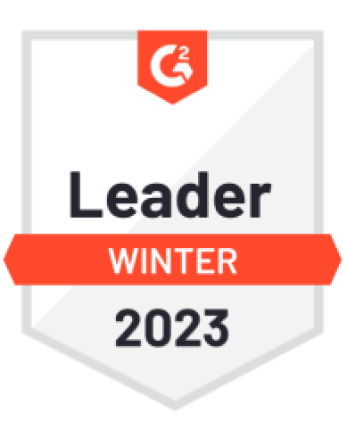
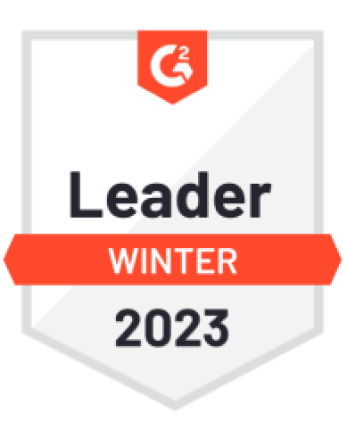

“[I like] The easy to use interface and the speed of finding the relevant assets that you're looking for in your database. I also really enjoy the score given to each table, [which] lets you prioritize the results of your queries by how often certain data is used.” - Michal P., Head of Data