The Semantic Data Layer Demystified: Organizing and Structuring Data for Meaning and Context
Uncover the secrets of the semantic data layer in this insightful article, as we delve into the art of organizing and structuring data for enhanced meaning and context.
In today's fast-paced digital world, data is abundant and plays a crucial role in decision-making processes across various industries. However, the sheer volume and complexity of data can often create a challenge when it comes to extracting meaningful insights. This is where the concept of a semantic data layer comes into play. By organizing and structuring data for meaning and context, a semantic data layer enables businesses to harness the full potential of their data assets.
Understanding the Basics of Semantic Data Layer
A semantic data layer can be defined as a structured framework that provides a common language for data, facilitating better understanding and interpretation. Its primary function is to establish relationships between different data elements, allowing for seamless integration and interoperability. In simple terms, it acts as a bridge between raw data and human comprehension, unlocking valuable insights that would otherwise remain hidden.
Defining Semantic Data Layer
The semantic data layer can be thought of as a virtual layer that sits between the backend data sources and the frontend applications. It acts as an intermediary, transforming raw data into a more organized and meaningful format. It utilizes standardized vocabularies, such as ontologies and taxonomies, to define the structure of data and the relationships between different entities. This enables applications to retrieve and process data in a more efficient and intuitive manner.
Importance of Semantic Data Layer in Data Management
Effective data management is crucial for businesses looking to unlock the true potential of their data assets. With the proliferation of data sources and formats, organizations often struggle with data integration and consistency. A semantic data layer addresses these challenges by providing a unified framework for organizing and structuring data. It promotes data consistency, reduces redundancy, and improves data quality, ensuring that stakeholders can trust the information they are working with.
Furthermore, a semantic data layer also plays a vital role in data governance. By establishing clear rules and guidelines for data management, it helps organizations maintain compliance with regulatory requirements and industry standards. This ensures that sensitive data is protected and handled appropriately, mitigating the risk of data breaches and legal consequences.
Moreover, the benefits of a semantic data layer extend beyond internal data management. It also facilitates data sharing and collaboration with external partners and stakeholders. By providing a standardized format for data exchange, it enables seamless integration with third-party systems, fostering interoperability and enhancing business partnerships.
Organizing Data in the Semantic Layer
The principles of data organization in a semantic layer revolve around creating a logical and hierarchical structure that reflects the relationships between different data elements. This allows for easy navigation and exploration of data, resulting in more meaningful insights.
When designing a semantic layer, it is crucial to consider not only the current data needs but also future scalability and flexibility. By anticipating potential growth and changes in data requirements, organizations can build a semantic layer that is robust and adaptable. This forward-thinking approach ensures that the data organization remains effective in the long run.
Principles of Data Organization
One of the key principles of data organization is the concept of classification. By categorizing entities into different classes, businesses can establish a clear hierarchy and enable more efficient data retrieval. Additionally, the use of attributes and properties helps to provide more granular information about each entity, facilitating a more comprehensive understanding.
Another important aspect of data organization is the concept of data governance. Implementing data governance practices ensures that data is accurate, consistent, and secure throughout its lifecycle. By defining data ownership, quality standards, and access controls, organizations can maintain the integrity of their data in the semantic layer.
Techniques for Effective Data Organization
There are various techniques that can be employed to ensure effective data organization in a semantic layer. One such technique is the use of controlled vocabularies, which define standardized terms that are used consistently across the organization. This promotes data consistency and helps to eliminate ambiguity. Another technique is the utilization of metadata, which provides additional information about the data, such as its source, format, and quality. This helps to enhance data discoverability and allows for better decision-making.
Furthermore, data lineage is a critical aspect of effective data organization in the semantic layer. By documenting the origins and transformations of data as it moves through various processes, organizations can track data quality issues, ensure regulatory compliance, and improve overall data transparency. Understanding data lineage also helps in identifying dependencies and impacts when making changes to the data structure or architecture.
Structuring Data for Meaning and Context
In order to derive meaningful insights from data, it is crucial to structure it in a way that captures its inherent meaning and context. Without proper structuring, data can be overwhelming, making it difficult for stakeholders to make sense of it.
Effective data structuring involves organizing and categorizing data elements in a logical and coherent manner. This process not only simplifies data management but also enables easier retrieval and analysis. By structuring data with a clear understanding of its context and intended use, organizations can harness its full potential to drive strategic decision-making and innovation.
The Role of Context in Data Structuring
Context plays a vital role in data structuring. By taking into account the specific domain or industry in which the data is being utilized, businesses can structure their data in a manner that is relevant and actionable. For example, in the healthcare industry, data may be structured to capture patient demographics, medical conditions, and treatment outcomes. By considering the context in which the data is being used, businesses can unlock valuable insights and drive informed decision-making.
Furthermore, context-aware data structuring involves understanding the relationships between different data points and how they contribute to the overall narrative. By contextualizing data elements within a broader framework, organizations can gain a more holistic view of their operations and performance, leading to more effective strategies and outcomes.
Strategies for Meaningful Data Structuring
When it comes to structuring data for meaning and context, there are several strategies that can be employed. One such strategy is the use of ontologies, which define the relationships between different entities and concepts within a specific domain. By leveraging ontologies, businesses can establish a common understanding of the data and facilitate interoperability across different systems. Additionally, the use of semantic annotations, such as RDF (Resource Description Framework), can enhance the meaning and context of data by providing additional descriptive information.
Another effective strategy for meaningful data structuring is the adoption of data modeling techniques that align with the organization's objectives and analytical requirements. By designing data models that reflect the underlying business processes and goals, companies can ensure that their data structures are optimized for decision support and predictive analytics. This proactive approach to data structuring not only enhances data quality and consistency but also empowers users to extract valuable insights with confidence.
Challenges in Implementing Semantic Data Layer
While the benefits of a semantic data layer are significant, implementing it can present certain challenges. Organizations need to be aware of these challenges and develop strategies to overcome them.
Common Obstacles in Semantic Data Layer Implementation
One common challenge in implementing a semantic data layer is the lack of data governance. Without proper governance, data can become fragmented and inconsistent, making it difficult to establish meaningful relationships. Another challenge is the complexity of data integration. Organizations often have multiple data sources with different formats, making it challenging to integrate them seamlessly into a semantic layer. Additionally, the lack of awareness and understanding about the benefits of a semantic data layer can hinder adoption.
Overcoming Challenges in Semantic Data Layer Implementation
To overcome the challenges associated with implementing a semantic data layer, organizations need to prioritize data governance. This involves establishing clear guidelines for data management, including data quality standards, metadata management, and data stewardship. Additionally, organizations should invest in technologies and tools that facilitate data integration and interoperability. This includes utilizing ETL (Extract, Transform, Load) processes and leveraging APIs (Application Programming Interfaces) for data exchange. Finally, raising awareness and providing training on the benefits of a semantic data layer can drive adoption and ensure organizational buy-in.
The Future of Semantic Data Layers
As businesses continue to recognize the importance of organizing and structuring data for meaning and context, the future of semantic data layers looks promising.
Emerging Trends in Semantic Data Layers
One emerging trend in semantic data layers is the use of machine learning and artificial intelligence. These technologies can enhance the capabilities of a semantic layer by automating data structuring and analysis, enabling faster and more accurate insights. Another trend is the adoption of industry-specific ontologies, which capture the unique characteristics and relationships within a particular domain. This allows businesses to leverage pre-defined structures and minimize the effort required for data organization.
Predictions for Future Developments in Semantic Data Layers
In the future, we can expect to see further advancements in semantic data layers. One development could be the integration of external data sources, such as social media feeds and IoT (Internet of Things) devices, into the semantic layer. This would enable businesses to gain a more comprehensive understanding of their data ecosystem and uncover new insights. Additionally, advancements in natural language processing and data visualization techniques will make semantic data layers more accessible to non-technical users, democratizing data-driven decision-making.
In conclusion, the semantic data layer is a powerful tool that enables businesses to organize and structure data for meaning and context. By establishing relationships between different data elements and capturing the inherent meaning of data, organizations can unlock valuable insights and make informed decisions. While implementing a semantic data layer may present challenges, organizations that prioritize data governance, invest in integration technologies, and raise awareness about its benefits will be well-positioned to harness the full potential of their data assets. As the industry continues to evolve, the future of semantic data layers looks promising, with emerging trends such as machine learning and artificial intelligence driving further advancements in data organization and analysis. Embracing the semantic data layer is no longer a luxury but a necessity for businesses looking to thrive in the data-driven era.
Ready to elevate your business's data strategy and overcome the challenges of implementing a semantic data layer? CastorDoc is here to guide you through the process. As the most reliable AI Agent for Analytics, CastorDoc empowers your teams with trustworthy, instantaneous data answers, enabling self-service analytics and breaking down data literacy barriers. Maximize the ROI of your data stack and give your business users the autonomy and trust they need to make informed decisions. Try CastorDoc today and start truly activating your data's potential.
You might also like
Get in Touch to Learn More
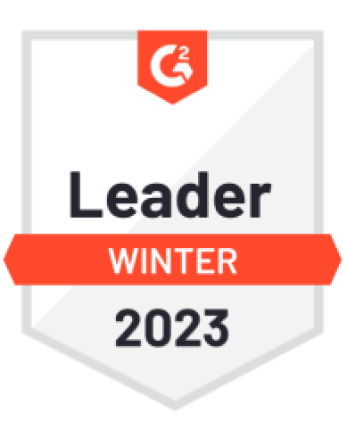
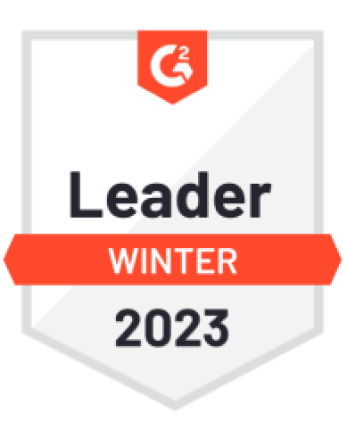

“[I like] The easy to use interface and the speed of finding the relevant assets that you're looking for in your database. I also really enjoy the score given to each table, [which] lets you prioritize the results of your queries by how often certain data is used.” - Michal P., Head of Data