What Is AI Model Governance & Why It's Important?
Explore the components, benefits, and more.

AI's prominence in business decision-making has surged, especially with the recent advancements in generative AI technologies like ChatGPT. McKinsey Digital estimates that generative AI could impact global productivity to the tune of $4.4 trillion annually. That's not pocket change. With over half of CIOs projecting that AI will be either widespread or critical in their organizations by 2025, it's clear that AI isn't just a fleeting trend—it's a strategic imperative.
But let's not forget, AI isn't a silver bullet. It comes with a set of inherent risks—think bias, regulatory compliance, and privacy concerns—that could result in significant legal and reputational damage. This is where AI Model Governance enters the picture. Consider it your risk management playbook for ensuring your AI initiatives are transparent, ethical, and above all, trustworthy.
In this article, we'll unpack the critical role AI Model Governance plays in mitigating these risks and why it should be a priority in your data strategy.

What is AI Model Governance?
AI Model Governance is essentially a structured approach for overseeing AI models from their inception to retirement. As we’re dealing with machine learning models that learn and adapt, this governance framework ensures that they do so within set boundaries that align with business objectives and compliance requirements.
Key Components:
- Version Control: Just like you wouldn't want multiple versions of a financial report floating around, you don't want that with your AI models either. Version control helps you track changes, who made them, and when. This aids in easy rollback, comparison, and, most importantly, accountability.
- Model Validation: Before deploying any model into a production environment, rigorous testing is essential. This includes assessing the model for performance, fairness, and potential bias. Having a validation process as part of your governance framework provides a systematic way to evaluate and approve models.
- Auditing: This is your safeguard against internal errors and external scrutiny. Auditing involves regularly reviewing model performance, data inputs, and outputs. It helps ensure that models are compliant with internal policies and external regulations, and it provides documentation to prove it.
The Importance of AI Model Governance
As AI and machine learning technologies continue to mature, their complexities and the stakes associated with them are growing exponentially. AI Model Governance serves as an essential framework that touches every facet of AI project development and deployment, effectively increasing the project's overall value. Here's why it's critical:
- Risk Mitigation: One of the core functions of AI Model Governance is to manage risks. AI models can inadvertently produce biased or incorrect outputs if trained on biased or flawed data. Governance provides a mechanism to assess and control these risks proactively.
- Performance Monitoring: Governance isn't a one-off activity; it's an ongoing process. Key performance indicators like model speed, accuracy, and drift must be continuously monitored to ensure the model's effectiveness over time.
- Compliance & Regulation: With a governance framework, you can map out the compliance landscape clearly. It helps in identifying whether a particular model is subject to regulations like GDPR or CCPA and ensures that the model complies through its lifecycle.
- Ownership & Accessibility: Governance frameworks also outline the ownership of the model, defining who is responsible for it, and ensuring that only authorized personnel have access. This adds an additional layer of security and accountability.
- Lifecycle Management: From development to post-production, governance offers a structured pathway for model lifecycle management. It provides clarity on what steps are necessary for monitoring models after they've been deployed, ensuring they continue to meet organizational and regulatory standards.
How To Implement AI Model Governance
Let's break down the steps you should consider: Manage, Develop, Deploy, and Monitor.
Manage: Set Up a Model Governance Framework
- Establish a Framework: Before you even start sketching out models or selecting data sources, put together a comprehensive governance document. This should detail procedures, guidelines, and standards that your AI projects will adhere to.
- Identify Roles: Governance is a team sport. Here's your starting lineup:
- Legal: To handle regulatory requirements and data usage policies.
- Management: To oversee resource allocation and project timelines.
- Team Leaders: Data scientists, engineers, developers—you need technical folks to ensure the governance process itself is up to snuff.
- Business Unit Rep: Someone who understands the business objectives and can keep the project aligned with them.
Model Development: Version Control and Documentation
- Log Data Sources: Document every dataset you use. If the data is cleaned or updated, assign a version number to it.
- Algorithm and Model Versioning: Each variant of the algorithms and models used should be documented and versioned.
Deploy: Validation and Testing
- Pre-deployment Checks: Before you push a model to production, it must undergo a thorough inspection. This includes performance tests, drift analysis, and compliance reviews.
- Rollback Plans: Always have the most recent successful version ready to roll back to if the new deployment runs into issues.
Model Monitoring: Continuous Oversight
- Performance Metrics: Once the model is live, it needs to be continuously monitored. Be on the lookout for model drift, resource overuse, and any other abnormalities.
- Automated Alerts: Implement automated alerting mechanisms to notify team leads when the model's performance deviates from set parameters.
What to Look for in an AI Governance Solution
Choosing an AI Model Governance solution? Don't just grab the shiniest tool off the shelf. Here's a checklist to keep you grounded:
- Simplicity and Integration: Look for a solution that's easy to integrate into your existing workflows. The last thing you want is to add more complexity to an already intricate setup.
- Visibility: The governance solution should offer transparent monitoring capabilities, giving both data scientists and business stakeholders insights into model performance and behavior.
- Environment Agnostic: Stay away from solutions that try to lock you into a specific ML development environment. The tool should be compatible with multiple platforms, freeing your data scientists to choose the best tools for the job.
- Practicality over Hype: Be skeptical of solutions that make grand claims. The ML landscape is still maturing, and no single tool can manage the entire lifecycle from development to deployment. Choose practicality over marketing fluff.
- Multi-layered Defense:
- First Layer: For the data scientists, the tool should offer features like model validation and explainability, essential for the developmental phase.
- Second Layer: For production, it should offer real-time monitoring of model behavior to catch issues as they arise.
- Third Layer: For auditors and compliance officers, it should generate detailed reports based on the model’s output data.
6. Avoid Vendor Lock-in: Be wary of solutions that look attractive upfront but eventually leave you stuck with an overpriced, underperforming tool.
7. Focus on Production: While lab-level validation is crucial, the real test of a model’s mettle is in a production environment. Ensure that the governance tool offers robust production-level monitoring without interfering with the work of your data scientists.
Subscribe to the Newsletter
About us
We write about all the processes involved when leveraging data assets: from the modern data stack to data teams composition, to data governance. Our blog covers the technical and the less technical aspects of creating tangible value from data.
At Castor, we are building a data documentation tool for the Notion, Figma, Slack generation.
Or data-wise for the Fivetran, Looker, Snowflake, DBT aficionados. We designed our catalog software to be easy to use, delightful and friendly.
Want to check it out? Reach out to us and we will show you a demo.
You might also like
Get in Touch to Learn More
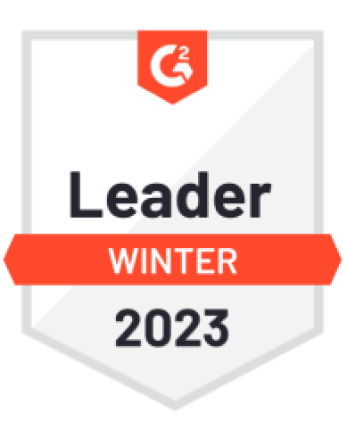
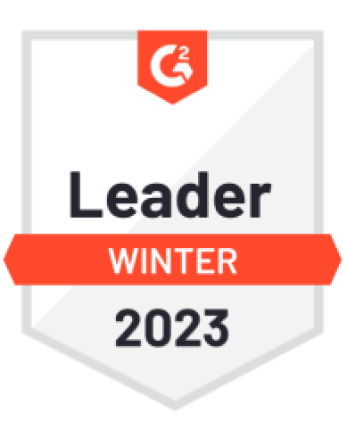

“[I like] The easy to use interface and the speed of finding the relevant assets that you're looking for in your database. I also really enjoy the score given to each table, [which] lets you prioritize the results of your queries by how often certain data is used.” - Michal P., Head of Data