10 Powerful Dataset Examples to Inspire Your Next Data-Driven Project
Explore 10 dataset examples that will fuel your next data-driven project.
Datasets are essential for the success of data-driven projects, as they provide the foundation for analysis, insights, and decision-making. However, not all datasets are created equal. In this article, we will explore the characteristics of a powerful dataset, their importance in different industries, and the value of both open source and proprietary datasets. By the end, you'll have a clearer understanding of how datasets can inspire and drive your next data-driven project.
Understanding the Importance of Datasets in Data-Driven Projects
Datasets are the raw materials that fuel data-driven projects. They are collections of data points that are organized and structured to be analyzed, interpreted, and used to make informed decisions. Without high-quality datasets, data-driven projects lose their power and effectiveness.
When it comes to datasets, quality is key. High-quality datasets are accurate, reliable, and relevant to the problem at hand. They are free from errors, inconsistencies, and biases that could lead to incorrect conclusions. Data scientists and analysts spend a significant amount of time cleaning and preparing datasets to ensure their quality, as the success of a data-driven project often hinges on the quality of the datasets used.
The Role of Datasets in Data Analysis
Data analysis is the process of inspecting, cleaning, transforming, and modeling datasets to discover meaningful patterns, draw conclusions, and support decision-making. Without datasets, data analysis becomes impossible. Datasets provide the necessary context and information for analysts to extract insights and make data-driven recommendations.
Furthermore, datasets play a crucial role in machine learning and artificial intelligence applications. These technologies rely heavily on large, diverse datasets to train models and make accurate predictions. The quality and quantity of the training data directly impact the performance and reliability of machine learning algorithms, highlighting the importance of datasets in cutting-edge data-driven technologies.
How Datasets Drive Decision-Making
In data-driven projects, decisions are made based on evidence, not intuition. Datasets provide the evidence needed to support decision-making. They help stakeholders understand trends, identify opportunities, and mitigate risks. By analyzing datasets, decision-makers can make well-informed choices that drive business growth and success.
Moreover, datasets enable organizations to measure the effectiveness of their strategies and initiatives. By tracking key performance indicators and analyzing relevant datasets, businesses can assess the impact of their decisions and adjust their course of action accordingly. This data-driven approach to decision-making fosters a culture of continuous improvement and innovation within organizations, driving them towards long-term success.
Characteristics of a Powerful Dataset
Not all datasets are created equal. To be powerful and impactful, datasets must possess certain characteristics.
When considering the size and complexity of a dataset, it's important to understand how these factors can significantly impact its usefulness. Large datasets with a wide range of variables provide more opportunities for in-depth analysis and the discovery of hidden patterns or correlations. The complexity of a dataset, which can stem from the inclusion of multiple dimensions and intricate relationships, allows for a more thorough and nuanced examination of the data, leading to richer insights and more informed decision-making.
Size and Complexity in Datasets
The size and complexity of a dataset can significantly impact its usefulness. Large datasets with a wide range of variables provide more opportunities for analysis and deeper insights. Complex datasets that incorporate multiple dimensions and relationships allow for more comprehensive and nuanced analysis.
Moreover, the quality and accuracy of data within a dataset are paramount for ensuring the reliability of any analysis conducted. Data integrity is crucial as it underpins the trustworthiness of the insights derived from the dataset. Inaccurate or incomplete data can not only skew the results but also lead to misguided conclusions and ineffective decision-making processes. Therefore, meticulous attention to data quality is essential for harnessing the full potential of a dataset.
Quality and Accuracy of Data
The quality and accuracy of data within a dataset are paramount. Data integrity ensures the reliability and trustworthiness of the analysis and insights derived from the dataset. Inaccurate or incomplete data can lead to misleading conclusions and ineffective decision-making.
Furthermore, a powerful dataset is one that contains not only relevant but also timely data. Relevance is key as it ensures that the information within the dataset aligns with the specific goals and objectives of the data-driven project at hand. Timeliness, on the other hand, guarantees that the data is current and reflective of the rapidly evolving business landscape. Without the combination of relevance and timeliness, datasets run the risk of offering outdated or extraneous insights that may hinder rather than enhance decision-making processes.
Relevance and Timeliness of Data
A powerful dataset is one that contains relevant and timely data. Relevance ensures that the information within the dataset aligns with the goals and objectives of the data-driven project. Timeliness ensures that the data is up to date and reflective of the current business environment. Without relevance and timeliness, datasets may provide outdated or irrelevant insights.
Datasets in Different Industries
Datasets have applications across various industries. Let's explore how datasets are utilized in healthcare, finance, and retail.
Datasets in Healthcare
In the healthcare industry, datasets are essential for research, disease surveillance, and improving patient care. From electronic health records to genomic data, healthcare datasets provide invaluable insights into disease trends, treatment effectiveness, and patient outcomes. These datasets help healthcare professionals make evidence-based decisions and improve the quality of care.
Moreover, healthcare datasets play a crucial role in advancing medical research and innovation. By analyzing large datasets, researchers can identify new treatment options, predict disease outbreaks, and develop personalized medicine approaches. The integration of datasets from various sources, such as clinical trials and wearable devices, enables a comprehensive understanding of patient health and contributes to the development of cutting-edge medical technologies.
Datasets in Finance
The finance industry heavily relies on datasets for risk management, investment analysis, and financial modeling. Datasets containing market data, economic indicators, and historical trends provide valuable information for making informed financial decisions. In finance, datasets are instrumental in assessing market conditions, predicting future trends, and managing investment portfolios.
Furthermore, the use of machine learning algorithms and artificial intelligence in analyzing financial datasets has revolutionized the industry. These technologies can process vast amounts of data in real-time, identify patterns, and make predictions with a high degree of accuracy. By leveraging advanced analytics on financial datasets, institutions can optimize trading strategies, detect fraudulent activities, and enhance regulatory compliance.
Datasets in Retail
Retail datasets offer valuable insights into customer behavior, market trends, and inventory management. With the rise of e-commerce and online shopping, retailers can collect vast amounts of data, including purchase history, browsing patterns, and customer feedback. Analyzing these datasets allows retailers to personalize marketing strategies, optimize pricing, and enhance the overall customer experience.
In addition, retail datasets are instrumental in supply chain management and demand forecasting. By analyzing data on sales trends, seasonal variations, and customer preferences, retailers can streamline inventory levels, reduce stockouts, and improve operational efficiency. The integration of data from point-of-sale systems, customer loyalty programs, and social media platforms enables retailers to gain a comprehensive understanding of consumer behavior and tailor their business strategies accordingly.
Open Source Datasets and Their Potential
Open source datasets provide a wealth of opportunities for data-driven projects. Let's explore the benefits of utilizing open source datasets and how to find and leverage them.
Benefits of Using Open Source Datasets
Open source datasets offer numerous advantages. Firstly, they are freely available, which reduces costs and increases accessibility. Secondly, open source datasets are often community-led, meaning they undergo continuous improvement and refinement by a collective of contributors. Lastly, they promote collaboration and knowledge sharing, allowing data scientists and analysts to build upon existing work and drive innovation.
Finding and Utilizing Open Source Datasets
When looking for open source datasets, various platforms and repositories can be utilized. Websites like Kaggle, Data.gov, and GitHub offer a vast collection of open source datasets across different domains. Additionally, online communities and forums dedicated to data science and machine learning often share valuable datasets and insights. Utilizing open source datasets involves proper attribution and adhering to any licensing requirements.
Proprietary Datasets and Their Value
While open source datasets offer numerous opportunities, proprietary datasets also have their advantages. Let's explore the benefits of proprietary datasets and how organizations can create and maintain their own.
Advantages of Proprietary Datasets
Proprietary datasets provide organizations with a competitive edge. They offer unique and proprietary data that cannot be easily replicated or accessed by competitors. With proprietary datasets, organizations have more control over data quality, ensuring that it meets their specific requirements. Additionally, proprietary datasets can be tailored to address specific business needs, enabling organizations to derive highly targeted insights.
Creating and Maintaining Proprietary Datasets
To create proprietary datasets, organizations need to collect and curate data from various sources. This may involve capturing data from internal systems, partnering with external vendors, or leveraging third-party data providers. Once acquired, organizations must ensure data quality, perform data cleaning and transformation, and establish robust data governance practices to maintain the integrity of the dataset over time.
Conclusion
Data-driven projects rely on powerful datasets to fuel their insights and decision-making. Understanding the characteristics of a powerful dataset, exploring their importance in various industries, and considering the benefits of both open source and proprietary datasets equip organizations with the tools they need to drive successful data-driven projects. By harnessing the potential of datasets, organizations can unlock a world of possibilities and inspire innovation in their future endeavors.
Ready to transform your data-driven projects with the power of CastorDoc? As the most reliable AI Analytics Agent, CastorDoc empowers your teams to tackle strategic challenges with confidence. Experience the freedom of self-service analytics, elevate data literacy, and maximize your data stack's ROI. Give your business users the autonomy and trust they need to make informed decisions and lighten the load on your data teams. Try CastorDoc today and unlock the full potential of your data.
You might also like
Get in Touch to Learn More
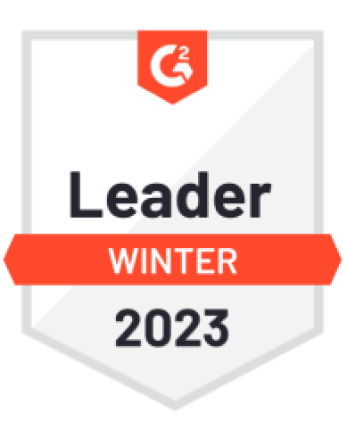
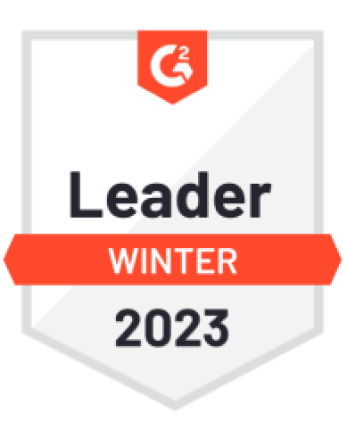

“[I like] The easy to use interface and the speed of finding the relevant assets that you're looking for in your database. I also really enjoy the score given to each table, [which] lets you prioritize the results of your queries by how often certain data is used.” - Michal P., Head of Data