AI analytics explained: How it works and key industry use cases
Discover the transformative power of AI analytics in this guide.

Understanding the Basics of AI Analytics
AI analytics combines artificial intelligence (AI) and data analytics to derive insights from large and complex data sets. It leverages machine learning algorithms, natural language processing, and data processing techniques to analyze data and provide actionable insights that can significantly enhance decision-making processes.
At its core, AI analytics helps organizations uncover hidden patterns, trends, and correlations within their data. Unlike traditional analytics methods, which often rely on manual inputs and pre-defined models, AI analytics can adapt and learn from new data in real time, enabling organizations to stay agile and responsive in their strategies.
Defining AI Analytics
AI analytics refers to the integration of artificial intelligence technologies with analytical processes to automate, augment, and optimize data analysis. It encompasses a variety of applications, including predictive analytics, natural language processing, and automated data visualization.
By combining these technologies, AI analytics can sift through vast amounts of data efficiently, identify significant patterns, and provide forecasts that empower businesses to make more informed decisions. The use of AI in analytics revolutionizes traditional data-analysis methodologies, allowing for a deeper understanding of customer behavior, market trends, and operational efficiencies.
The Mechanics of AI Analytics
The mechanics of AI analytics involve several key processes. Initially, data is collected from various sources, such as databases, sensors, and online platforms. This raw data is then cleaned and pre-processed to remove inconsistencies and prepare it for analysis.
Machine learning algorithms play a critical role in AI analytics. They are trained on historical data to recognize patterns and predict future outcomes. As new data becomes available, these algorithms continuously learn and refine their models, ensuring ongoing accuracy in predictions and insights.
Furthermore, AI analytics often employs advanced techniques such as deep learning, which mimics the human brain's neural networks to process complex data structures. This allows for more sophisticated analysis, particularly in areas like image and speech recognition. For instance, businesses can analyze customer feedback through sentiment analysis, gaining insights into public perception and enhancing their marketing strategies accordingly.
Another vital component of AI analytics is data visualization, which transforms complex analytical results into intuitive graphical representations. This not only aids in understanding the data but also facilitates communication across teams, enabling stakeholders to grasp insights quickly and make collaborative decisions. As a result, organizations can harness the power of AI analytics not just for operational efficiency but also for fostering innovation and competitive advantage in their respective markets.
The Role of AI in Data Analysis
AI is reshaping the landscape of data analysis by introducing capabilities that were previously unattainable with conventional statistical methods. It enhances the analytical process through automation, scalability, and real-time insights.
Through the application of AI, organizations can perform complex analyses that would take human analysts significantly longer to execute. This increased efficiency opens doors to new opportunities in understanding market dynamics and consumer behavior, aligning business strategies with real-time data insights. Moreover, AI's ability to handle unstructured data, such as text and images, allows companies to tap into previously overlooked information sources, enriching their analytical capabilities and driving more informed decision-making.
AI in Predictive Analysis
Predictive analysis uses historical data and AI algorithms to forecast future events. In sectors such as finance, it helps in risk assessment by predicting potential defaults on loans. In marketing, predictive analytics can enhance customer targeting by identifying likely purchasers based on previous purchasing behaviors.
Machine learning models are essential in this process, as they allow the predictive systems to learn from existing data patterns continuously. As these models improve over time, predictions become more accurate, providing businesses with a competitive edge. Furthermore, the integration of AI with big data technologies enables organizations to analyze vast amounts of information at unprecedented speeds, allowing for more nuanced and timely predictions that can adapt to changing market conditions.
AI in Descriptive Analysis
Descriptive analysis focuses on understanding past data to identify trends and patterns. AI technologies can process vast datasets more effectively than traditional methods, helping organizations to summarize historical data in real-time.
For example, in customer service, AI can analyze interactions and feedback to determine overall satisfaction levels, identifying key areas needing improvement. This descriptive ability empowers organizations to implement changes based on data-driven insights. Additionally, AI can automate the generation of reports and visualizations, making it easier for stakeholders to grasp complex data narratives quickly. By transforming raw data into intuitive dashboards, AI not only enhances comprehension but also fosters a culture of data literacy across the organization, enabling teams to make more informed decisions based on comprehensive insights.
Key Components of AI Analytics
Several critical components enable AI analytics to function efficiently. These technologies and methodologies are at the heart of what makes AI analytics a robust approach to understanding data. The integration of these components allows organizations to harness the full potential of their data, transforming raw information into actionable insights that can drive decision-making processes.
Machine Learning in AI Analytics
Machine learning (ML) is a subset of AI that involves training algorithms on datasets to enable them to learn and make predictions. In AI analytics, ML algorithms are essential for processing data, discovering patterns, and making forecasts. The iterative nature of machine learning allows models to improve over time as they are exposed to more data, making them increasingly accurate and reliable.
Various algorithms—including decision trees, neural networks, and clustering techniques—can be employed depending on the nature of the data and the specific analytical requirements. The choice of algorithm directly influences the quality and accuracy of the insights generated. For instance, neural networks are particularly effective for complex datasets with non-linear relationships, while decision trees offer a more interpretable approach for straightforward classification tasks. Additionally, the implementation of ensemble methods, which combine multiple algorithms, can further enhance predictive performance by leveraging the strengths of different models.
Natural Language Processing in AI Analytics
Natural language processing (NLP) is another vital component of AI analytics. It allows machines to understand and interpret human language. This capability is crucial for sentiment analysis and gaining insights from unstructured data, such as customer reviews and social media interactions. By employing NLP techniques, organizations can tap into vast amounts of textual data that would otherwise remain untapped, providing a richer context for decision-making.
NLP algorithms can analyze text data to extract meaning, identify relationships, and categorize information, enabling organizations to leverage insights from conversations or written feedback effectively. This deep analysis leads to more informed business strategies and improved customer engagement. Furthermore, advancements in NLP, such as transformer models and contextual embeddings, have significantly enhanced the ability to understand nuances in language, including sarcasm and sentiment shifts, allowing for more sophisticated interpretations of customer feedback. As a result, businesses can tailor their products and services to better meet the evolving needs of their customers, fostering loyalty and satisfaction in an increasingly competitive marketplace.
The Impact of AI Analytics on Various Industries
AI analytics is not confined to a single sector; its influence can be seen across various industries. Businesses are adopting AI-driven analytics to enhance their operations and achieve strategic goals.
AI Analytics in Healthcare
In healthcare, AI analytics helps in patient care by predicting disease outbreaks, improving diagnosis accuracy, and optimizing treatment plans. By analyzing patient data and medical histories, healthcare providers can identify patterns that support more personalized and effective treatments.
Additionally, AI analytics enables hospitals to streamline operations by predicting patient admissions, thereby optimizing resource allocation and improving service delivery.
AI Analytics in Finance
Financial institutions employ AI analytics to enhance fraud detection and risk management. By analyzing transaction patterns, AI systems can quickly identify suspicious activities that may indicate fraud, allowing for real-time responses.
Moreover, AI analytics aids in investment decision-making by analyzing market trends, economic indicators, and historical performance, enabling investors to make data-driven choices.
AI Analytics in Retail
In the retail industry, AI analytics provides insights into customer preferences and inventory management. By analyzing sales data and customer interactions, retailers can tailor their offerings to meet market demands, optimizing both inventory levels and customer satisfaction.
Through predictive analytics, retailers can forecast trends, set pricing strategies, and improve marketing campaigns, leading to increased sales and customer loyalty.
Future Trends in AI Analytics
The future of AI analytics holds significant promise as advancements in technology continue. Organizations are increasingly recognizing the value of integrating AI analytics into their operations as tools grow more sophisticated and intuitive.
As data continues to proliferate, the capacity for AI-driven analytics to process and make sense of this information will become indispensable for maintaining competitive advantage.
The Growing Importance of AI Analytics
The growing importance of AI analytics is reflected in its widespread adoption across industries. Organizations that leverage AI analytics efficiently can transform vast amounts of data into valuable insights, guiding strategic decisions and enhancing operational efficiency.
Furthermore, as companies strive to adapt to changing market conditions, the ability to predict future trends becomes increasingly crucial, solidifying AI analytics as a foundational resource for any progressive organization.
Potential Challenges and Solutions in AI Analytics
Despite its advantages, AI analytics also presents challenges, such as data privacy concerns, the need for skilled personnel, and algorithmic bias. Organizations must navigate these challenges strategically to maximize the benefits of AI analytics.
Implementing robust data governance frameworks, investing in employee training, and focusing on ethical AI development are some ways to address these issues. By tackling potential pitfalls head-on, organizations can unlock the full potential of AI analytics in driving business success.
As we've explored the transformative power of AI analytics and its capacity to reshape industries, the importance of robust data governance and user-friendly analytics tools cannot be overstated. CastorDoc stands at the forefront of this revolution, offering an advanced governance platform with an intuitive AI assistant designed to empower your business with self-service analytics. Whether you're looking to streamline your data governance lifecycle, ensure compliance, or enable business users to harness data with confidence, CastorDoc is your partner in navigating the data-driven future. Don't let the complexities of AI analytics hold you back. Try CastorDoc today and unlock the full potential of your data to drive informed decision-making across your enterprise.
You might also like
Get in Touch to Learn More
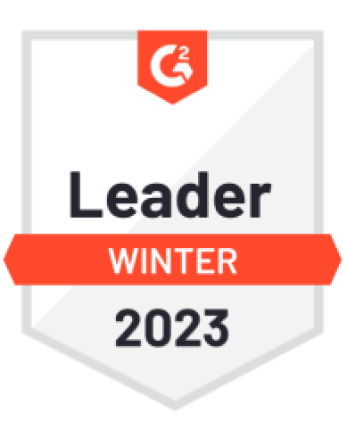
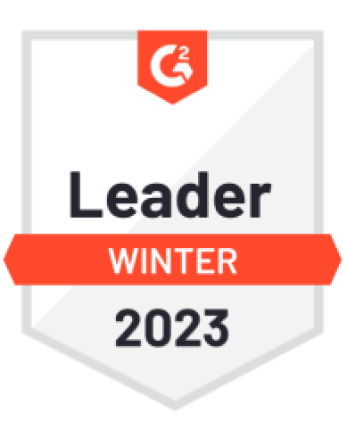

“[I like] The easy to use interface and the speed of finding the relevant assets that you're looking for in your database. I also really enjoy the score given to each table, [which] lets you prioritize the results of your queries by how often certain data is used.” - Michal P., Head of Data