What is Data Product Management?
Get a clear picture of the data product manager’s role and responsibilities, tips to rocking the job, and more.
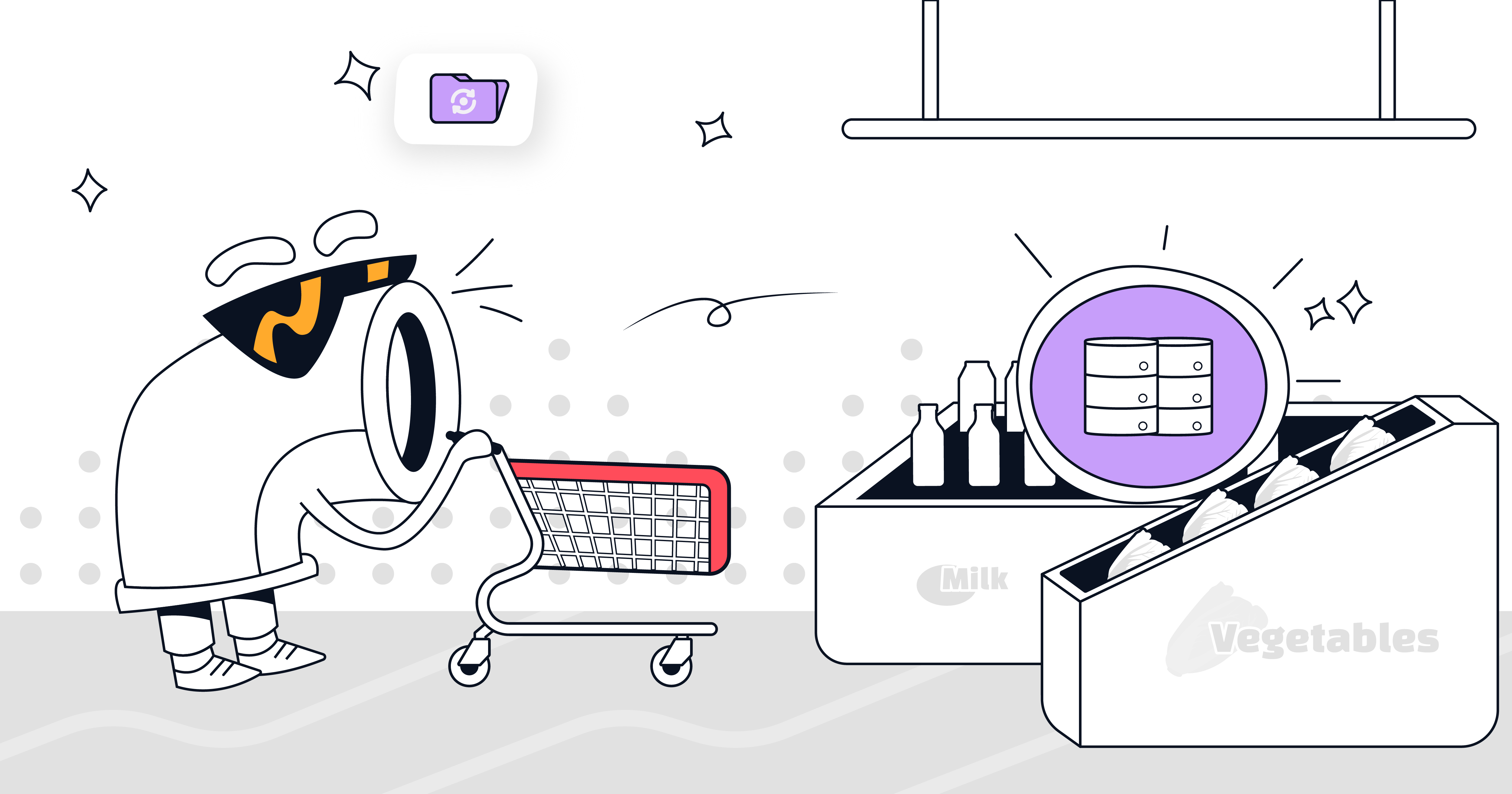
Most people are familiar with what product management is and the role of product managers. Data product management, however, is a new discipline that has recently emerged in the workplace. Data product management is the best approach when it comes to developing data-driven products. This new approach gave rise to a new role in tech companies: the data product manager. This article outlines what data product management is and how it differs from regular product management. What is a typical project conducted by data product managers? What are the skills needed for the job? Does your company need a data product manager? These are the questions this article seeks to answer.
What is data product management?
Data product management aims to strategically develop, launch on the market and, support and improve a company’s data products. Data product management requires focusing on data products and on each user, to design the best products.
What is a data product?
You might be wondering what precisely these data products are. Data products are products that generate value for the end-user thanks to intensive use of data, analytics, machine learning, and other techniques related to data science. For example, a product management project at Spotify could be to develop a new type of personalized playlist that would fit each user's taste. Another example of a data product could be a visual search engine provided as a SaaS through an app. Finally, the product could be a tool for the human resources department that automatically filters job applications.
Role of the data product manager
A data product management project can’t come to an end without a data product manager. The data product manager is key because he ensures the collaboration of people with very diverse profiles and skills within the company. A typical data product team usually comprises front and back-end dev, UI and UX designers, DevOps, data scientists, data engineers, and even Data Ops for the most complex products. Additionally, the data product manager should represent each user's voice to design a product that responds to users' needs.
Structure of a data PM team
A data product team can be divided into two poles. The first pole is mainly in charge of model performance. The second pole is primarily in charge of activation performance. In other words, the first pole has to transform the raw data, build a model around the refined data and tune the model to get the best forecast possible. This pole is mainly composed of data specialists. The second team has to create the best activation, i.e. the mechanism through which the prediction will bring value to the user. It encompasses the delivery of the model's output (the display of recommended videos) and the activation of that output (the act of clicking on one of the videos to watch it). This part is mainly the responsibility of the development teams.

These two poles cannot work separately. The activation depends on the chosen model. Reciprocally, the model depends on the activation. For example, product recommendations have to be displayed almost instantly on an e-commerce platform. This activation constraint (time constraint) immediately sets some requirements for the model. Therefore, a good data product manager is able to deliver the best products by understanding and coordinating these two poles.
Product Manager vs Data Product Manager
After reading the previous section, you might be wondering whether there are any differences between traditional product managers and data product managers. In fact, the two roles differ way more than you think.

Similarities between the two jobs
A data product manager also has some more classical duties like a regular product manager would have. Challenge number one for both data product managers and product managers is prioritization. In other words, both PMs have to decide what problems have to be tackled first to develop the best product. To do so, both PMs will have to build roadmaps, compile backlogs, present release plans, develop business cases, and design the metrics that define the product's success.
Still two very different jobs
Overall, while a regular product manager is at the intersection of engineering, business, and UX, a data product manager is at the intersection of engineering, business, and data!
A regular product manager focuses on fulfilling its users’ needs through his product. A regular product manager will try to close the empathy gap with its users to design the best product. Thus, data is often only used in a second time to validate the features brought to the product and to clarify insights. For this reason, product managers are not obliged to know every detail about data (knowing how to understand common visualization, how to interpret user feedback, and how to perform A/B testing is enough).
On the other hand, a data product manager will tell you that data wins the argument. In other words, a data product manager will mainly make decisions based on quantitative assessment and experiments. Moreover, as mentioned before, data always fuels data product managers' products. Therefore, data product managers should always be up to date with data science’s state of the art to accurately understand and manage their team. Among the numerous tasks that a data product manager can supervise, here are a few examples:
Discovering new data sources: identifying new data sources that could be useful for the business (for example, competitor data)
Serving data: creating new data pipelines to fulfill other departments' needs
Optimizing existing process: switching from manual ID recognition to automated recognition
Implementing new feature: cross-selling product recommendation
Introducing new internal metric: providing new forecasting model to the finance department for advanced analytics
Continuous news and research watch: being aware of any new tools, models, and trending topics in the field of data science
A concrete example of a project led by a Data PM: Cross-selling product recommendation
At this point, you should have a better idea of what data product management is and how it differs from regular product management. However, it might be interesting to look for a concrete and detailed project that a data PM could lead. This section gives an example of a project by showing how a data PM can implement cross-selling product recommendations on an eCommerce platform.
Cross-selling product recommendation: cross-selling is a sales technique used to get a customer to buy additional products that are related to the products that are already being bought (i.e., products in the shopping cart). Many studies show that cross-selling product recommendations increased the amount spent by customers on e-commerce platforms. Therefore, this type of recommendation is crucial for e-commerce platforms. Adding such features to an e-commerce platform is a typical product management project. The project requires building a recommendation engine. Recommendation engines heavily rely on data science techniques such as matrix factorization or on some deep learning tool.

The typical roadmap for building a product is divided into sprints. In short, a sprint is a period where ideas are turned into concrete action. Usually, a sprint lasts one month. The end of a sprint is always followed by an interaction phase where the previous goals are updated, and new goals are set for the following sprint phase. You are now going to see how a data PM can develop a product over five sprints.
Sprint 1 – Analysis work to answer fundamental questions:
- Are current product recommendations efficient?
- Would cross-selling recommendations significantly improve the platform's net revenue?
The data PM and his team formulate hypotheses for each question. Then, the team answers each of the two questions using past data. Each answer might as well generate sub-questions. The team should answer these sub-questions until self-evident truths are found.
Sprint 2- The analysis led in the first sprint usually brings significant findings. Let’s say, the team finds that the platform would benefit from more accurate product recommendations and, more particularly, from product cross-selling recommendations.
In this case, the data PM decides to build an MVP (a small recommender system that enables cross-selling) to collect as much data and feedback as possible. The team also tries to find where to put these new recommendations on the website by testing it on current users. The data PM formulates new hypotheses to be tested. The data engineering team provides all the data needed for the recommender. The data science team builds a recommender (MVP). The frontend team performs A/B testing on actual users to find the best location for the recommendations.
Overall, during this sprint, the different teams create and build metrics, selecting the best ones to optimize the recommender's usage. The optimization is achieved through great analytics of these metrics, and it can take some time and numerous iterations before reaching the right product features.
Sprint 3- Let's say the results from the previous sprint are significant but not outstanding. In other words, cross-selling recommendations, on average, increase the net revenue. However, the increase isn’t considerable. Moreover, say the frontend team locates two places where the recommendations would have the best impact. The first location is on the product page, and the second is on the checkout page.
Despite the slight increase in net revenue, the data PM should be confident that the increase will be greater during this sprint phase, thanks to the improved recommender system. The data PM now asks data scientists to finalize the recommender system by the next sprint. Additionally, the data PM asks the frontend team to focus on two major hot spots for the A/B testing: the product and the checkout pages.
Sprint 4- The expected increase in net revenue is significant on both locations (product and checkout pages). Moreover, the team has enough data to compute a very important metric: user retention. A retention curve shows the percentage of users lost over time while using the product. Analytics clearly shows that users with access to the new recommendations have a better retention curve than those who did not have access. Finally, the recommender is now fully functional.
The data PM can now decide to deploy the cross-selling recommendation for all users.
Sprint 5- The data science team regularly monitors the model's performances and makes additional checks.
Overall, thanks to this example, you saw how the data PM goes from a simple investigation (are my product recommendations efficient?) to the development of a fully new data product (cross-selling recommendations). You also noticed that during sprint 3 the data PM chose to continue the product development, although the results were not as good as expected.
This is because each feature of data products is usually the fruit of many iterations. Moreover, during sprint 3, the data PM was also able to drive its team toward the best product by focusing on a specific feature that would activate on precise website location during sprint 3.
The skillset of a data product manager
I hope that you have learned interesting aspects of data product management in the previous sections. Maybe some of you already want to make a career change and become data PMs and are wondering how to become one. The following section describes the skills needed to become a data PM.
- Master the life cycle and development of data products
First of all, you should perfectly handle the lifecycle of data products. You should always keep in mind how your products are going to impact the business. Moreover, during each of the sprint phases, you should know what action to take and which teammate to solicit for a given task. Things to be done during sprints include the following: data preparation, hypothesis and modeling, evaluation and interpretation, deployment, operation, optimization, and launch.
- Sharp technical knowledge
Secondly, you should hone your technical and analytical knowledge. A data PM should have knowledge of computer science, statistics, data analytics, science, and the tools associated with these disciplines. For example, a data PM should know the pros and cons of each machine learning model. Moreover, as mentioned earlier, a data PM should constantly be learning new techniques to deliver the best products. Additionally, data PMs are problem solvers. Therefore, you will need great analytical skills to choose how to tackle the issues that you will face. Finally, this knowledge should always be kept up to date. If you plan to become a data PM, it's thus best if you plan some free time to digest new knowledge.
- Develop emotional intelligence
Thirdly, you will need to develop emotional intelligence. Emotional intelligence is at the nexus of self-awareness, self-regulation, motivation, empathy, and social skills. Great emotional intelligence is of the utmost importance for your products and your team. First of all, you will close the empathy gap with your user thanks to emotional intelligence. This will result in better products that will truly fulfill customers’ needs. Secondly, great emotional intelligence will positively impact your leadership, collaboration, and interactions. This will result in a more effective team and a healthier working environment. Finally, having this skill will allow you to give and receive quality feedback. Feedback is key to improving user experience and learning.
Overall, the skills required for data product managers are very diverse, and there is a very steep learning curve for this type of job.
Do you need a data product manager?
You might be in a decision-making position and wondering whether you need a data PM. In this section, you will find guidelines to figure out if you need a data PM.
The first question you should be asking is whether data fuel the products you are selling to your customers. As explained before, products or features fueled by data can be personalized recommendations, voice assistants, or fuel consumption optimizers for f1 cars. If your products/features are of this type, you should definitely hire a data PM.
If data do not fuel your product, then you should ask yourself a second question. This question is whether your internal operations are heavily data-driven. Does your business generate a gigantic amount of data that needs to be stored, cleaned, and analyzed? Does some department of your company need accurate forecasts? Does your company face a lot of challenges in terms of logistics? If the answer to these questions is yes, then you have highly data-driven operations. If you have highly data-driven operations, then you should hire a data PM. On the contrary, if you do not have highly data-driven operations, a PM will do the job.

Subscribe to the Castor Blog
About us
We write about all the processes involved when leveraging data assets: from the modern data stack to data teams composition, to data governance. Our blog covers the technical and the less technical aspects of creating tangible value from data.
At Castor, we are building a data documentation tool for the Notion, Figma, Slack generation. We designed our catalog software to be easy to use, delightful and friendly.
Want to check it out? Try CastorDoc for free with a 14 day demo.
You might also like
Get in Touch to Learn More
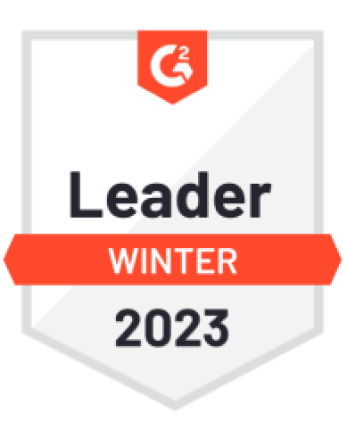
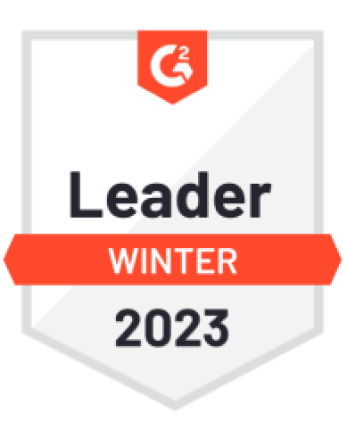

“[I like] The easy to use interface and the speed of finding the relevant assets that you're looking for in your database. I also really enjoy the score given to each table, [which] lets you prioritize the results of your queries by how often certain data is used.” - Michal P., Head of Data