A Deep Dive into the Data Value Curve: Stages and Templates
Uncover the intricacies of the Data Value Curve as this comprehensive article delves into its various stages and provides insightful templates.

In the era of big data, organizations are constantly seeking ways to extract value from the massive amounts of information they collect. One concept that has gained significant attention is the Data Value Curve. This article explores the stages and templates involved in understanding and implementing the Data Value Curve, highlighting its importance in today's business landscape.
Understanding the Concept of Data Value Curve
Data value refers to the degree to which data can contribute to achieving business objectives. The Data Value Curve framework provides a structured approach to maximize the value of data over time. By visualizing the relationship between data maturity and value creation, organizations can better assess their data assets and investments.
Delving deeper into the realm of data value, it becomes evident that the concept extends beyond mere numbers and statistics. Data value encompasses the potential for strategic insights, predictive analytics, and transformative opportunities within an organization. As businesses navigate the digital landscape, harnessing the full potential of data becomes a cornerstone for sustainable growth and competitive advantage.
The Importance of Data Value in Business
Data is the lifeblood of modern businesses. The ability to transform raw data into actionable insights can drive innovation, optimize operations, and enhance decision-making. Understanding the value that can be derived from data helps organizations prioritize investments, allocate resources effectively, and gain a competitive edge.
Moreover, in an era where data privacy and security are paramount, the value of data extends to its integrity and trustworthiness. Building a data-driven culture that upholds ethical standards and regulatory compliance is essential for fostering customer trust and maintaining organizational credibility.
Key Components of the Data Value Curve
The Data Value Curve comprises several key components that form the foundation for data-driven decision-making. These components include data collection, storage, processing, analysis, visualization, and decision-making. Each stage plays a crucial role in unlocking the value of data.
Furthermore, the iterative nature of the Data Value Curve underscores the importance of continuous improvement and adaptation. As data sources evolve, technologies advance, and business needs change, organizations must recalibrate their data strategies to stay ahead of the curve. Embracing agility and innovation in data management is key to sustaining long-term value creation and relevance in a dynamic market landscape.
Exploring the Stages of the Data Value Curve
Implementing the Data Value Curve involves progressing through various stages. Let's delve into each stage to understand their significance and potential impact on business outcomes.
The journey through the stages of the Data Value Curve is not just a linear progression but a strategic evolution that can revolutionize how organizations leverage their data assets. Each stage builds upon the foundation laid by the previous one, creating a comprehensive framework for data-driven decision-making and innovation.
Initial Stage: Data Collection and Storage
In the initial stage, organizations focus on collecting and storing vast amounts of data. This involves establishing robust data infrastructure, architecting data lakes or warehouses, and implementing data governance practices. Ensuring data quality, security, and compliance are critical considerations in this stage.
Furthermore, in this stage, organizations also lay the groundwork for future data utilization by implementing data cataloging mechanisms and metadata management processes. These practices not only streamline data discovery and accessibility but also enhance data lineage tracking and governance, ensuring the integrity and reliability of the data assets.
Intermediate Stage: Data Processing and Analysis
Once the data has been collected and stored, the next stage involves processing and analyzing the data. This entails cleaning, transforming, and structuring the data for analysis. Advanced analytics techniques, such as machine learning and artificial intelligence, are leveraged to derive insights, identify patterns, and make predictions from the data.
Moreover, in this stage, organizations focus on building scalable data pipelines and implementing automation processes to expedite data processing and analysis. By harnessing the power of cloud computing and distributed computing frameworks, such as Apache Spark, organizations can efficiently handle large volumes of data and perform complex analytics tasks in near real-time.
Advanced Stage: Data Visualization and Decision Making
With data processed and analyzed, the advanced stage focuses on presenting the insights in a meaningful and visually appealing manner. Data visualization tools and techniques come into play, allowing decision-makers to gain a holistic view of the data and make informed decisions. Interactive dashboards, graphs, and charts facilitate the exploration and understanding of complex data sets.
Furthermore, in this stage, organizations also emphasize the democratization of data and insights by implementing self-service BI tools and fostering a data-driven culture across the organization. By empowering business users with the ability to explore data independently and derive actionable insights, organizations can accelerate decision-making processes and drive innovation at all levels.
The Role of Templates in the Data Value Curve
Templates play a crucial role in simplifying and enhancing the implementation of the Data Value Curve. They provide predefined frameworks and structures to expedite data collection, analysis, and visualization. Let's explore the significance of templates in each stage of the Data Value Curve.
Templates not only serve as efficient tools for data management but also contribute significantly to the overall scalability and agility of an organization's data processes. By standardizing data collection methods and analysis techniques, templates establish a common language and framework that promotes collaboration and consistency across different departments and teams. This standardization not only enhances data quality but also fosters a culture of data-driven decision-making within the organization.
Simplifying Data Analysis with Templates
Data analysis templates provide prebuilt formulas, algorithms, and statistical models that streamline the data analysis process. By leveraging templates, organizations can accelerate insights generation and reduce the time spent on repetitive analysis tasks. These templates act as a starting point for customization based on specific data and business requirements.
Moreover, data analysis templates can also assist in ensuring compliance with industry regulations and best practices by embedding predefined compliance checks and validation mechanisms. This not only saves time but also mitigates the risk of errors and inaccuracies in the analysis process, ultimately leading to more reliable and trustworthy insights.
Enhancing Data Visualization through Templates
Data visualization templates offer preconfigured visual elements, layouts, and color schemes that enable the presentation of data in a visually appealing manner. These templates ensure consistency and coherence in visual representations, making it easier for stakeholders to interpret and derive value from the data. Templates also facilitate rapid creation of meaningful visualizations, saving time and effort.
Furthermore, data visualization templates can play a crucial role in promoting data literacy within an organization by providing intuitive and user-friendly visualization options that cater to a wide range of stakeholders with varying levels of technical expertise. This democratization of data through visually engaging templates empowers decision-makers at all levels to extract valuable insights and make informed choices based on data-driven evidence.
Challenges in Implementing the Data Value Curve
While the Data Value Curve presents a promising framework for data-driven success, its implementation is not without challenges. Organizations must be aware of and address the following challenges to maximize the benefits of the Data Value Curve.
One of the key challenges in implementing the Data Value Curve is the need to establish a data-driven culture within the organization. This involves not only technological investments but also a shift in mindset and behavior towards data utilization. Leaders need to promote data literacy among employees, encourage data-driven decision-making, and foster a culture of continuous learning and improvement based on data insights.
Overcoming Data Quality Issues
Poor data quality can significantly impact the accuracy and reliability of insights derived from the data. Organizations need to invest in data cleansing, data validation, and data quality management processes to ensure the integrity and trustworthiness of their data. By addressing data quality issues, organizations can increase the value of their data and improve decision-making.
Furthermore, organizations need to establish clear data governance frameworks to define roles, responsibilities, and processes related to data management. Data governance helps in standardizing data practices, ensuring compliance with regulations, and promoting data integrity across the organization. It also aids in resolving data ownership issues and establishing accountability for data-related decisions and outcomes.
Addressing Data Security Concerns
The increasing volume and variety of data being collected have heightened concerns regarding data security. Organizations must implement robust data security measures, including encryption, access controls, and privacy protocols, to safeguard sensitive data. By ensuring data security, organizations can instill confidence in stakeholders and mitigate the risks associated with data breaches.
In addition to technical safeguards, organizations need to focus on creating a culture of data security awareness among employees. Training programs, regular security audits, and incident response drills can help in building a proactive approach towards data security. By involving employees in data security initiatives, organizations can enhance overall data protection measures and reduce vulnerabilities arising from human error or negligence.
Future Trends in Data Value Curve
The Data Value Curve is an evolving framework that continues to be shaped by emerging trends and technologies. Two prominent trends that hold immense potential in shaping the future of the Data Value Curve are artificial intelligence and big data.
The Impact of Artificial Intelligence on Data Value
Artificial intelligence, with its ability to analyze and learn from large volumes of data, holds the key to unlocking hidden insights and patterns. By leveraging AI-powered algorithms, organizations can enhance data analysis, optimize processes, and drive innovation. As AI continues to advance, its potential to maximize data value will continue to grow.
The Role of Big Data in Shaping the Data Value Curve
Big data, characterized by high volume, velocity, and variety, has revolutionized the way organizations leverage data for decision-making. Big data technologies and platforms enable organizations to capture, store, process, and analyze vast amounts of data in real-time. This accelerates the progression through the Data Value Curve, enabling rapid insights generation and informed decision-making.
As organizations continue to navigate the complexities of the data-driven landscape, understanding and implementing the Data Value Curve becomes increasingly crucial. By embracing the stages and leveraging templates, organizations can unlock the full potential of their data and drive transformative business outcomes.
You might also like
Get in Touch to Learn More
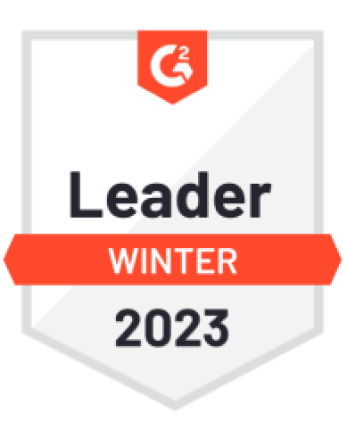
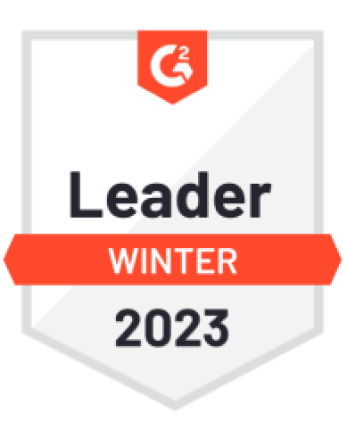

“[I like] The easy to use interface and the speed of finding the relevant assets that you're looking for in your database. I also really enjoy the score given to each table, [which] lets you prioritize the results of your queries by how often certain data is used.” - Michal P., Head of Data