Business Semantic Layer: Understanding the Foundation of Modern Data Analytics
Unlock the power of modern data analytics with a deep dive into the Business Semantic Layer.

Understanding the intricacies of data analytics isn't merely about employing advanced algorithms or powerful computing resources. At the heart of efficient data utilization lies the Business Semantic Layer (BSL), a crucial element that serves as a bridge between raw data and actionable insights. This article delves into the foundational aspects of the BSL, its significance in modern analytics, and what the future holds for this technology.
Defining Business Semantic Layer
The Business Semantic Layer refers to an abstraction layer that sits between the raw data sources and the end-user analytics tools. Its primary purpose is to convert complex data structures into more user-friendly formats, making it easier for business users to access and interpret data without needing deep technical expertise. It encapsulates business terms, definitions, and relationships to ensure that end-users have a coherent and meaningful way to interact with data.
Beneath this layer, there exist numerous data sources, databases, and data warehouses that house vast amounts of information. The BSL acts as a translator, simplifying these diverse data formats into a consistent framework that can be easily understood by analysts, decision-makers, and executives within an organization. By providing this intermediary layer, organizations can significantly reduce the time and effort required to derive actionable insights from their data, ultimately fostering a more data-driven culture.
Furthermore, the Business Semantic Layer often incorporates metadata management, which enriches the data with context and additional information. This includes details about data lineage, quality, and usage patterns, allowing users to make informed decisions about the reliability and relevance of the data they are analyzing. By enhancing the understanding of data provenance, organizations can build trust in their analytics processes and ensure that strategic decisions are based on accurate and timely information.
The Role of Business Semantic Layer in Data Analytics
The significance of the Business Semantic Layer cannot be overstated. It plays a pivotal role in creating a unified view of an organization’s data. By establishing clear definitions and meanings for various data artifacts, it improves collaboration among business units and helps to eliminate discrepancies that can arise from differing interpretations of data. This is particularly crucial in large organizations where various departments may have their own data silos, leading to inconsistencies and confusion.
Moreover, this layer enhances data discovery and exploration by providing intuitive access to data. Users are empowered to generate reports and dashboards, exploring data trends and making data-driven decisions without needing extensive training or technical prowess in data warehousing or data mining techniques. The result is a more agile organization where insights can be rapidly gleaned, allowing for quicker responses to market changes and customer needs. As a result, the Business Semantic Layer not only democratizes data access but also fosters a culture of continuous improvement and innovation within the organization.
Key Components of a Business Semantic Layer
A well-structured Business Semantic Layer comprises several critical components that together foster effective data management and utilization. These components include:
- Data Models: These describe the entities and relationships within the data, creating a clear framework for how data should be interpreted and utilized.
- Business Glossary: This provides definitions for terms, ensuring all stakeholders share a common understanding of key concepts and metrics.
- Access Controls: These are vital for ensuring that the right users have access to the right data, maintaining security and compliance.
- Data Transformation Rules: These rules dictate how raw data is transformed into meaningful insights, outlining the processes that govern data extraction, calculation, and presentation.
In addition to these components, the Business Semantic Layer often includes visualization tools that facilitate the representation of data in a more digestible format. These tools can help users identify patterns, trends, and anomalies in the data, making it easier to draw conclusions and make informed decisions. Furthermore, the integration of advanced analytics capabilities, such as predictive modeling and machine learning, can enhance the insights derived from the data, allowing organizations to not only understand past performance but also anticipate future trends and behaviors.
Another important aspect of the Business Semantic Layer is its ability to support self-service analytics. By providing business users with the tools and resources they need to explore data independently, organizations can reduce the burden on IT departments and empower employees at all levels to engage with data. This self-service capability is essential in today’s fast-paced business environment, where timely access to information can be the difference between seizing an opportunity and missing out.
The Importance of a Semantic Layer in Business
Businesses increasingly recognize the value of implementing a robust semantic layer as part of their data strategy. It brings clarity and coherence to complex datasets, significantly enhancing operational efficiency and decision-making processes.
Enhancing Data Quality and Consistency
Data quality is a persistent challenge for organizations, as inconsistencies can lead to erroneous conclusions and poor business strategies. The Business Semantic Layer mitigates these issues by standardizing definitions and formats across different data sources. This ensures that all data used in reporting and analysis are consistent, leading to more reliable outcomes.
With standardized metrics and definitions, organizations can ensure they are accurately measuring performance and analyzing trends, thereby significantly enhancing their overall data quality. Furthermore, the semantic layer acts as a bridge between technical and non-technical users, allowing stakeholders from various departments to engage with the data without needing extensive technical expertise. This democratization of data access fosters a culture of data-driven decision-making throughout the organization, empowering employees at all levels to contribute to strategic initiatives.
Facilitating Better Decision Making
Given that informed decision-making is foundational to successful business operations, the semantic layer plays a critical role by delivering relevant data to the right individuals. Users can elucidate insights swiftly, transforming raw data into actionable recommendations.
This empowerment enables executives and managers to respond effectively to market changes and operational challenges, ultimately driving innovation and maintaining competitive advantages in their respective industries. Moreover, the semantic layer supports advanced analytics and machine learning initiatives, as it provides a structured framework for data interpretation. By enabling predictive analytics and trend forecasting, businesses can anticipate customer needs and market shifts, allowing them to proactively adjust their strategies. This forward-thinking approach not only enhances operational agility but also positions organizations to capitalize on new opportunities as they arise, ensuring sustained growth and success in an ever-evolving business landscape.
Building a Robust Business Semantic Layer
Developing an effective Business Semantic Layer involves meticulous planning and execution. Organizations must identify their needs and how the semantic layer can support their strategic goals.
Essential Steps in Creating a Semantic Layer
Creating a Business Semantic Layer involves several essential steps:
- Assessment of Current Data Sources: Evaluate and document existing data sources and ensure they align with business objectives.
- Defining Business Vocabulary: Develop a comprehensive business glossary to foster a common understanding across the organization.
- Modeling Data Relationships: Create entity-relationship diagrams to depict how different data elements relate to one another.
- Implementing Governance Policies: Establish rules and guidelines for data access and usage to ensure compliance and security.
- Continuous Review and Evolution: Regularly update the semantic layer to adapt to changing business needs and technological advancements.
Overcoming Challenges in Semantic Layer Development
While the advantages of a Business Semantic Layer are compelling, organizations often encounter challenges in its development. These can include resistance to change within business units, technical integration issues, and the ongoing maintenance required to keep the layer relevant and effective.
Successfully addressing these challenges requires strong leadership and collaboration among stakeholders at all levels. Engaging users early in the development process and providing adequate training can help ease resistance and foster a culture of data-driven decision-making across the organization.
The Future of Business Semantic Layers
As we look ahead, the future of Business Semantic Layers appears promising yet challenging. The rapid evolution of data analytics technologies and methodologies will likely continue to shape how semantic layers are developed and deployed.
Emerging Trends in Semantic Layer Technology
One noteworthy trend is the increasing integration of semantics with cloud-based analytics platforms. These platforms offer advanced capabilities for processing large datasets while providing flexible tools for users. The ease of integration with various data sources allows businesses to streamline the creation and management of semantic layers.
Another trend is the shift towards metadata-driven architectures, where the semantic layer is dynamically generated based on user interactions and queries. This real-time adaptability can lead to more accurate and relevant insights as user needs evolve.
The Impact of AI and Machine Learning on Semantic Layers
Artificial intelligence and machine learning are set to revolutionize Business Semantic Layers by automating many processes involved in data modeling and transformation. These technologies can help identify patterns, suggest data relationships, and optimize data access based on user behavior.
Ultimately, as AI and machine learning mature, they will enhance the effectiveness of semantic layers, making them more intuitive and empowering businesses to derive insights with unprecedented speed and accuracy.
In conclusion, the Business Semantic Layer is not just a technical construct but a vital framework that enables organizations to derive meaningful insights from their data. By understanding its components, importance, and future trends, businesses can harness the full potential of their data analytics capabilities.
As you've learned about the transformative power of the Business Semantic Layer in modern data analytics, it's clear that the right tools can elevate your organization's data capabilities to new heights. CastorDoc is designed to seamlessly integrate advanced governance, cataloging, and lineage capabilities with a user-friendly AI assistant, offering a comprehensive solution for enabling self-service analytics. Embrace the future of data management and experience how CastorDoc can revolutionize your data governance and analytics processes. Try CastorDoc today and empower your team to unlock the full potential of your data, driving informed decision-making and strategic success across your enterprise.
You might also like
Get in Touch to Learn More
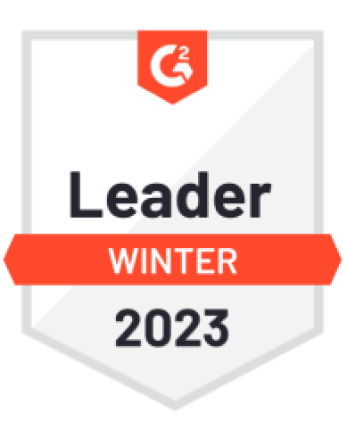
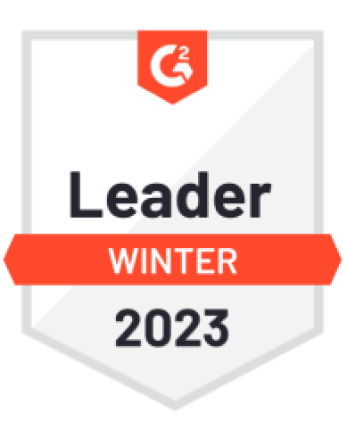

“[I like] The easy to use interface and the speed of finding the relevant assets that you're looking for in your database. I also really enjoy the score given to each table, [which] lets you prioritize the results of your queries by how often certain data is used.” - Michal P., Head of Data