Where are you in your data journey?
and what are the next steps for your data team
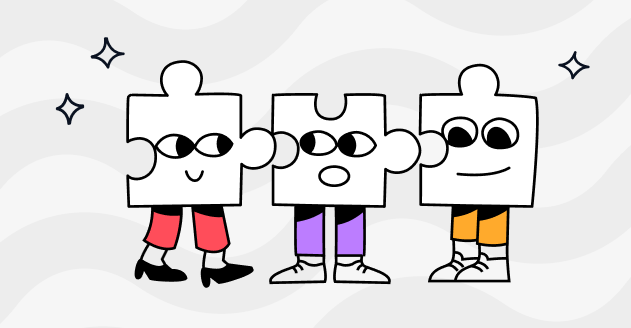
The next steps for your data team
Today, every company wants to "leverage data". You hear it everywhere, everyday, you know you have to do it. It sounds great on paper, you're going to "create business value rooted in strong and meaningful data insights". But the truth is, the journey towards truly leveraging data is long, rocky, and not so straightforward. And to identify the next step in your data journey, you need to start by identifying where you are. That's what we propose today: a simplified data maturity assessment. Why?
- It will help you build and structure a strong data practice in your company. The data maturity of your organisation will affect the size, the composition, the reporting system and the structure your data team. If you want to know more about data team structure, we talk about it here. But read this first.
- It will help you define your data priorities, and which projects you will take on next. If you do things in the right order, leveraging data will be a breeze. If you don't, it will become a real nightmare. There is a right order? What? Yes. We explain it here.
Beware, company size, and data maturity are two different things. Your organization can be large but immature on a data level.
Understanding your Past, knowing your Present, predicting your Future
Data maturity is the journey towards seeing tangible value from your data assets. We propose a simple framework of data maturity assessment, in which you measure your ability to understand your past, know your present and predict your future. What do I mean by this?
Well, in most companies each department has its own set of KPIs that support the execution of the corporate strategy. It’s not enough just to define them, they must also be clearly tracked, and you must also have the ability to predict future outcomes against these KPIs. This ability rests on a clear knowledge of your present, which, in turn, builds on a strong understanding of the past. Do this, and you have found a simple way to assess your data maturity. For example, If you're unable to identify the revenue drivers for your company (your past), it means you need to work on your data maturity by bringing visibility to your business before you seek to predict future outcomes. We don't recommend skipping steps. It's like Maslow's hierarchy of needs, but for data.
.png)
Let's look at a couple of practical examples:
Marketing ROI. Define your ROI, across multiple channels, by using an identified attribution model. Then understand its evolution in the previous 12 months, and especially its drivers (identify performing channels, time of the year, product, ....). Then track on a daily/weekly/monthly basis its evolution thanks to a reporting tool you trust (present). Forecast your marketing budget based on these predictive models (future).
Customer Satisfaction. Define your customer satisfaction measure. Is it NPS, CSAT? Everyone in your company should share a common understanding of how it is computed. As with our previous example, compute its evolution in the previous 12 months, find its drivers (past). Then track daily the satisfaction of your customers with trusted dashboards. Identify action to take from today to increase it. Your understanding of the past and the present state of customers satisfaction will allow to predict churn efficiently (future)
Understanding your past and present is commonly referred to as performing descriptive analytics. Descriptive analytics help an organization understand its performance by providing context to help key stakeholders interpret information. This context is usually in the form of data visualization, including graphs, dashboards, reports and charts. When you’re analysing data to forecast the future, you’re engaging in predictive analytics. The idea with predictive analytics is to take historical data, feed it into a machine learning model that considers key patterns. Apply this model to current data, and hope that it will forecast the future. We’ll use the terms of descriptive and predictive analytics throughout the article to refer to understanding past, present or predicting the future.
Understanding your past
The first step of a successful data journey is to understand where you come from, and how you've arrived here. But where should you start to understand the key drivers of your growth, customer churn, etc?
- Have clear business definitions, and ensure everyone in your company is aligned with respect to these definitions. Defining your KPIs' is not a Friday afternoon activity, when you're done with the other stuff, it's a Monday morning priority. Why? When you ask an employee to calculate the Monthly Recurring Revenue (MRR), he will calculate his interpretation of the MRR, which is usually different than what you have in mind. And it's like this for every business term. If you don't define your KPIs' first, you end up with as many definitions as you have employees. And that's the beginning of chaos.
- Get a data warehouse. A data warehouse allows you to consolidate data from different sources, and have all your data in the same place. It also converts this data into the consistent formats required by your analytics platforms. Additionally, it ensures that the data produced by different business divisions is at the same quality and standard – allowing a more efficient feed for analytics.
Knowing your present
A sign that you have a good knowledge of your present is that you can produce reliable dashboards which are consistent across departments, and develop strong data governance policy. Concretely, this entails going though the following steps:
- Once your data is stored in a single place, you can start data modelling. At a high level, data modeling is the process of visualizing and representing data for storage in a data warehouse. A data model is a conceptual representation of the data, the relationships between the tables, and the rules. The simplest data model is nothing more than entities and relationships. Why is data modelling so important? By imposing a clear structure on data, it allows you to understand exactly which data you have and how it relates to your business.
- After completing these three (substantial, I give it to you) projects, you will be able to truly understand your past. That is, the exact drivers of your growth, customer churn, and so on. If you've done things in the right order, dashboard creation will be extremely simple. You just need to choose which pre-defined KPI's to track, select your data sources, and build your dashboards with simple graphs.
- Only then should you focus on data governance. Data governance is about establishing practices to help you get clear answers to the following important questions:
- What data do we have?
- Where did this data come from?
- How is this data used?
- Who is responsible for this data?
- Can we trust this data?
Data governance defines who can take what action, upon what data, in what situations, using what methods. You can't establish the foundation for effective data governance if you haven't cleared the previous steps. In fact, it's impossible to assign roles and decide who has access to which datasets if you can't locate the datasets (2) and haven't clearly established how they relate to your business (3).
Predicting your future
Once you have an effective data governance, it means your organization is fairly mature. You can confidently turn towards predicting future outcomes. Predictive analytics focuses specifically on learning past behaviours to predict future behaviours. For example, financial institutions use predictive models to assign credit scores. These scores take data on past financial interactions and use them to predict future financial behaviour. This is why prediction should be the last step of your data journey. In our example, it would unthinkable to seek to predict financial behaviour if you can't locate or exploit the data on past financial transactions.
To sum up, your data journey should follow the ensuing milestones: Define - Collect - Arrange - Govern - Predict. These steps should be taken with the aim of understanding your past, knowing your present and predicting your future.
About us
We write about all the processes involved when leveraging data assets: from the modern data stack to data teams composition, to data governance. Our blog covers the technical and the less technical aspects of creating tangible value from data.
At Castor, we are building a data documentation tool for the Notion, Figma, Slack generation. We designed our catalog software to be easy to use, delightful and friendly.
Want to check it out? Give the CastorDoc software a test drive with our free 14-day demo.
Subscribe to the Castor Blog
You might also like
Get in Touch to Learn More
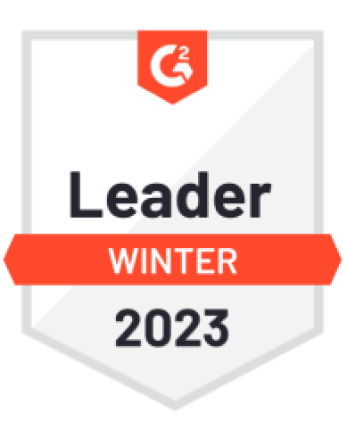
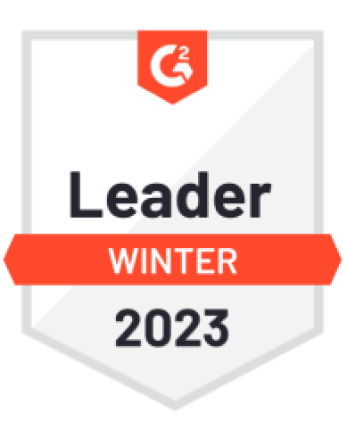

“[I like] The easy to use interface and the speed of finding the relevant assets that you're looking for in your database. I also really enjoy the score given to each table, [which] lets you prioritize the results of your queries by how often certain data is used.” - Michal P., Head of Data