Implementing a Semantic Layer in Databricks
Learn how to enhance your data analytics capabilities by implementing a semantic layer in Databricks.
In today's data-driven world, organizations are constantly seeking innovative ways to make sense of their vast amounts of data. One such solution that has gained considerable momentum is the implementation of a semantic layer in Databricks. By leveraging the power of Apache Spark and Delta Lake, this cutting-edge technology enables users to extract meaningful insights and deliver accurate information to business users. In this article, we will explore the concept of a semantic layer, its importance in data management, and the steps to successfully implement it in Databricks.
Understanding the Concept of a Semantic Layer
Before diving into the implementation process, it is crucial to have a clear understanding of what a semantic layer is and why it plays a pivotal role in data management. Put simply, a semantic layer acts as an intermediary between the raw, complex data sources and the end users who require simplified and consistent information.
Think of it as a translation mechanism that translates technical jargon and complex data structures into a common language that business users can easily comprehend. By providing a logical and unified view of the underlying data, a semantic layer eliminates the need for users to have extensive knowledge of databases or complex query languages.
Definition and Importance of a Semantic Layer
At its core, a semantic layer represents a semantic model that defines the relationships and hierarchies within the data. It provides a conceptual representation of the data, abstracting away the underlying complexities.
The importance of a semantic layer cannot be overstated when it comes to data management. It enables organizations to foster a data-driven culture by empowering business users to explore and analyze data without extensive technical knowledge or reliance on IT teams.
Furthermore, a well-designed semantic layer promotes data consistency and accuracy by enforcing standardized business rules and definitions. This ensures that everyone within the organization is working with a single version of the truth.
Role of a Semantic Layer in Data Management
A semantic layer enhances data management by providing several key benefits. Firstly, it simplifies data access and retrieval by offering a user-friendly interface to query and analyze data. Business users can leverage intuitive tools and dashboards to effortlessly navigate through the data, empowering them to make informed decisions.
Secondly, a semantic layer enables data governance and control by enforcing security measures and access controls at a granular level. System administrators can define and manage user roles and permissions, ensuring that sensitive data is only accessible to authorized personnel.
Lastly, a semantic layer facilitates data integration and consolidation by harmonizing disparate data sources into a unified view. This ensures that data from various systems and departments can be seamlessly combined, providing a comprehensive and holistic understanding of the organization's operations.
But the benefits of a semantic layer don't stop there. It also plays a crucial role in data quality management. By defining and enforcing data validation rules, a semantic layer helps organizations maintain high-quality data, free from errors and inconsistencies.
Additionally, a semantic layer enables data lineage and traceability, allowing organizations to track the origin and transformation of data throughout its lifecycle. This not only enhances data governance but also facilitates compliance with regulatory requirements.
Moreover, a semantic layer promotes collaboration and knowledge sharing within an organization. By providing a common understanding of data, it enables different teams and departments to work together seamlessly, fostering cross-functional collaboration and driving innovation.
Overall, a well-implemented semantic layer is a fundamental building block for effective data management. It empowers organizations to harness the full potential of their data, enabling data-driven decision-making, improving operational efficiency, and driving business growth.
Databricks: A Brief Overview
Now that we have delved into the fundamentals of a semantic layer, let's turn our attention to Databricks - the platform that serves as the foundation for implementing this technology. Databricks is a unified analytics and data engineering platform that brings together the power of Apache Spark and Delta Lake.
Key Features of Databricks
Databricks offers a plethora of features that make it an ideal choice for implementing a semantic layer. Firstly, its scalable and distributed processing capabilities, powered by Apache Spark, enable organizations to analyze massive volumes of data in real-time.
Furthermore, Databricks provides a collaborative environment for data scientists, analysts, and engineers to work together seamlessly. Its notebook interface promotes collaborative coding, making it easier to develop, test, and iterate on data processing pipelines.
Additionally, Databricks integrates seamlessly with various data sources and tools, allowing organizations to leverage their existing investments. It supports a wide range of data formats, databases, and data lakes, enabling users to extract insights from diverse data sources.
Benefits of Using Databricks for Data Processing
Implementing a semantic layer in Databricks offers numerous benefits that accelerate data processing and analysis. Firstly, Databricks provides an optimized and parallelized execution framework, resulting in faster processing times and improved performance.
Furthermore, Databricks' integration with Delta Lake ensures data reliability and integrity. Delta Lake brings transactional capabilities to the data lake, enabling users to perform atomic updates and maintain multiple versions of the data.
Moreover, Databricks offers a zero-management platform that handles infrastructure provisioning and auto-scaling, freeing up resources and reducing operational overhead. This allows organizations to focus on data analysis and strategic decision-making rather than infrastructure management.
Steps to Implement a Semantic Layer in Databricks
Now that we have established the importance of a semantic layer and explored the features of Databricks, let's delve into the steps involved in implementing a semantic layer in Databricks.
Preparing Your Data for the Semantic Layer
The first step in implementing a semantic layer is to prepare your data for analysis. This involves identifying relevant data sources, understanding the data schema, and cleansing and transforming the data as necessary.
Databricks provides a rich set of data processing libraries and functions that simplify these tasks. Leveraging the power of Apache Spark, you can perform complex data transformations, filter out irrelevant data, and aggregate the data to prepare it for the semantic layer.
Building the Semantic Layer
Once the data is prepared, the next step is to build the semantic layer. In Databricks, this can be achieved by leveraging various SQL-based tools and libraries such as Delta Lake and Apache Spark SQL.
Delta Lake, with its ACID transactions and schema enforcement capabilities, provides a robust foundation for building the semantic layer. You can define tables, views, and materialized views to represent the logical structure of the data and enforce business rules.
Apache Spark SQL, on the other hand, enables you to query and manipulate the data using SQL-like syntax. It provides a familiar and intuitive interface for business users to analyze the data without the need for writing complex code.
Testing and Validating the Semantic Layer
Once the semantic layer is built, it is crucial to thoroughly test and validate its accuracy and performance. This involves conducting extensive data quality checks, testing the semantic layer against real-world scenarios, and ensuring that the results align with the expected outcomes.
By performing comprehensive testing, you can identify and rectify any issues or discrepancies in the semantic layer, ensuring that the information delivered to business users is reliable and consistent.
Challenges in Implementing a Semantic Layer in Databricks
While implementing a semantic layer in Databricks offers numerous advantages, it is not without its challenges. Understanding and addressing these challenges is vital to ensure a successful implementation.
Common Obstacles and How to Overcome Them
One common challenge in implementing a semantic layer is ensuring alignment between business requirements and the underlying data model. This can be overcome by involving business stakeholders early in the process, conducting regular feedback sessions, and iterating on the semantic layer based on their inputs.
Another challenge is data governance and security. It is essential to define robust access controls and implement data masking and encryption techniques to protect sensitive data.
Ensuring Data Quality and Consistency
Data quality and consistency are of paramount importance in a semantic layer. Ensuring that the data is accurate, valid, and up-to-date requires implementing data validation mechanisms, performing regular data audits, and monitoring data sources for any discrepancies.
Moreover, establishing well-defined business rules and incorporating data profiling techniques can help identify and rectify inconsistencies, guaranteeing data integrity and reliability.
Optimizing the Performance of Your Semantic Layer in Databricks
An effective semantic layer not only delivers accurate information but also performs optimally to meet the ever-increasing demands of data analysis. These best practices ensure optimal performance and efficiency.
Best Practices for Performance Optimization
To optimize the performance of your semantic layer, it is crucial to leverage the inherent capabilities of Databricks. This includes partitioning the data, leveraging Delta Lake's indexing and caching features, and optimizing SQL queries using techniques such as query pruning and predicate pushdown.
Furthermore, monitoring the performance of the semantic layer using Databricks' built-in monitoring tools allows you to identify bottlenecks and fine-tune the system accordingly.
Regular Maintenance and Updates
Lastly, regular maintenance and updates are essential to ensure the longevity and efficiency of your semantic layer. This involves monitoring and managing data sources, incorporating new data into the semantic layer, and evolving the semantic model as business requirements and data sources change.
By maintaining a proactive approach to maintenance and updates, you can guarantee that your semantic layer remains up-to-date, relevant, and aligned with the evolving needs of your organization.
In conclusion, implementing a semantic layer in Databricks empowers organizations to harness the true power of their data. By providing a simplified, unified view of the underlying data, a semantic layer enhances data management, accelerates data analysis, and promotes data-driven decision-making. By following the steps outlined in this article and adopting best practices, organizations can unlock the full potential of their data, revolutionizing the way they derive insights and drive business success.Ready to elevate your data management and analytics capabilities to the next level? CastorDoc is here to seamlessly guide your business through the strategic challenges of data analysis. With our cutting-edge AI Agent for Analytics, you can empower your teams to make data-driven decisions with confidence and ease. Experience the freedom of self-service analytics and the efficiency of a data stack that delivers maximum ROI. Don't let data literacy barriers hold you back. Try CastorDoc today and unlock the full potential of your organization's data.
You might also like
Get in Touch to Learn More
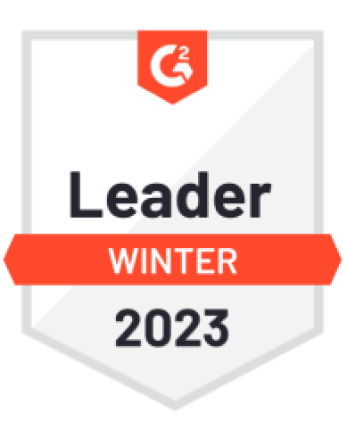
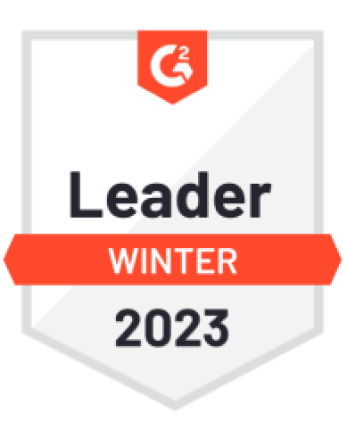

“[I like] The easy to use interface and the speed of finding the relevant assets that you're looking for in your database. I also really enjoy the score given to each table, [which] lets you prioritize the results of your queries by how often certain data is used.” - Michal P., Head of Data