Understanding LLM Schema for Data Structuring
Unlock the power of data structuring with this comprehensive guide to understanding LLM schema.
LLM Schema is a crucial aspect of data structuring that plays a significant role in maintaining consistency and facilitating integration. In this article, we will delve into the depths of LLM Schema and explore its various components, importance, and challenges. We will also discuss the future trends in LLM Schema and its relevance in data management.
Defining LLM Schema
At its core, LLM Schema is a structured format that defines the organization and layout of data within a system. It provides a blueprint for organizing and structuring data elements, ensuring consistency and coherence across different datasets. LLM Schema acts as a foundation for data integration, enabling smooth interoperability and efficient information exchange.
LLM Schema plays a crucial role in data management by serving as a common language for different systems to communicate effectively. By defining the structure and relationships of data elements, LLM Schema facilitates seamless data flow and enhances data quality. This standardized approach not only streamlines data processing but also improves the overall accuracy and reliability of information.
The Basics of LLM Schema
The basics of LLM Schema revolve around defining the structure, attributes, and relationships of the data elements. It involves specifying the data types, constraints, and the hierarchy of the data elements. This enables effective data representation and retrieval, ensuring that the information is stored in a logical and meaningful manner.
Furthermore, LLM Schema provides a framework for data governance and security. By clearly outlining the rules and standards for data organization, LLM Schema helps in maintaining data quality and protecting sensitive information. This structured approach not only enhances data security but also ensures compliance with regulatory requirements and industry standards.
Key Components of LLM Schema
The key components of LLM Schema include entities, attributes, relationships, and constraints. Entities represent the real-world objects or concepts that the data describes. Attributes define the characteristics of the entities. Relationships establish connections between entities, determining how they interact and relate to each other. Constraints impose rules on the data, ensuring data integrity and enforcing business rules.
Moreover, LLM Schema supports data scalability and flexibility by allowing for the expansion and modification of data structures as business needs evolve. This adaptability ensures that the data architecture remains agile and responsive to changing requirements, enabling organizations to stay competitive in dynamic market environments. By incorporating best practices and industry standards, LLM Schema serves as a cornerstone for effective data management and decision-making processes.
Importance of LLM Schema in Data Structuring
LLM Schema plays a crucial role in data structuring, offering several benefits that enhance data consistency and facilitate seamless integration across diverse systems.
When delving deeper into the realm of LLM Schema, it becomes evident that its impact extends far beyond just structuring data. The schema serves as a blueprint, guiding not only the organization of data but also the relationships and dependencies within the dataset. This comprehensive approach ensures that data is not only structured uniformly but also interconnected in a meaningful way, enhancing the overall data quality and usability.
Enhancing Data Consistency with LLM Schema
By defining a standardized structure for data representation, LLM Schema ensures uniformity and consistency in how data is organized and stored. This consistency eliminates data redundancies and discrepancies, reducing the chances of data inconsistency and ensuring reliable and accurate information retrieval.
Furthermore, the utilization of LLM Schema fosters a culture of data governance within organizations. By adhering to a predefined schema, data management practices become more structured and disciplined, leading to improved data quality and integrity. This proactive approach not only enhances data consistency but also instills trust in the data-driven decision-making process.
Facilitating Data Integration through LLM Schema
The use of LLM Schema helps in smooth data integration across different systems and applications. It provides a common language and framework for sharing and exchanging data, allowing disparate systems to communicate effectively. This simplifies data integration efforts, facilitating efficient data flow and enabling organizations to leverage the power of interconnected systems.
Moreover, the interoperability enabled by LLM Schema extends beyond just technical integration. It fosters collaboration and synergy among different departments within an organization by breaking down data silos and promoting cross-functional data sharing. This holistic approach to data integration not only streamlines operational processes but also nurtures a data-driven culture where insights are derived from a unified and comprehensive view of the organization's data landscape.
Working with LLM Schema
To effectively utilize LLM Schema for data structuring, it is crucial to understand the process of creating and modifying an LLM Schema.
LLM Schema, short for Logical Level Modeling Schema, serves as a blueprint for organizing and defining the structure of data within a database system. It provides a framework for developers to design databases that meet specific business requirements and ensure data integrity and consistency.
Creating an LLM Schema
The process of creating an LLM Schema involves analyzing the data requirements, identifying the entities and attributes, defining relationships, and specifying constraints. This process requires a thorough understanding of the data and its intended use. Using specialized tools and techniques, developers can create a well-defined LLM Schema that aligns with the organizational needs.
During the creation of an LLM Schema, developers also need to consider normalization techniques to eliminate data redundancy and improve data integrity. Normalization involves organizing data into multiple related tables to reduce data duplication and dependency, leading to a more efficient and robust database design.
Modifying an Existing LLM Schema
As data needs evolve, it becomes necessary to modify the existing LLM Schema to incorporate new requirements. Modifying an LLM Schema involves making alterations to the structure, attributes, relationships, or constraints. This requires careful consideration of the impact on existing data and systems, ensuring minimal disruption to the overall data structure.
When modifying an existing LLM Schema, developers must also pay attention to data migration strategies to ensure a smooth transition from the old schema to the new one. Data migration involves transferring data from one database schema to another while maintaining data quality, consistency, and integrity. Proper planning and execution of data migration are essential to prevent data loss or corruption during the schema modification process.
Common Challenges in Implementing LLM Schema
While LLM Schema offers numerous benefits, its implementation can sometimes pose challenges that organizations must be prepared to overcome.
Overcoming Complexity in LLM Schema
LLM Schema can become complex and intricate, especially when dealing with large and diverse datasets. It requires careful planning and meticulous design to ensure that the schema adequately represents the data and meets the organizational requirements. Overcoming complexity requires skilled professionals and robust tools that simplify the schema creation and maintenance processes.
One way to address complexity in LLM Schema is through the use of data modeling techniques such as entity-relationship diagrams and normalization. These tools help in visualizing the relationships between different data entities and ensure that the schema is structured in a way that minimizes redundancy and improves data integrity. By breaking down the data into smaller, manageable components, organizations can navigate the complexities of LLM Schema more effectively.
Addressing Performance Issues in LLM Schema
In some cases, using LLM Schema for data structuring may impact system performance, especially when dealing with massive datasets or complex relationships. Organizations must optimize their LLM Schema implementation to strike a balance between data structure and system performance. This requires efficient indexing, caching mechanisms, and performance tuning techniques to ensure optimal data retrieval and manipulation.
Another approach to improving performance in LLM Schema is through the use of parallel processing and distributed computing. By distributing the workload across multiple nodes or servers, organizations can enhance the scalability and speed of data processing. This approach is particularly useful for handling real-time data streams or conducting complex analytical queries on large datasets. Implementing parallel processing techniques can significantly boost the performance of LLM Schema systems and enable organizations to harness the full potential of their data.
Future Trends in LLM Schema and Data Structuring
Looking ahead, several developments in LLM Schema and data structuring are likely to shape the future of data management.
Predicted Developments in LLM Schema
LLM Schema is expected to evolve further, incorporating advanced features and capabilities to handle complex data requirements. This includes support for semantic data modeling, machine learning algorithms, and natural language processing techniques. These advancements will enable organizations to extract valuable insights and unlock the potential of their data assets.
One of the key predicted developments in LLM Schema is the integration of blockchain technology. By leveraging the decentralized and immutable nature of blockchain, LLM Schema can enhance data security and integrity. This integration will provide organizations with a tamper-proof and transparent data management solution, ensuring the trustworthiness and reliability of their data.
The Role of LLM Schema in Future Data Management
As the volume and complexity of data continue to increase, LLM Schema will play an even more significant role in data management. It will serve as the foundation for data governance, enabling organizations to establish clear guidelines for data storage, access, and usage. LLM Schema will ensure data integrity, promote data sharing, and support interoperability, allowing organizations to harness the power of data effectively.
In addition to its foundational role in data governance, LLM Schema will also become a crucial tool in data privacy and compliance. With the rise of privacy regulations such as the General Data Protection Regulation (GDPR) and the California Consumer Privacy Act (CCPA), organizations will need to implement robust data management practices. LLM Schema can help organizations classify and categorize sensitive data, ensuring compliance with privacy regulations and protecting the rights of individuals.
In conclusion, understanding LLM Schema for data structuring is vital for organizations aiming to achieve data consistency, integration, and efficient data management. By defining the structure and organization of data elements, LLM Schema forms the backbone of data interoperability and enables seamless information exchange. Despite the challenges, LLM Schema offers immense benefits, and as the industry continues to evolve, it will play a central role in shaping the future of data structuring and management.
As you navigate the complexities of LLM Schema and strive for excellence in data management, CastorDoc stands ready to elevate your organization's capabilities. Embrace the future with CastorDoc, the most reliable AI Agent for Analytics, and transform the way your business teams interact with data. Experience the power of self-service analytics and make strategic decisions with confidence. Try CastorDoc today and unlock the full potential of your data stack, ensuring your data governance is robust and your insights are actionable. Your journey towards data mastery begins here.
You might also like
Get in Touch to Learn More
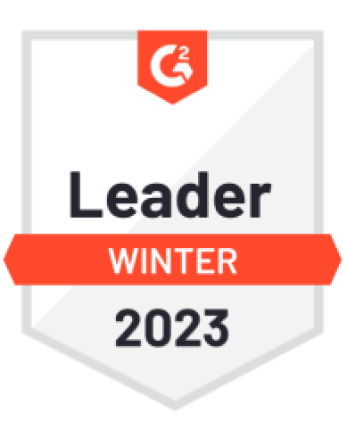
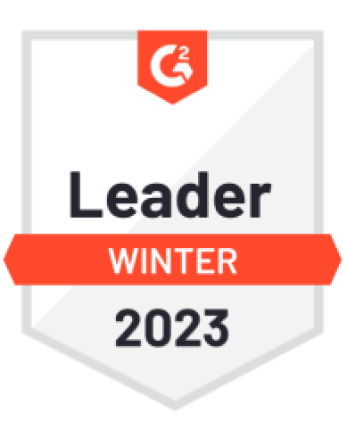

“[I like] The easy to use interface and the speed of finding the relevant assets that you're looking for in your database. I also really enjoy the score given to each table, [which] lets you prioritize the results of your queries by how often certain data is used.” - Michal P., Head of Data