Automated Data Analysis: Streamlining Processes for Efficiency
Discover how automated data analysis can revolutionize your workflow and streamline processes for optimum efficiency.
Automated data analysis has revolutionized the way organizations analyze and interpret their data. By leveraging advanced algorithms and machine learning technologies, businesses can unlock valuable insights and streamline their processes for greater efficiency. In this article, we will explore the concept and importance of automated data analysis, examine its key components, discuss its role in process optimization, delve into the technology behind it, and highlight challenges and solutions in implementing it. Furthermore, we will take a glimpse into the future of automated data analysis and how it has the potential to shape the business landscape.
Understanding Automated Data Analysis
Automated data analysis refers to the use of software tools and algorithms to process large volumes of data and extract meaningful insights. In today's data-driven world, businesses encounter an overwhelming amount of information that can be challenging to navigate and comprehend. Automated data analysis provides a solution by employing computational methods to analyze data sets quickly and accurately, eliminating the need for manual analysis and reducing the risk of human errors.
The Concept and Importance of Automated Data Analysis
Automated data analysis is the backbone of data-driven decision-making. It enables organizations to uncover patterns, trends, and correlations that may otherwise go unnoticed. By identifying these insights, businesses can make data-informed decisions, optimize their operations, and gain a competitive advantage in the market. Whether it's identifying customer preferences, predicting market trends, or optimizing supply chain management, automated data analysis plays a pivotal role in driving success.
Key Components of Automated Data Analysis
Automated data analysis comprises several key components that work together to process and interpret data. One such component is data preprocessing, which involves cleaning, transforming, and organizing raw data to ensure its quality and consistency. Another critical component is algorithm selection, where the appropriate analytical algorithms are chosen based on the specific task at hand. Moreover, automated data analysis relies on robust statistical techniques, data visualization tools, and machine learning algorithms to derive actionable insights from complex datasets.
One important aspect of automated data analysis is data preprocessing. This step involves cleaning the data by removing any inconsistencies, errors, or duplicates. It also includes transforming the data into a format that is suitable for analysis. For example, if the data is in a raw text format, preprocessing may involve converting it into numerical values or categorical variables. Data preprocessing is crucial because the quality of the input data directly affects the accuracy and reliability of the analysis results.
Another key component of automated data analysis is algorithm selection. Different analytical algorithms are designed to solve specific types of problems, such as classification, regression, or clustering. The choice of algorithm depends on the nature of the data and the objectives of the analysis. For instance, if the goal is to predict customer churn, a classification algorithm like logistic regression or decision trees may be appropriate. On the other hand, if the goal is to segment customers into different groups based on their purchasing behavior, a clustering algorithm like k-means or hierarchical clustering may be more suitable.
The Role of Automated Data Analysis in Streamlining Processes
Enhancing Efficiency with Automated Data Analysis
Efficiency is paramount in today's fast-paced business environment. Automated data analysis streamlines processes and minimizes manual work, allowing organizations to save time and resources. By automating repetitive and labor-intensive tasks, such as data cleaning and transformation, businesses can allocate their resources to more strategic initiatives, leading to improved productivity and overall efficiency.
Automated data analysis tools utilize algorithms and machine learning techniques to process large datasets quickly and accurately. These tools can identify patterns, trends, and outliers that may not be easily detectable through manual analysis. By harnessing the power of automation, organizations can make data-driven decisions with confidence, driving innovation and staying ahead of the competition.
The Impact of Automated Data Analysis on Business Processes
Automated data analysis has a profound impact on various business processes. From sales and marketing to finance and operations, every department can benefit from the insights generated by automated analysis. For instance, predictive analytics can help sales teams improve forecasting accuracy and target high-value customers. Similarly, operations can leverage automation to optimize inventory levels and reduce costs. By integrating automated data analysis into their processes, organizations can achieve better performance and drive continuous improvement initiatives.
Furthermore, automated data analysis enhances data security and compliance efforts within organizations. By automating data processing and analysis, businesses can ensure that sensitive information is handled securely and in accordance with regulatory requirements. This not only reduces the risk of data breaches but also enhances trust with customers and stakeholders, ultimately safeguarding the organization's reputation.
The Technology Behind Automated Data Analysis
The Evolution of Data Analysis Automation
Automated data analysis has come a long way since its inception. Initially, businesses relied on manual data analysis methods, which were time-consuming and prone to human error. However, with advances in technology and the advent of machine learning algorithms, the landscape of data analysis has transformed. Today, organizations have access to sophisticated tools and techniques that can handle massive datasets and uncover insights at unprecedented speeds.
One key aspect of the evolution of data analysis automation is the integration of natural language processing (NLP) capabilities. NLP allows systems to interpret and generate human language, enabling them to analyze unstructured data sources such as text documents, social media posts, and customer reviews. By incorporating NLP into automated data analysis processes, organizations can gain valuable insights from a wider range of data sources, leading to more informed decision-making.
Modern Tools and Techniques in Automated Data Analysis
The technology stack behind automated data analysis continues to evolve rapidly. Modern tools and techniques, such as artificial intelligence and cloud computing, have revolutionized the field. Machine learning algorithms, such as decision trees, neural networks, and random forests, can detect and predict patterns in data, making accurate predictions and recommendations. Cloud computing allows businesses to leverage vast computational resources for handling and analyzing massive datasets, providing scalability and agility.
Another cutting-edge technology that is shaping the future of automated data analysis is edge computing. Edge computing involves processing data closer to the source of data generation, reducing latency and enabling real-time analysis. By deploying edge computing solutions, organizations can analyze data streams from IoT devices, sensors, and other sources in real time, allowing for immediate insights and actions to be taken. This distributed computing approach complements traditional cloud-based data analysis methods, offering new opportunities for organizations to optimize their data processing workflows.
Challenges and Solutions in Implementing Automated Data Analysis
Common Obstacles in Adopting Automated Data Analysis
Despite the numerous benefits offered by automated data analysis, implementing it comes with its share of challenges. One common obstacle is the lack of data quality and availability. Poor data quality can lead to inaccurate analysis results and unreliable insights. This issue can stem from various sources such as data entry errors, outdated information, or inconsistent data formats. Addressing data quality concerns requires implementing data cleansing techniques, establishing data validation processes, and ensuring data is collected from reliable sources. Additionally, businesses may encounter resistance from employees who fear job displacement due to automation. It is essential for organizations to communicate transparently with their workforce, emphasizing how automated data analysis can enhance their roles by allowing them to focus on more strategic tasks that require human creativity and critical thinking.
Strategies for Successful Implementation of Automated Data Analysis
To ensure successful implementation of automated data analysis, organizations should adopt a strategic approach. It starts with clearly defining the business objectives and identifying the key metrics to measure success. This step is crucial in aligning automated data analysis efforts with the overall goals of the organization, ensuring that the insights generated are actionable and contribute to business growth. Next, organizations must establish a data infrastructure that supports automated analysis, including data storage, integration, and security. Investing in scalable and secure data storage solutions, implementing efficient data integration processes, and prioritizing data security measures are essential components of building a robust data infrastructure for automated analysis.
Training the workforce to become data literate and capable of leveraging automated analysis tools is equally crucial. Employees need to develop data literacy skills to interpret analysis results accurately and make informed decisions based on data-driven insights. Providing comprehensive training programs, workshops, and resources on data analysis tools and techniques can empower employees to embrace automation and leverage it effectively in their roles. Lastly, organizations should continuously monitor and evaluate the effectiveness of their automated data analysis processes, making adjustments as necessary to drive continuous improvement. Regular performance reviews, feedback mechanisms, and benchmarking against industry best practices can help organizations optimize their automated data analysis workflows and stay ahead of the competition.
The Future of Automated Data Analysis
Emerging Trends in Automated Data Analysis
The future of automated data analysis is promising, with several emerging trends on the horizon. One such trend is the integration of artificial intelligence and machine learning into analytical tools, enabling autonomous decision-making and real-time analytics. Additionally, automated data analysis is expanding its reach beyond traditional industries, including healthcare, retail, and transportation, paving the way for new applications and use cases. The advent of big data analytics, natural language processing, and edge computing further fuels the advancement of automated data analysis, unlocking new possibilities for organizations seeking to harness the power of their data.
The Potential Impact of Future Developments in Automated Data Analysis
The rapid pace of technological advancements indicates a highly transformative future for automated data analysis. As organizations embrace cutting-edge technologies, such as quantum computing and blockchain, the capabilities of automated data analysis are expected to reach new heights. These developments have the potential to revolutionize industries, improve decision-making processes, and drive innovation at unprecedented levels.
In conclusion, automated data analysis is a powerful tool that can streamline processes and enhance efficiency in organizations. By understanding the concept and importance of automated data analysis, leveraging its key components, and addressing challenges in its implementation, businesses can unlock valuable insights and gain a competitive edge. As technology continues to evolve, the future of automated data analysis holds immense promise, with emerging trends and developments paving the way for a data-driven future.
Ready to harness the transformative power of automated data analysis and propel your business into the future? CastorDoc is here to elevate your organization's efficiency and decision-making capabilities. With our AI Agent for Analytics, you can empower your teams to obtain trustworthy, instantaneous data answers, enabling self-service analytics and maximizing your data stack's ROI. Experience the autonomy, trust, and reduced dependency on data teams that CastorDoc provides. Try CastorDoc today and unlock the full potential of your data.
You might also like
Get in Touch to Learn More
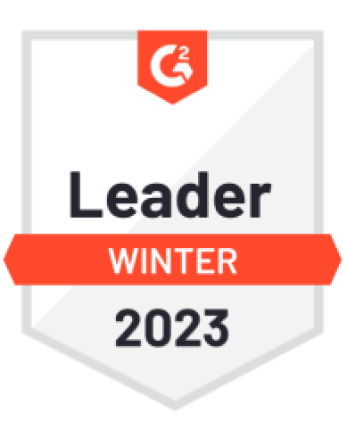
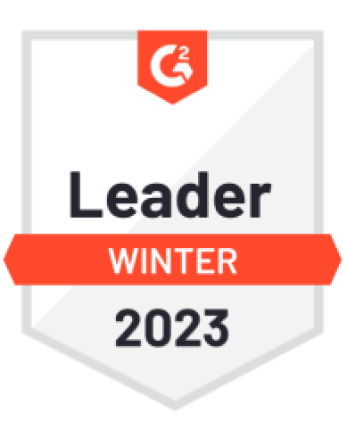

“[I like] The easy to use interface and the speed of finding the relevant assets that you're looking for in your database. I also really enjoy the score given to each table, [which] lets you prioritize the results of your queries by how often certain data is used.” - Michal P., Head of Data