Semantic Data Models: The Secret Weapon for Unlocking Deeper Business Insights
Uncover the power of semantic data models in our latest article! Discover how these innovative tools can revolutionize your business insights, providing a deeper understanding of your data and unlocking untapped potential.
Semantic data models play a pivotal role in extracting valuable insights from vast amounts of business data. By organizing and structuring data in a way that reflects the real-world entities and relationships, semantic data models provide a powerful framework for understanding and analyzing complex business information. In this article, we will explore the basics of semantic data models, their importance in business intelligence, the benefits they offer, the challenges they pose, and the future trends in semantic data modeling.
Understanding the Basics of Semantic Data Models
At the core of semantic data modeling is the concept of semantics, which refers to the meaning and interpretation of data. Semantic data models focus on capturing the inherent meaning of data elements and their relationships, enabling more efficient data integration, analysis, and interpretation.
Defining Semantic Data Models:
A semantic data model represents the relationships between entities in a domain-specific context. It defines the structure, properties, and associations of data elements, allowing for a comprehensive understanding of the data and its connections.
When creating a semantic data model, data architects carefully consider the semantics of the data elements and how they relate to each other. This process involves identifying entities, attributes, and relationships, as well as defining constraints and business rules to ensure data integrity and consistency.
The Importance of Semantic Data Models in Business:
Businesses generate a vast amount of data from various sources, including customer interactions, transactions, and market trends. However, to extract meaningful insights, it is crucial to have a clear understanding of the underlying semantics of this data.
Semantic data models enable businesses to:
- Efficiently integrate and harmonize data from disparate sources by providing a common language and structure.
- Uncover hidden patterns and relationships within the data, leading to valuable insights and predictive analysis.
- Facilitate data governance and ensure data quality and consistency.
Moreover, semantic data models play a vital role in supporting advanced analytics and machine learning initiatives within organizations. By structuring data in a way that emphasizes meaning and context, these models enhance the accuracy and effectiveness of analytical algorithms, resulting in more precise predictions and actionable insights.
Furthermore, the use of semantic data models can improve collaboration and communication across different departments and teams within a company. By establishing a common understanding of data semantics, employees from various disciplines can work together more effectively, leading to better decision-making and streamlined business processes.
The Role of Semantic Data Models in Business Intelligence
Semantic data models are at the core of business intelligence (BI) systems. They play a crucial role in enhancing the effectiveness and efficiency of BI processes.
Enhancing Data Integration with Semantic Models:
Traditional data integration approaches often struggle with the complexity and diversity of data. However, by leveraging semantic data models, businesses can overcome these challenges and achieve a unified view of their data assets.
Semantic models enable:
- Mapping and transformation of data from different sources into a consistent format.
- Identification and resolution of semantic conflicts and inconsistencies.
- Automatic data enrichment through the discovery of interrelationships between data elements.
For example, let's consider a scenario where a company collects customer data from various sources, such as online transactions, social media interactions, and customer surveys. Without a semantic data model, integrating and analyzing this data would be a daunting task. However, by utilizing semantic models, the company can effortlessly map and transform the data into a consistent format, ensuring accurate and reliable analysis.
Semantic Models and Predictive Analysis:
Predictive analysis is a vital component of business intelligence, empowering organizations to anticipate future trends and make informed decisions. Semantic data models provide a solid foundation for predictive analysis by capturing the contextual knowledge and semantic relationships within the data.
With semantic data models, organizations can:
- Identify relevant variables and features for predictive models.
- Enhance the accuracy and reliability of predictive algorithms through data enrichment.
- Discover hidden patterns and correlations that contribute to accurate predictions.
For instance, imagine a retail company that wants to predict customer churn. By utilizing semantic data models, the company can identify the relevant variables, such as purchase history, customer demographics, and customer sentiment from social media. These models not only enhance the accuracy and reliability of the predictive algorithm but also enable the discovery of hidden patterns and correlations that contribute to accurate predictions.
In conclusion, semantic data models are essential in business intelligence systems. They enable seamless data integration and provide a solid foundation for predictive analysis. By leveraging semantic models, organizations can overcome data complexity, achieve a unified view of their data assets, and make informed decisions based on accurate predictions.
The Benefits of Implementing Semantic Data Models
Implementing semantic data models offers several compelling benefits for businesses seeking to derive meaningful insights from their data assets.
Improved Data Quality and Consistency:
One of the key advantages of semantic data models is their ability to ensure data quality and consistency. By enforcing standardized definitions, formats, and relationships, semantic models enable organizations to minimize data errors and inconsistencies.
Enhanced Decision-Making Process:
Accurate and timely decision-making is crucial for business success. Semantic data models enable organizations to make informed decisions by providing a comprehensive understanding of the underlying data. By uncovering hidden relationships and dependencies, businesses can gain deeper insights into customer behavior, market trends, and operational efficiency.
Increased Data Integration Capabilities:
Another significant benefit of implementing semantic data models is the enhancement of data integration capabilities. Semantic models facilitate the integration of data from disparate sources by providing a common framework for understanding and mapping data elements. This interoperability allows organizations to combine data from various systems and sources, enabling a more holistic view of their operations and performance.
Facilitates Data Governance and Compliance:
Effective data governance is essential for ensuring data security, privacy, and regulatory compliance. Semantic data models play a crucial role in facilitating data governance by establishing clear data definitions, ownership, and usage policies. By implementing semantic models, organizations can better manage and control their data assets, reducing the risk of data breaches and non-compliance with regulatory requirements.
Overcoming Challenges in Semantic Data Modeling
While semantic data modeling offers immense benefits, it also presents challenges that organizations must address to leverage its full potential.
Dealing with Complex Data Structures:
Businesses often deal with highly complex data structures that include nested hierarchies, multi-valued attributes, and other intricacies. Semantic data modeling requires careful handling of these complexities to ensure accurate representation and analysis of the data.
Ensuring Data Security and Privacy:
As organizations gather and analyze increasing amounts of data, ensuring data security and privacy becomes paramount. Semantic data models must incorporate appropriate data access controls, encryption mechanisms, and pseudonymization techniques to protect sensitive business information.
Addressing Data Quality Challenges:
Another significant challenge in semantic data modeling is maintaining data quality. Data from various sources may have inconsistencies, errors, or missing values, which can affect the accuracy of semantic models. Organizations need robust data quality processes, such as data cleansing, normalization, and validation, to ensure the reliability of their semantic data models.
Scaling Semantic Models for Big Data:
With the exponential growth of data in today's digital age, organizations face the challenge of scaling their semantic models to handle big data effectively. This involves optimizing storage, processing capabilities, and query performance to accommodate large volumes of data while maintaining the semantic integrity of the models.
Future Trends in Semantic Data Modeling
The field of semantic data modeling is continually evolving, driven by advancements in technology and emerging business needs. Here are two prominent trends shaping the future of semantic data modeling:
The Rise of Semantic AI in Business:
Semantic AI combines the power of artificial intelligence and semantic data models to deliver intelligent, context-aware insights. By leveraging natural language processing (NLP) algorithms and machine learning techniques, businesses can extract valuable insights from unstructured data sources such as social media feeds, customer reviews, and industry reports.
With the rise of semantic AI, businesses are able to gain a deeper understanding of their customers and market trends. For example, a retail company can analyze customer reviews to identify patterns and sentiments, allowing them to tailor their marketing strategies accordingly. Additionally, semantic AI can assist in automating customer support processes by understanding and responding to customer queries in a more personalized and efficient manner.
Semantic Models and Big Data Analytics:
As the volume, velocity, and variety of data continue to grow, businesses must explore new ways to handle big data. Semantic data models provide an effective means of structuring and analyzing massive datasets, enabling businesses to rapidly gain insights and make data-driven decisions.
By utilizing semantic data models in big data analytics, businesses can uncover hidden patterns and correlations that were previously difficult to identify. For instance, a healthcare organization can analyze large volumes of patient data to identify risk factors for certain diseases and develop targeted preventive measures. Furthermore, semantic data models can enhance data governance and data integration processes, ensuring data quality and consistency across various systems and sources.
In conclusion, semantic data models are the secret weapon for unlocking deeper business insights. By harnessing the power of semantics, businesses can organize, integrate, and analyze data more effectively. With improved data quality, enhanced decision-making processes, and the ability to overcome complex data challenges, semantic data models offer a transformative approach to business intelligence. Looking ahead, the rise of semantic AI and the continued evolution of big data analytics will further propel the adoption of semantic data modeling in businesses across industries.
Ready to harness the transformative power of semantic data models and unlock deeper business insights? CastorDoc is here to elevate your data journey. As the most reliable AI Agent for Analytics, CastorDoc empowers your teams with instant, trustworthy data answers, enabling self-service analytics and informed decision-making. Experience how CastorDoc can activate your data stack's ROI and give your business users the autonomy and trust they need. Try CastorDoc today and move forward with confidence in your strategic challenges.
You might also like
Get in Touch to Learn More
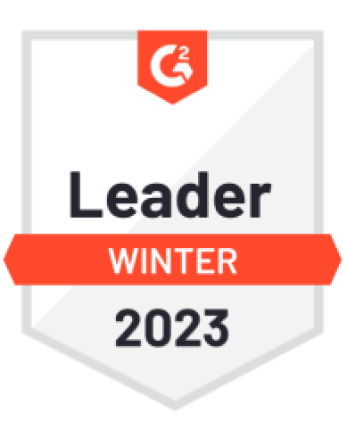
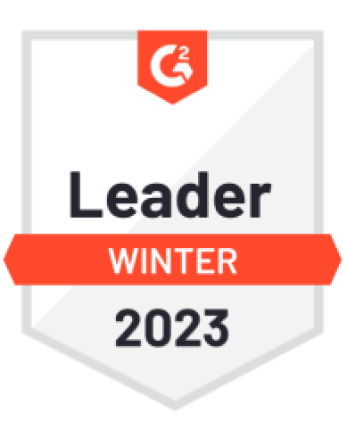

“[I like] The easy to use interface and the speed of finding the relevant assets that you're looking for in your database. I also really enjoy the score given to each table, [which] lets you prioritize the results of your queries by how often certain data is used.” - Michal P., Head of Data