Unlocking Big Data Analytics with Google BigQuery: A Comprehensive Guide to Google's Scalable Data Warehouse
Learn how to harness the power of Google BigQuery with this comprehensive guide to unlocking big data analytics.
In today's digital age, data is being generated at an unprecedented rate. From online purchases to social media interactions, every action we take leaves behind a digital footprint. However, the sheer volume and complexity of this data often poses a challenge when it comes to extracting valuable insights. This is where big data analytics comes into play.
Understanding Big Data Analytics
In a nutshell, big data analytics refers to the process of examining large and varied datasets to uncover hidden patterns, unknown correlations, market trends, customer preferences, and other useful information that can aid in making informed business decisions. It involves the use of sophisticated algorithms and tools to analyze, interpret, and visualize data, transforming it into actionable insights.
But what exactly makes big data analytics so important in today's business landscape? Let's explore some of the key reasons why organizations are increasingly turning to big data analytics to gain a competitive edge.
The Importance of Big Data Analytics
Big data analytics offers businesses a multitude of benefits. By harnessing the power of big data, organizations can gain a competitive edge, drive innovation, and improve operational efficiency. Let's take a closer look at some of the specific advantages:
- Gain valuable insights: Big data analytics allows businesses to uncover valuable insights and trends that may have otherwise gone unnoticed. These insights can help in identifying customer preferences, market trends, and patterns, allowing organizations to make data-driven decisions.
- Improve decision-making: With accurate and timely data insights, businesses can make informed decisions, reduce risks, and predict future outcomes more effectively. This can lead to improved business outcomes and increased profitability.
- Enhance customer experience: By understanding customer behavior and preferences, organizations can personalize their offerings, tailor marketing strategies, and deliver a seamless customer experience. This can foster customer loyalty and drive business growth.
- Optimize operations: Big data analytics can help businesses optimize operations by identifying bottlenecks, streamlining processes, and improving overall efficiency. This can lead to cost savings and increased productivity.
Now that we understand the importance of big data analytics, let's delve into some key concepts that form the foundation of this field.
Key Concepts in Big Data Analytics
Before diving into Google BigQuery, it's important to familiarize yourself with some key concepts in big data analytics:
- Data processing: This involves collecting, storing, and processing large volumes of data, often in real-time. Various tools and technologies, such as Hadoop and Spark, are used for efficient data processing.
- Data mining: Data mining refers to the process of discovering patterns and relationships within datasets. It involves applying statistical techniques and machine learning algorithms to extract valuable information.
- Data visualization: Data visualization is the graphical representation of data. It helps in understanding complex datasets and communicating insights effectively through charts, graphs, and other visual elements.
- Predictive analytics: Predictive analytics uses historical data and statistical modeling techniques to forecast future outcomes. By identifying patterns and trends in data, businesses can make predictions and take proactive measures.
By understanding these key concepts, you'll be better equipped to explore the world of big data analytics and leverage its potential to drive business success.
Introduction to Google BigQuery
Now that we have a solid understanding of big data analytics, let's delve into Google BigQuery - a powerful cloud-based data warehouse developed by Google. BigQuery allows businesses to store, analyze, and query massive datasets effortlessly, without the need for complex infrastructure and maintenance.
What is Google BigQuery?
Google BigQuery is a serverless, highly-scalable, and fully-managed data warehouse that enables organizations to process and analyze massive amounts of data quickly. It provides an infrastructure that allows you to focus on insights rather than worrying about server provisioning, optimization, and management.
Features and Benefits of Google BigQuery
BigQuery offers a wide range of features and benefits, making it a popular choice among businesses for their big data analytics needs:
- Scalability: With BigQuery, you can scale your data warehouse seamlessly as your data grows. It can handle terabytes and even petabytes of data without compromising on performance.
- Real-time analytics: BigQuery supports real-time analytics, allowing you to analyze streaming data and uncover insights instantly.
- Serverless architecture: BigQuery's serverless architecture eliminates the need to manage and scale infrastructure. You can focus on analyzing your data rather than spending time on maintenance and optimization.
- Cost-effective: BigQuery offers a pay-as-you-go pricing model, ensuring that you only pay for the storage and processing resources you use.
- Security and compliance: Google takes data security seriously, and BigQuery is built with robust security features to protect your data. It also complies with various industry certifications and regulations.
One of the key advantages of Google BigQuery is its integration with other Google Cloud services. This allows you to easily combine BigQuery with tools like Google Data Studio for data visualization, Google Cloud Machine Learning Engine for predictive analytics, and Google Cloud Pub/Sub for real-time data ingestion. By leveraging the power of these services together, you can create a comprehensive data analytics ecosystem that meets your organization's specific needs.
Furthermore, BigQuery provides a rich set of SQL-like functions and operators that enable you to perform complex data transformations and aggregations. Whether you need to join multiple tables, calculate statistical measures, or apply machine learning algorithms, BigQuery's SQL capabilities make it easy to manipulate and analyze your data.
In addition to its technical capabilities, Google BigQuery also offers extensive documentation, tutorials, and community support. This ensures that you have the resources and guidance you need to get started with BigQuery and make the most out of its features. The active community of developers and data analysts who use BigQuery regularly share their knowledge and best practices, creating a collaborative environment for learning and problem-solving.
The Architecture of Google BigQuery
Understanding the underlying architecture of Google BigQuery is crucial for utilizing it effectively. Let's take a closer look at the different components and how they work together to provide a scalable and efficient data warehousing solution.
Google BigQuery's architecture is designed to handle massive datasets and complex queries with ease. One key component of its architecture is the separation of storage and compute, which allows for independent scaling of each. This means that users can scale their processing power based on their needs without affecting the storage capacity, providing flexibility and cost-effectiveness.
Understanding the Infrastructure
BigQuery leverages Google Cloud Platform's infrastructure to handle the heavy lifting. It utilizes distributed computing techniques to parallelize data processing, allowing for speedy queries and analysis. By spreading the workload across multiple nodes, BigQuery can process vast amounts of data in a matter of seconds.
Additionally, Google BigQuery is built on Google's secure and reliable global network, ensuring high availability and data durability. This network infrastructure enables seamless data transfer and processing across regions, making it ideal for organizations with a global footprint.
Data Storage and Processing in BigQuery
Data storage in BigQuery is handled using a columnar storage format. This allows for efficient compression and optimized query performance. When it comes to data processing, BigQuery employs a distributed query engine called Dremel. The power of Dremel lies in its ability to execute complex queries across distributed data sources, making it the backbone of BigQuery.
Moreover, BigQuery's architecture is designed to support real-time data streaming, enabling users to ingest and analyze data in a continuous manner. This real-time processing capability is crucial for applications requiring up-to-date insights and analytics, making BigQuery a versatile solution for various use cases.
Getting Started with Google BigQuery
Now that we have a clear understanding of what Google BigQuery is and how it works, let's dive into getting started with this powerful tool. This section will walk you through the initial setup process and introduce you to the BigQuery user interface.
Setting Up Google BigQuery
Before you can start using BigQuery, you need to set up a Google Cloud project and enable the BigQuery API. Once your project is set up, you can create a BigQuery dataset, which serves as a container for your tables and views. You also need to grant appropriate permissions to users and groups to access and work with your BigQuery resources.
Navigating the Google BigQuery Interface
Once you're set up, you can start exploring the Google BigQuery interface. The interface provides a user-friendly and intuitive environment for managing your data and executing queries. From the console, you can create tables, load data, perform queries, and visualize results. Familiarizing yourself with the various features and options in the BigQuery interface will help you leverage its full potential.
Data Management in Google BigQuery
One of the key aspects of any data warehousing solution is effective data management. In this section, we'll discuss how to load data into BigQuery and query it to extract valuable insights.
Loading Data into BigQuery
BigQuery supports various methods of loading data, allowing you to choose the one that best suits your needs. You can load data from Google Cloud Storage, from a local file, or directly from other Google services such as Google Sheets or Google Cloud Pub/Sub. BigQuery also provides options for loading data incrementally or in batch mode, depending on your requirements.
Querying Data in BigQuery
Once your data is loaded into BigQuery, you can start performing queries to extract valuable insights. BigQuery supports a powerful yet easy-to-use SQL-like query language, allowing you to filter, aggregate, and transform your data. You can also join multiple tables, create views, and utilize advanced analytical functions to dig deeper into your data.
In conclusion, Google BigQuery is a game-changer when it comes to unlocking the power of big data analytics. With its scalability, ease of use, and powerful features, businesses can analyze vast amounts of data, gain meaningful insights, and make data-driven decisions. By harnessing the potential of Google BigQuery, organizations can stay ahead of the competition and drive business growth in today's data-driven world.
Ready to elevate your data analytics journey and transform your business intelligence? CastorDoc is here to empower your team with the most reliable AI Agent for Analytics. Experience the power of self-service analytics and gain trustworthy, instantaneous data answers to your strategic challenges. With CastorDoc, you can maximize the ROI of your data stack, break down data literacy barriers, and give your business users the autonomy and trust they need to make informed decisions. Don't let the complexities of data overwhelm your team. Try CastorDoc today and start unlocking the full potential of tools like Google BigQuery, making every data interaction meaningful.
You might also like
Get in Touch to Learn More
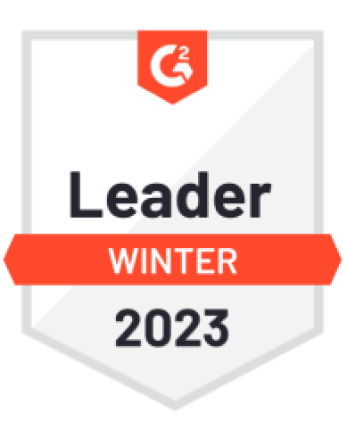
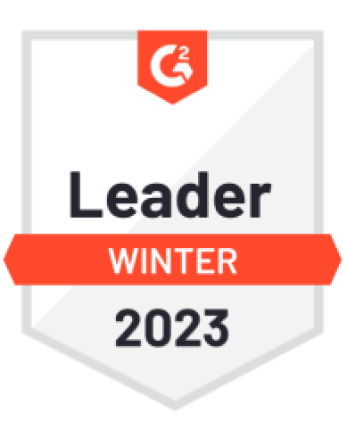

“[I like] The easy to use interface and the speed of finding the relevant assets that you're looking for in your database. I also really enjoy the score given to each table, [which] lets you prioritize the results of your queries by how often certain data is used.” - Michal P., Head of Data