How to Improve Accuracy in Natural Language Search
Learn effective strategies to enhance the precision and relevance of natural language search results.
Natural language search has revolutionized the way we interact with search engines. It allows us to ask questions using everyday language, rather than relying on specific keywords. However, ensuring the accuracy of natural language search results can be a complex task. In this article, we will explore different techniques to improve the accuracy of natural language search and address the challenges associated with it.
Understanding Natural Language Search
Natural language search refers to the ability of search engines to understand and interpret human language in order to provide relevant search results. To achieve this, search engines rely on two key components: semantics and syntax.
When it comes to natural language search, semantics plays a crucial role in deciphering the meaning behind the words and phrases used in a search query. It goes beyond just looking at individual words and takes into account the context in which they are used. By analyzing the semantics of a search query, search engines can better understand the user's intent and deliver more accurate and contextually relevant results.
The Role of Semantics in Natural Language Search
Semantics involves understanding the meaning behind the words and phrases used in a search query. It takes into account the context in which the words are used and the relationships between them. By analyzing the semantics of a search query, search engines can provide more accurate and contextually relevant results.
On the other hand, syntax in natural language search focuses on the grammatical structure of sentences. It helps search engines understand the order of words, the role of different parts of speech, and the overall grammatical correctness of a query. By correctly interpreting the syntax of a search query, search engines can provide more accurate and precise results.
The Importance of Syntax in Natural Language Search
Understanding the syntax of a search query is essential for search engines to accurately interpret the user's intent. By analyzing the grammatical structure of the query, search engines can better grasp the relationships between different elements and deliver search results that closely match what the user is looking for.
Techniques to Enhance Accuracy in Natural Language Search
There are several techniques that can be employed to enhance the accuracy of natural language search. One such technique is the utilization of machine learning algorithms.
Machine learning algorithms enable search engines to analyze large volumes of data and learn patterns and relationships between different words and phrases. By training these algorithms using relevant datasets, search engines can improve their understanding of natural language queries and provide more accurate search results.
Another important technique for enhancing accuracy in natural language search is the utilization of semantic search.
Semantic search focuses on the meaning behind words and phrases, rather than just the presence of keywords. By understanding the context and intent of a user's query, search engines can deliver more relevant results that match the user's true intent.
Utilizing Machine Learning for Improved Accuracy
In addition to machine learning, the advent of deep learning has also had a significant impact on natural language search accuracy.
Deep learning algorithms, which are a type of machine learning algorithms, use neural networks to simulate human-like learning and decision-making processes. By processing vast amounts of data, deep learning algorithms are able to uncover complex patterns in natural language and improve the accuracy of search results.
Furthermore, the integration of natural language processing (NLP) techniques has revolutionized the way search engines interpret and understand human language.
NLP allows search engines to analyze and derive meaning from unstructured data, such as text and speech, enabling them to provide more accurate and relevant search results based on the user's natural language queries.
The Impact of Deep Learning on Natural Language Search
Challenges in Improving Natural Language Search Accuracy
While there have been significant advancements in improving natural language search accuracy, there are still challenges that need to be addressed.
Dealing with Ambiguity in Natural Language Search
One of the challenges is dealing with ambiguity in search queries. Natural language is inherently ambiguous, and a single query can have multiple interpretations. Search engines need to understand the context and user intent behind a query in order to provide accurate results. This requires sophisticated algorithms and semantic analysis techniques.
Addressing ambiguity in natural language search involves implementing machine learning models that can analyze vast amounts of data to identify patterns and infer the most likely meaning of a query. By incorporating deep learning algorithms, search engines can better grasp the nuances of language and improve the accuracy of search results.
Overcoming the Problem of Context in Natural Language Search
Context plays a crucial role in natural language search. The same query can have different meanings depending on the context in which it is used. For example, the query "apple" can refer to the fruit or the technology company. Search engines need to consider the user's location, previous searches, and other contextual factors to provide accurate results.
Furthermore, advancements in natural language processing (NLP) have enabled search engines to incorporate contextual information more effectively. By utilizing techniques such as named entity recognition and sentiment analysis, search algorithms can better understand the context in which a query is made and tailor the results accordingly. This contextual understanding enhances the relevance of search results and improves the overall user experience.
Future Trends in Natural Language Search
The field of natural language search is constantly evolving, with new advancements and trends shaping its future.
As technology continues to advance, one of the key areas driving innovation in natural language search is the integration of voice search capabilities. With the rise of virtual assistants like Siri, Alexa, and Google Assistant, users are increasingly relying on voice commands to perform searches. This shift towards voice search requires search engines to adapt to conversational language patterns and understand spoken queries, presenting a new frontier for natural language search development.
The Role of AI in Advancing Natural Language Search
Artificial Intelligence (AI) is expected to play a significant role in advancing natural language search. AI-powered algorithms can understand and analyze the nuances of human language, leading to more accurate search results. By leveraging AI, search engines can continuously improve their understanding of natural language queries and deliver highly relevant results to users.
Furthermore, the application of AI in natural language search extends beyond traditional text-based queries. Image and video recognition technologies powered by AI are also being integrated into search engines, allowing users to perform visual searches using natural language descriptions. This fusion of AI capabilities with different types of search modalities is revolutionizing the way users interact with search engines.
Predictive Analytics and Natural Language Search
Predictive analytics, which involves using historical data and statistical algorithms to make predictions, can further enhance the accuracy of natural language search. By analyzing user behavior and preferences, search engines can anticipate user intent and deliver more personalized and accurate search results.
Moreover, the evolution of natural language search is not limited to traditional web search engines. E-commerce platforms are also leveraging natural language processing and machine learning techniques to enhance product search functionalities. By understanding user queries in natural language, e-commerce sites can provide tailored product recommendations and streamline the shopping experience for customers.
In conclusion, improving accuracy in natural language search is an ongoing challenge, but advancements in machine learning, deep learning, and AI are driving significant progress. By understanding the role of semantics and syntax, utilizing advanced techniques, and addressing challenges such as ambiguity and context, search engines can enhance the accuracy of natural language search and provide users with more accurate and relevant search results.
As we've discussed the importance of improving accuracy in natural language search, it's clear that the right tools can make a significant difference. CastorDoc stands at the forefront of this technology, integrating advanced governance with a user-friendly AI assistant to enhance self-service analytics. If you're looking to empower your data teams and business users with the ability to conduct natural language searches that are both accurate and contextually relevant, try CastorDoc today and experience a new era of data accessibility and informed decision-making.
You might also like
Get in Touch to Learn More
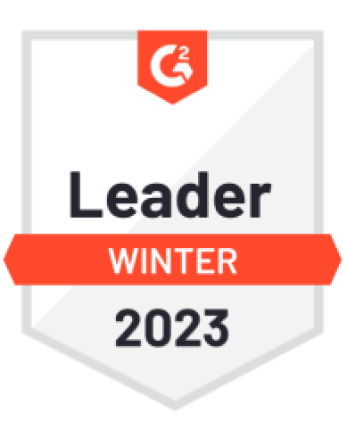
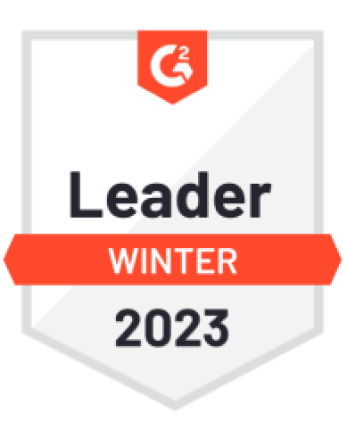

“[I like] The easy to use interface and the speed of finding the relevant assets that you're looking for in your database. I also really enjoy the score given to each table, [which] lets you prioritize the results of your queries by how often certain data is used.” - Michal P., Head of Data