Which type of "Head of Data" are you?
The roles hidden behind the "Head of Data" title
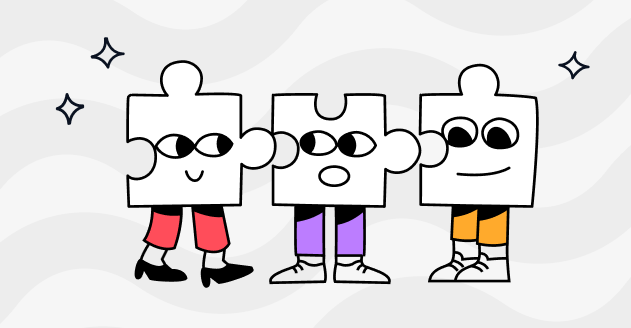
Introduction
In the last few years, we've seen an explosion in the number of Heads of Data. As this role has evolved, so too have the types of people who are stepping into this position. So what kind of person should become a data leader? Well, there is no straightforward answer to that. The job of a Head of Data is not one-size-fits-all, and neither are the people who fill this role.
There is a problem with how this job title is used. Because the term “Head of Data” is used so loosely, you can't really tell what a company is looking for. They may be looking for a Business Intelligence leader or a data engineering leader. Or maybe they want both! Maybe they're just looking for a unicorn. Or maybe they don't know what they want at all. This leads to confusion among employees who don't know where they fit into this model, and also makes it difficult for companies to hire the right people for their needs. This article is here to help get everyone on the same page.
Most of the time, when a company declares that they need a "Head of Data," they can either mean that they need:
- A BI leader or manager: Someone who knows how to make sense of data by bringing clarity to business problems or opportunities
- A Data Engineering leader: Someone who is good at making sure that data is accurate and available according to IT standards
These are two very different roles. I agree that they can sometimes overlap, but they usually require different types of people, wearing different kinds of hats. How can you figure out which type of Head of Data you want to be? The background/skillset needed? We cover it all here.
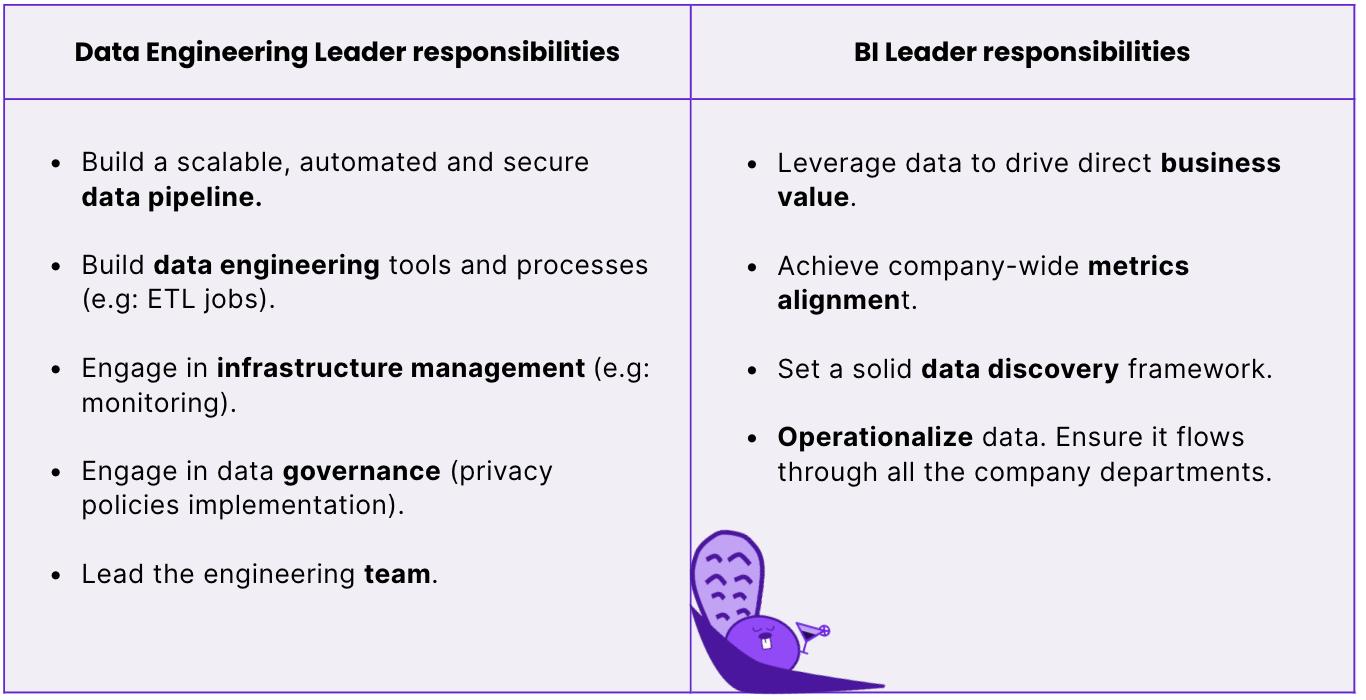
Type 1: The Engineering Leader
Mission
The data engineering leader is the person who makes it possible for data scientists to do their jobs. They work with engineers and data analysts to make sure that the company's data infrastructure is scalable, reliable, secure, and cost-effective.
What do they do?
The engineering leader focuses on building the technical backbone of the data science team and organization. They work behind the scenes to make analytics work possible. They're tasked with creating a data pipeline that's scalable, automated, secure, and auditable from end to end. Their work may involve building new tools or processes for data engineering (e.g., ETL jobs), infrastructure management (e.g., monitoring), and security and governance (e.g., privacy policies). When the organization is mature enough, engineering leaders might engage in Data Science and Machine learning. If you're in this role yourself, you likely wear multiple hats at once: prioritizing projects; leading teams of engineers; communicating with other departments about what they can expect from your department moving forward —all while keeping up with industry trends to keep your organization competitive. We dive deeper into each category.
Data Engineering
A key responsibility of the engineering leader is to supervise and optimize the data integration process. Data integration refers to the activity of combining data from different sources into a single source of truth: the data warehouse. It is the first step in the data engineering lifecycle, and it can be done using ETL tools. Data engineers are often responsible for completing this task, but it may also be completed by a combination of business analysts, data scientists, and software developers. The data engineering is responsible for:
- Ensuring there are sufficient resources to support the pipeline at all times.
- Tracking and monitoring capacity utilization for each component in the pipeline, including storage, compute, network bandwidth, and latency considerations.
- Review performance metrics regularly to identify issues before they become critical problems.
Infrastructure management
As an engineering leader, attending to the details of data infrastructure is no option. This means understanding how your data is stored, how it moves around your system, and how you can get it into the right shape to analyze. Data engineering leaders need to know what tools are available for working with the data. They should also have a working knowledge of how these tools work under the hood so they can make informed decisions on when they should be used, or which features might be worth incorporating into existing tools. Data infrastructure tools have been proliferating in the past few years, and it has become very hard to keep track of the ecosystem. We’ve pulled together a guide for simplifying purposes. Don’t hesitate to check it out.
Security and governance
Security & governance are also the daily bread of a data engineering leader.
- Data governance is a set of processes that ensures that data assets are managed in an efficient manner. In the context of data engineering, this means ensuring that you have a plan for how to handle your data, how to track its quality, and who will use it. Data quality is a measure of the accuracy, relevance, and timeliness of data. It's also a measure of its usefulness in supporting business processes. A good data quality program will ensure that all your organization's information is up-to-date and accurate before being used by any applications or services (i.e., users). This can help reduce errors within your company as well as save time (and money) when you have to fix problems later on down the line.
- Data security & privacy: as the data engineer leader, you're responsible for ensuring that your systems adhere to security standards. This means keeping up with industry-leading tools and protocols to ensure your company's sensitive information is protected from hackers and other malicious actors. Remember: every time you make a change or deploy an update to one of your systems, there's a chance it could compromise the privacy of those using them.
The data engineering leader position reports to the CTO and includes leading a team of data engineers. Data engineers are responsible for storing and processing data, building and maintaining data pipelines, and creating ETL jobs that load data into systems of record (SOR). They also work with data scientists to understand how to scale and optimize the SOR in order to support business needs.
What’s their background?
The engineering leader usually has a background in computer science, software engineering, or equivalent experience. They also have experience leading teams of developers, which can include managing teams remotely or in person.
The data engineering leader is responsible for building out the technology roadmap and ensuring that it aligns with the company’s business strategy. This includes setting up an agile process (like Scrum) to manage releases, working with customers to define requirements, writing technical specs, reviewing code written by other developers, and doing all of this while keeping an eye on costs and maintaining quality control.
Key metrics for a Data Engineering leader
The mission of the data engineering leader is to ultimately provide clean and reliable data to other stakeholders in the company. The key metrics to measure his success are thus mostly related to data quality and include data accuracy, consistency, completeness, and reliability. The data engineering leader is also responsible for good data management within the company, and for automating processes to increase productivity. A natural consequence of automation and good data management is infrastructure cost savings. This is also a nice metric to look at if you’re seeking to evaluate your performance as a data leader. We’ve compiled a small guide explaining how these metrics work and how to measure them.
Type 2: The Business Intelligence Leader
Mission
The BI leader's mission is to use data to drive business outcomes. That can mean anything from helping a company make better decisions, to optimizing revenue and profit, to improving customer experience. The data business leader is a person who might not be very technical but has strong business knowledge. They can understand how to align the technical team with what the business wants and needs.
What do they do?
The BI leader is responsible for the success or failure of their organization's data initiatives, but they don't need to be an expert on how every tool works or be able to explain it in detail. They need to know how things work as a whole and what goals each department has in mind, but not necessarily how those goals will be achieved using specific techniques.
Business Intelligence leaders’ mission is to leverage data to drive business value. In a way, they are more directly in touch with ROI generation. Concretely, they fight for three things: Data discovery, operationalization, and metrics alignment.
Discovery
Data discovery is the process of finding, understanding, and using data to make decisions. To be successful at data-driven decision-making, you need a solid data discovery process in place.
With data discovery as part of your regular business processes (and not just an afterthought), you'll find yourself making better decisions faster than ever before!
Data discovery starts with asking questions about what you want to achieve. In other words, it's not enough to have access to all the information in your organization; it's important to know how that information can be used as well. Data discovery ensures that people from different teams and groups work together on gathering and analyzing data in order for everyone involved to understand what they're working with -- whether they're trying out a new product idea or looking at which advertising campaign has performed best so far this year.
Metrics alignment
The BI leader also enforces company-wide alignment around metrics. Without strong processes around metrics, the latter end up being spread out across spreadsheets, internal dashboards, and data tools. Reporting supposedly simple metrics such as revenue can become very tricky due to multiple definitions and a lack of trust in the data. Fragmented systemleads to team disagreements about definitions, ownership, and accuracy of metrics.
In order to ensure that metrics are being managed consistently across the company, you can do the following:
- Write documentation for any framework or codebase you create. If you already have invested in a data catalog tool, you can document your metrics in the section dedicated to this purpose.
- Ensure that all departments in your organization use a metrics store. This should be a tool where they can document their metrics, and it should support visualization so that users can look at trends over time. You should also enforce good documentation practices to support your knowledge workers' access to data.
Operationalization
If data engineer leaders seek to provide clean data to the rest of the company, data business leaders’ mission is to operationalize this data. Operationalization is an approach consisting of making data accessible to “operational” teams (sales, marketing, ..). We distinguish it from the more classical approach of using data only for reporting and business intelligence. Instead of using data to influence long-term strategy, operational analytics inform strategy for the day-to-day operations of the business. To put it simply, it’s putting the company’s data to work so everyone in your organization can make smarter, faster decisions.
Operationalizing data can be achieved only once data discovery is already part of your regular business processes, and not just a mere afterthought. Operational teams are not always used to working with data. Domain experts will only use data as part of their daily operations, independently from technical teams if you have enforced a strong data discovery process.
Usually, putting data in the hands of other teams requires investing in a rock-solid data documentation tool. This will ensure company data is:
- Easy to search (including by yourself) allowing all departments to access information when they need it.
- Easy to understand: users can quickly understand what exists within each table in their source system(s).
- Easy to update, query and audit.
These three pillars are intertwined. You can’t have data operationalization without data discovery or metrics alignment. BI leaders usually push these three projects in parallel.
What’s their Background?
A BI leader usually has a background in either marketing/sales or product management. They have experience working with customers and understanding what they want to achieve. They understand how to translate that into actionable items for their team. The key is that they have lived in the trenches themselves and know what it takes to make things happen in the real world — not just on paper.
They also have enough technical knowledge to understand what the engineers are doing and why they are doing it. They are able to articulate what they are doing and why it matters. They know to set goals, measure progress and hold people accountable for achieving results.
Key metrics for the Business Intelligence leader
Data business leaders impact variables that are slightly harder to quantify than those of data engineering leaders. You can look at the percentage of documented datasets to evaluate the discovery efforts, but it is harder to measure the alignment around metrics. A nice way to measure data operationalization is to look at the number of problems you allow other teams to solve independently. For example, when the data team is relatively young, it might get a lot of requests from the marketing team about attribution. As data democratization improves, with operational teams accessing the data easily, the need to rely on the data team to solve attribution issues decreases. The marketing team becomes more independent in solving this kind of problem, ultimately driving the number of attribution-related requests to zero. A good measure of how well you’re operationalizing your data is to look at the reduction in the number of requests in various categories. This metric measures exactly the extent to which the business is enabled to use data. And the more you can tick problems off the list, the more your data is operationalized.
Conclusion
It's not that there isn't a role called "Head of Data," it's just that it doesn't actually exist in most companies.
Instead, what happens is that when a company decides they need someone to take charge of their data, they usually reach out to two very different roles: BI leaders and data engineering leaders. The problem is that these roles have nothing to do with one another! Defining specifically the kind of profile you are looking for is hard for a company. Companies looking for a Head of Data are often just looking for someone who can’t help them make sense of all this data mess. But there is more granularity to this, and everyone would gain from companies clarifying which role they have in mind exactly in the hiring process.
Subscribe to the Castor Blog
About us
We write about all the processes involved when leveraging data assets: from the modern data stack to data teams composition, to data governance. Our blog covers the technical and the less technical aspects of creating tangible value from data.
At Castor, we are building a data documentation tool for the Notion, Figma, Slack generation. We designed our catalog software to be easy to use, delightful and friendly.
Want to check it out? Get a free demo.
You might also like
Get in Touch to Learn More
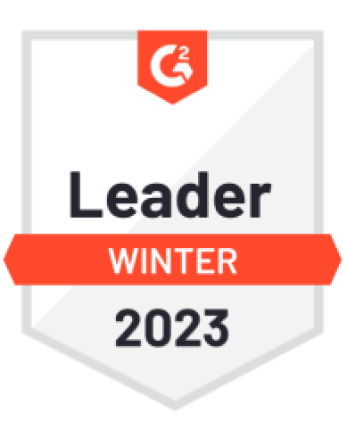
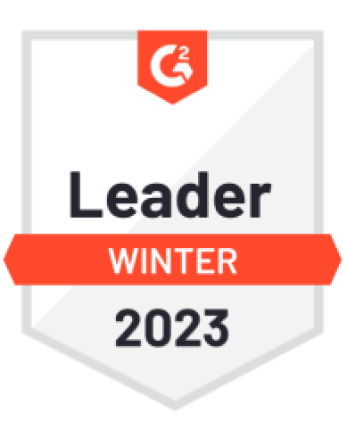

“[I like] The easy to use interface and the speed of finding the relevant assets that you're looking for in your database. I also really enjoy the score given to each table, [which] lets you prioritize the results of your queries by how often certain data is used.” - Michal P., Head of Data