Embedded Analytics Software: Integrating Insights into Applications
Discover how embedded analytics software can seamlessly integrate data insights into your applications, enhancing user experience and driving informed decision-making.
Embedded analytics software has emerged as a powerful tool for businesses looking to leverage data-driven insights directly within their applications. By seamlessly integrating analytics capabilities into their software, companies can empower users to make informed decisions and gain valuable insights in real time. In this article, we will explore the concept of embedded analytics software, its importance in the business world, the process of integrating analytics into applications, how to choose the right software, the challenges and solutions associated with implementation, and future trends in this rapidly evolving field.
Understanding Embedded Analytics Software
Embedded analytics software refers to the integration of analytical capabilities directly into applications, empowering users to access and analyze data within the context of their workflows. This integration eliminates the need for users to switch between different tools or interfaces, streamlining the decision-making process and enhancing overall productivity.
Definition and Key Features
At its core, embedded analytics software allows users to analyze data within the context of their applications. It enables the creation of interactive dashboards, data visualizations, and reports, providing users with the necessary tools to explore and derive insights from their data. Key features of embedded analytics software include:
- Seamless Integration: Embedded analytics seamlessly integrates into existing applications, preserving the user experience and eliminating the need for additional logins or complex setups.
- Customization: It allows for customization, enabling businesses to tailor the analytics experience according to their unique requirements, branding, and workflows.
- Real-time Data: Embedded analytics provides access to real-time data, allowing users to monitor and respond to changing conditions promptly.
- Self-service Analytics: Users can interact with data independently, using intuitive interfaces and tools to explore and analyze data without extensive technical knowledge.
Importance of Embedded Analytics in Business
The integration of analytics into applications is increasingly vital in today's data-driven business landscape. Embedded analytics software empowers users to make informed decisions based on real-time insights, eliminating the need for manual data extraction and analysis. By leveraging the power of embedded analytics, businesses can:
- Enhance Decision-making: With embedded analytics, users can access and analyze data within the context of their workflows, enabling them to make data-driven decisions quickly and effectively.
- Improve User Experience: By integrating analytics capabilities into applications, businesses can provide users with a seamless experience, eliminating the need to switch between different tools or interfaces.
- Drive Operational Efficiency: Real-time data and self-service analytics enable users to identify and rectify inefficiencies promptly, optimizing business processes and enhancing overall productivity.
- Increase Competitiveness: Embedded analytics empowers businesses to extract insights from their data and gain a competitive advantage by making informed and proactive decisions.
Integration of Insights into Applications
The process of integrating analytics into applications involves several steps, each crucial for ensuring a successful implementation. By following a systematic approach, businesses can seamlessly integrate analytics capabilities into their applications and reap the benefits of embedded analytics software.
Process of Integrating Analytics into Applications
The integration process typically involves the following steps:
- Define Objectives: Clearly identify the goals and objectives of integrating analytics into your application. This step helps align the integration process with your desired outcomes.
- Identify Data Sources: Determine the data sources that will provide the necessary information for analysis within your application. This includes both internal and external data repositories.
- Data Extraction and Transformation: Extract data from the identified sources, transform it into a format suitable for analysis, and load it into a centralized repository.
- Choose an Analytics Framework: Select an analytics framework that best aligns with your application's requirements. Consider factors such as scalability, flexibility, and support for various data visualization and analysis techniques.
- Design and Develop Analytics Interfaces: Create intuitive user interfaces that allow users to interact with and analyze data effectively. Consider the user experience, visualizations, and ease of navigation.
- Implement Security Measures: Ensure that appropriate security measures are in place to protect sensitive data. Data access controls and encryption are essential components in ensuring data integrity and privacy.
- Test and Validate: Thoroughly test the integrated analytics capabilities within your application to ensure its functionality, performance, and user experience meet your expectations.
Benefits of Application-Integrated Analytics
Integrating analytics directly into applications offers numerous benefits for businesses:
- Improved Decision-making: By providing users with real-time insights, embedded analytics enhances decision-making processes, leading to more informed and data-driven choices.
- Increased User Adoption: A seamless integration of analytics into applications increases user adoption rates, as users can access and analyze data within familiar interfaces.
- Enhanced User Experience: Application-integrated analytics offers a cohesive user experience, enabling users to interact with data without the need for additional tools or interfaces.
- Scalability and Flexibility: Embedded analytics frameworks can scale with the growing data needs of businesses, accommodating increasing data volumes and varied analytical requirements.
Choosing the Right Embedded Analytics Software
When selecting an embedded analytics software for your business, several factors should be considered to ensure an optimal fit with your application and analytical requirements.
Factors to Consider
Consider the following factors before choosing an embedded analytics software:
- Scalability: Ensure the software can handle the volume of data and user load anticipated, both currently and in the future.
- Flexibility: Look for a solution that is flexible enough to accommodate various data types, integration options, and analysis techniques.
- Customization: The ability to customize the analytics experience to align with your application's branding and workflows is crucial for a seamless integration.
- Security: Ensure that the software meets stringent security requirements to protect sensitive data and maintain regulatory compliance.
- Integration Capabilities: Consider the ease of integration with your application, including compatibility with existing technologies and frameworks.
- Support and Maintenance: Evaluate the level of support provided by the vendor, including access to technical assistance, updates, and ongoing maintenance.
Top Embedded Analytics Software in the Market
There are several leading embedded analytics software solutions available in the market today. Some notable ones include:
- Looker: Looker offers a robust analytics platform that enables businesses to integrate powerful data insights directly into their applications.
- Tableau: Tableau provides a comprehensive suite of analytics tools that can be seamlessly integrated into applications, offering intuitive data exploration and visualization capabilities.
- Sisense: Sisense offers an end-to-end analytics platform that combines powerful data analysis capabilities with ease of integration and customization.
- Microsoft Power BI: Microsoft Power BI integrates with a wide range of applications, enabling users to create interactive visualizations and reports within their workflows.
Challenges and Solutions in Implementing Embedded Analytics
While the benefits of implementing embedded analytics software are undeniable, businesses may encounter challenges during integration. By addressing these challenges proactively, companies can ensure a seamless implementation process.
Common Obstacles in Integration
Some common challenges in implementing embedded analytics include:
- Data Complexity: Dealing with complex and diverse datasets can pose challenges during integration. Ensuring data quality and consistency is crucial for accurate analysis.
- Integration Complexity: Integrating analytics capabilities into existing applications may involve complex technical requirements and dependencies on external systems.
- Resource Constraints: Limited resources, including skilled personnel, time, and budget, can pose challenges during the implementation process.
- Cultural Resistance: Organizations may face internal resistance to change, as users may be accustomed to traditional methods of data analysis.
Effective Strategies for Overcoming Challenges
To overcome these challenges, businesses should consider the following strategies:
- Data Governance and Quality: Establish robust data governance practices to ensure data quality and integrity, enabling accurate analysis within the application.
- Streamlined Integration: Optimize the integration process through careful planning, leveraging pre-built connectors, and utilizing agile development methodologies.
- Invest in Training and Skill Development: Provide adequate training and upskilling opportunities to personnel involved in the implementation, ensuring they have the necessary skills to overcome technical challenges.
- Change Management: Implement a change management strategy that addresses cultural resistance and fosters user adoption through effective communication and training programs.
Future Trends in Embedded Analytics
The field of embedded analytics is continuously evolving, with several exciting developments on the horizon. Businesses must stay abreast of these trends to remain competitive in the data-driven landscape.
Predicted Developments in the Field
Some predicted developments in embedded analytics include:
- Advanced AI and Machine Learning: The integration of advanced AI and machine learning algorithms will enable applications to provide more accurate and insightful predictions and recommendations.
- Embedding Analytics in IoT Devices: As the Internet of Things (IoT) continues to expand, embedding analytics directly into IoT devices will enable real-time data analysis and insights at the edge.
- Augmented Analytics: Augmented analytics, combining natural language processing and machine learning, will enable users to extract insights from data using conversational interfaces.
- Data Storytelling: Embedded analytics will increasingly focus on data storytelling, enabling users to visualize and communicate insights effectively to stakeholders.
Preparing for the Future of Embedded Analytics
To prepare for the future of embedded analytics, businesses should consider the following:
- Invest in AI and Machine Learning: Embrace advanced AI and machine learning technologies to leverage predictive and prescriptive analytics capabilities.
- Explore IoT Integration: Assess the potential benefits of integrating analytics directly into IoT devices, enabling real-time data analysis at the edge.
- Adopt Augmented Analytics: Consider incorporating augmented analytics capabilities into your applications, facilitating more intuitive and natural interaction with data.
- Foster Data Literacy: Ensure that users are equipped with the necessary data analysis skills to effectively leverage embedded analytics and communicate insights.
Conclusion
Embedded analytics software offers businesses the opportunity to harness the power of data-driven insights within their applications. By seamlessly integrating analytics capabilities, users can make informed decisions in real-time, resulting in enhanced productivity, improved user experience, and increased competitiveness. The key to successful implementation lies in understanding the concept of embedded analytics, following a systematic integration process, choosing the right software, overcoming implementation challenges, and preparing for future trends. By leveraging the potential of embedded analytics software, businesses can unlock the true value of their data and propel themselves towards success in the data-driven era.
Ready to elevate your business's data-driven decision-making to the next level? Discover CastorDoc, the most reliable AI Agent for Analytics, designed to empower your teams with instant, trustworthy data answers. Experience the power of self-service analytics and unlock the full potential of your data stack with CastorDoc. Our platform is tailored to provide your business users with the autonomy and trust needed to access, understand, and utilize data effectively, ensuring informed decisions are made swiftly. Reduce the burden on your data teams and analysts and maximize your ROI by activating your data stack with CastorDoc. Try CastorDoc today and transform your application's embedded analytics experience.
You might also like
Get in Touch to Learn More
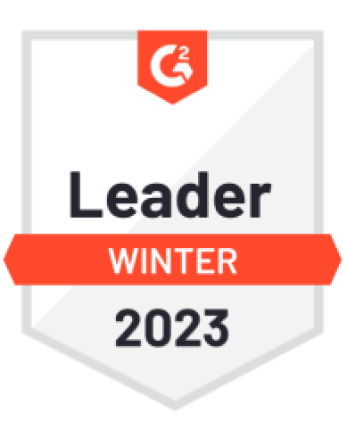
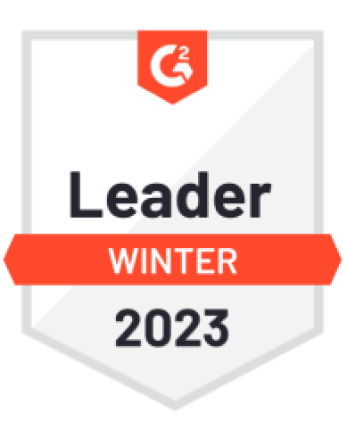

“[I like] The easy to use interface and the speed of finding the relevant assets that you're looking for in your database. I also really enjoy the score given to each table, [which] lets you prioritize the results of your queries by how often certain data is used.” - Michal P., Head of Data