How to Avoid Gaps in Data in Databricks?
Data is a crucial asset for any organization, and it plays a vital role in decision-making processes. In the context of Databricks, complete and accurate data is of utmost importance for achieving optimal performance and extracting meaningful insights. Failure to ensure data completeness can lead to severe consequences and hinder the overall efficiency of the data management system. In this article, we will explore the significance of complete data in Databricks, the potential causes of data gaps, effective data management strategies, utilizing Databricks features to prevent gaps, and establishing a robust data recovery plan.
Understanding the Importance of Complete Data in Databricks
Complete data refers to having all the necessary information required for a given analysis task. In Databricks, complete data is the foundation upon which accurate and reliable insights are derived. It enables businesses to make informed decisions, identify trends, detect anomalies, and optimize processes. Without complete data, organizations risk making faulty conclusions and compromising the overall integrity of their analyses. Therefore, ensuring data completeness is a critical aspect of data management in Databricks.
The Role of Data in Databricks
Data serves as the lifeblood of Databricks, fueling the power of analytics and machine learning models. It provides the raw material for training algorithms, validating hypotheses, and solving complex problems. By processing vast amounts of data, Databricks unlocks crucial insights and empowers companies to gain a competitive edge. However, data gaps can severely impact this process, leading to inaccurate results, flawed modeling, and ultimately, unreliable business decisions.
Consequences of Data Gaps in Databricks
The consequences of data gaps in Databricks can be far-reaching. Firstly, incomplete data can skew analysis results, resulting in misleading patterns or incorrect predictions. This can lead to faulty decision-making, operational inefficiencies, and missed opportunities. Furthermore, incomplete data can hinder the training of machine learning models, compromising their performance and undermining their ability to provide accurate predictions. Moreover, incomplete data can erode customer trust, as it may lead to unresponsive or inaccurate recommendations.
One example of the consequences of data gaps in Databricks can be seen in the retail industry. Imagine a retail company that relies on data analysis to make inventory decisions. If the data used for analysis is incomplete, the company may not have a clear understanding of customer demand for certain products. This could result in overstocking items that are not popular, leading to wasted resources and lost revenue. On the other hand, if the data gaps lead to underestimating demand, the company may not have enough stock to meet customer needs, resulting in dissatisfied customers and missed sales opportunities.
Another industry where data completeness is crucial is healthcare. In Databricks, healthcare organizations rely on data analysis to improve patient outcomes and optimize resource allocation. However, if the data used for analysis is incomplete, it can lead to inaccurate predictions and suboptimal decision-making. For example, if a hospital is analyzing patient data to identify high-risk individuals who may require additional care, incomplete data could result in missing out on crucial information. This could lead to inadequate treatment plans and potentially negative health outcomes for patients.
Identifying Potential Causes of Data Gaps
Before addressing the issue of data gaps in Databricks, it is essential to identify the potential causes that might be responsible for this problem. By understanding the root causes, organizations can take proactive measures to prevent data gaps from occurring. Let's explore some common causes:
Common Data Collection Issues
Data collection is the initial step in the data management process, and any issues during this phase can lead to data gaps. Common issues include incomplete data entry, human errors during data collection, missing timestamps or metadata, and inconsistent data formatting. For example, imagine a scenario where a sales representative forgets to input a crucial piece of customer information into the system. This missing data can create a gap in the overall dataset, making it challenging to analyze customer behavior accurately.
To address these issues, organizations need to establish robust data collection procedures. This can involve implementing automated data entry systems that minimize human errors, conducting regular data quality checks to identify missing or inconsistent data, and providing training to employees to ensure they understand the importance of accurate data collection.
Technical Glitches and System Errors
Technical glitches and system errors can disrupt the data flow into Databricks, resulting in data gaps. This can occur due to network failures, server outages, or incompatible data formats. For instance, imagine a situation where a sudden power outage occurs, causing the server hosting the data collection system to go offline. During this downtime, data might not be captured, leading to a gap in the dataset.
To mitigate these issues, organizations must monitor the data pipeline closely. Implementing real-time monitoring systems can help identify any disruptions in the data flow and enable prompt action to rectify the problem. Additionally, having backup systems in place, such as redundant servers or alternative data collection methods, can ensure continuous data capture even in the event of technical glitches or system errors.
By addressing these common causes of data gaps, organizations can enhance the reliability and completeness of their datasets. This, in turn, enables more accurate analysis and decision-making, leading to improved business outcomes.
Implementing Effective Data Management Strategies
To avoid data gaps in Databricks, organizations need to implement effective data management strategies. These strategies will ensure that data is collected, stored, and processed in a way that minimizes gaps and ensures data completeness. Here are two key strategies:
Regular Data Auditing
Regular data auditing is a proactive approach to identify and rectify data gaps. By periodically reviewing the data quality and completeness, organizations can spot any discrepancies and take corrective actions. Data auditing also includes verifying data sources, validating data integrity, and ensuring proper data documentation.
During the data auditing process, organizations can leverage advanced tools and technologies to automate the identification of data gaps. These tools can analyze large volumes of data, comparing it against predefined rules and patterns to identify any inconsistencies. By automating this process, organizations can save valuable time and resources, ensuring that data gaps are promptly addressed.
Data Validation Techniques
Data validation is the process of checking the accuracy, consistency, and integrity of data. This involves performing various checks, such as data type validation, range checks, and referential integrity checks. By implementing data validation techniques, organizations can detect and handle data gaps more effectively, ensuring the reliability of their analyses.
One important aspect of data validation is establishing clear data quality metrics and thresholds. Organizations can define specific criteria that data must meet to be considered valid. For example, they can set thresholds for acceptable data ranges or establish rules for data consistency across different sources. By setting these metrics, organizations can easily identify and address any data gaps that fall outside the defined criteria.
Furthermore, organizations can leverage machine learning algorithms and artificial intelligence to enhance their data validation techniques. These advanced technologies can analyze historical data patterns and identify potential data gaps or anomalies. By incorporating machine learning into their data management strategies, organizations can proactively detect and prevent data gaps, ensuring the accuracy and reliability of their data.
Utilizing Databricks Features for Gap Prevention
Databricks offers built-in features and tools that can significantly contribute to preventing data gaps. Leveraging these features can enhance the data management process and minimize the occurrence of gaps. Let's explore two notable features:
Leveraging Databricks' Built-in Tools
Databricks provides built-in tools for data quality monitoring, data validation, and data pipeline management. These tools enable organizations to establish robust data quality controls, identify anomalies, and ensure the integrity of data throughout the entire data lifecycle. By utilizing these tools effectively, businesses can proactively prevent data gaps from occurring.
Customizing Databricks for Better Data Management
Databricks allows organizations to customize their data management workflows to suit their specific requirements. By tailoring Databricks to automate data collection, implement data validation processes, and establish data quality checks, organizations can significantly minimize the chances of data gaps. This customization empowers businesses to maintain control over their data management processes and ensure data completeness.
Establishing a Robust Data Recovery Plan
Despite all preventive measures, data gaps can still occur in Databricks. Therefore, it is crucial to establish a robust data recovery plan to minimize the impact of data gaps and recover swiftly. Here are a few essential steps to create a data recovery plan:
Importance of Backup and Recovery
An effective data recovery plan involves regular backups of critical data and the ability to restore data quickly. By investing in reliable backup mechanisms and establishing recovery procedures, organizations can minimize data loss and minimize downtime caused by data gaps.
Steps to Create a Data Recovery Plan in Databricks
Creating a data recovery plan involves identifying critical data sources, defining recovery objectives and timelines, establishing backup schedules, and practicing simulated data recovery scenarios. By following these steps, organizations can ensure that in the event of data gaps, recovery is prompt and minimal disruption is caused.
In conclusion, data completeness is a vital factor in the success of any data management system, particularly in Databricks. By understanding the importance of complete data and implementing effective strategies, organizations can minimize data gaps, maximize data integrity, and make accurate and informed decisions. Proactive measures, such as regular data audits, data validation techniques, and leveraging Databricks features, coupled with a robust data recovery plan, will ensure that data gaps are avoided or swiftly rectified, allowing companies to unlock the true power of their data in Databricks.
Get in Touch to Learn More
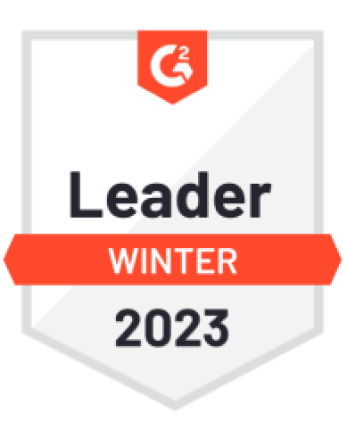
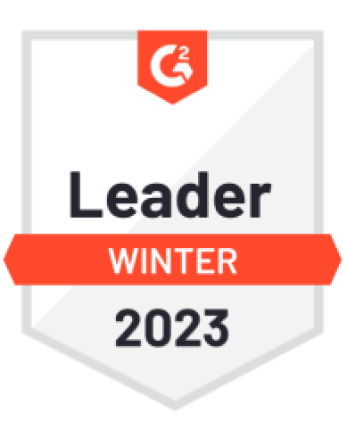

“[I like] The easy to use interface and the speed of finding the relevant assets that you're looking for in your database. I also really enjoy the score given to each table, [which] lets you prioritize the results of your queries by how often certain data is used.” - Michal P., Head of Data