Data Catalog for Data Scientists: A Comprehensive Guide
Explore the comprehensive guide to the ultimate data catalog for data scientists.
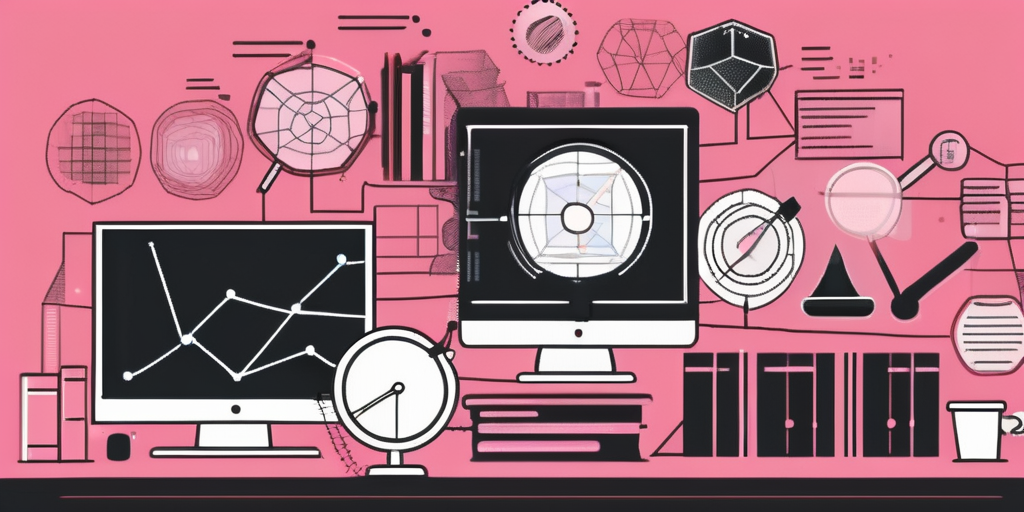
In today's data-driven world, where the sheer volume of information is overwhelming, data scientists face a unique challenge - how to efficiently manage and make sense of the vast amounts of data at their disposal. This is where a data catalog comes into play. A data catalog is an essential tool that helps data scientists organize, discover, and understand the data they work with. In this comprehensive guide, we will explore the importance of a data catalog, its key benefits, essential features, steps to create a robust catalog, how to choose the right tool, and best practices for maintaining a data catalog.
Understanding the Importance of a Data Catalog
As the field of data science continues to grow, the need for a centralized and accessible repository of data becomes paramount. A data catalog serves as a knowledge hub, enabling data scientists to easily locate and understand the data they require for their analyses. It provides a unified view of an organization's data assets, ensuring that data scientists can trust the data they are working with and make informed decisions. Without a data catalog, data scientists may spend valuable time searching for relevant datasets, leading to inefficiencies and potentially compromising the accuracy of their analyses.
The Role of a Data Catalog in Data Science
A data catalog plays a crucial role in the data science workflow. It serves as a bridge between data scientists and the vast array of data sources within an organization. By providing a comprehensive and centralized inventory of data assets, a data catalog helps data scientists identify the right data for their analysis. It facilitates data exploration and increases collaboration across teams, enabling data scientists to leverage the collective knowledge and expertise within their organization. Moreover, a data catalog promotes data governance, ensuring that data is managed, documented, and protected in a consistent manner.
Key Benefits of Using a Data Catalog
Implementing a data catalog offers numerous benefits for data scientists and organizations as a whole. Firstly, it improves productivity by reducing the time spent searching for data. With a data catalog, data scientists can quickly locate the datasets they need, freeing up valuable time for analysis and insights generation. Secondly, a data catalog enhances data quality and consistency. By providing detailed metadata and data lineage, it allows data scientists to assess the reliability and relevance of data. Additionally, a data catalog promotes collaboration and knowledge sharing, fostering a data-driven culture within the organization. Finally, a data catalog facilitates regulatory compliance by ensuring data governance and data privacy requirements are met.
Furthermore, a data catalog enables data scientists to discover hidden relationships and patterns within their data. By having a holistic view of the available data assets, data scientists can identify connections and correlations that may have otherwise gone unnoticed. This deeper understanding of the data can lead to more accurate and impactful analyses, ultimately driving better decision-making and business outcomes.
In addition, a data catalog can help streamline the onboarding process for new data scientists within an organization. By providing a centralized repository of data assets, it allows new team members to quickly familiarize themselves with the available data and understand its structure and context. This reduces the learning curve and enables data scientists to start contributing to projects more efficiently, accelerating the pace of innovation and problem-solving.
Essential Features of an Effective Data Catalog
When choosing a data catalog tool, it is crucial to consider the features that best align with your organization's requirements. Let's explore some essential features that an effective data catalog should possess:
Data Discovery and Inventory
A robust data catalog should enable data scientists to easily discover and inventory the available datasets. It should provide search functionality, allowing users to filter and find relevant data based on criteria such as data type, date, and source. Moreover, a data catalog should support automated data ingestion, ensuring that new datasets are automatically added to the catalog as they become available.
Data Classification and Metadata Management
Organizing and classifying data in a data catalog is essential for efficient data management. A data catalog should allow data scientists to tag and categorize data based on different attributes such as subject area, data source, and data format. Additionally, it should provide robust metadata management capabilities, allowing data scientists to capture and maintain detailed information about the data, including definitions, transformations, and data lineage.
Data Quality and Governance
Data quality is of utmost importance in any data-driven organization. A data catalog should include features that assess the quality of data, enabling data scientists to identify potential issues and anomalies. It should also support data governance by providing capabilities to define and enforce data standards, access controls, and data privacy policies. A comprehensive data catalog should empower organizations to meet regulatory compliance requirements and maintain data integrity.
Furthermore, an effective data catalog should offer data profiling capabilities. This means that it should be able to automatically analyze the structure, content, and relationships within the datasets. By performing data profiling, the catalog can provide insights into the quality, completeness, and accuracy of the data. This information is invaluable for data scientists who need to make informed decisions based on reliable and trustworthy data.
In addition to data profiling, a data catalog should also support data lineage tracking. This means that it should be able to trace the origin and transformation of data throughout its lifecycle. By understanding the lineage of data, data scientists can gain insights into how the data has been processed, ensuring transparency and accountability. This feature is particularly important for organizations that need to comply with data regulations and demonstrate data provenance.
Overall, an effective data catalog should not only provide basic features like data discovery and inventory, data classification and metadata management, and data quality and governance, but it should also offer advanced capabilities like data profiling and data lineage tracking. By incorporating these additional features, a data catalog becomes a powerful tool that enables data scientists to efficiently manage and utilize their data assets, ultimately driving better insights and decision-making.
Steps to Create a Robust Data Catalog
Building a robust and effective data catalog requires a thoughtful approach. Let's explore the key steps involved:
Identifying Data Sources
The first step in creating a data catalog is to identify and inventory the data sources within your organization. This includes databases, data lakes, data warehouses, APIs, and external data sources. It is crucial to have a comprehensive understanding of the various data sources to ensure that all relevant data is included in the catalog.
Cataloging and Indexing Data
Once the data sources have been identified, the next step is to catalog and index the data. This involves capturing metadata such as data descriptions, data structure, and relationships between different datasets. A well-designed data catalog should provide automated mechanisms to extract metadata from different data sources, ensuring that the catalog remains up to date as new datasets are added or existing ones are modified.
Implementing Data Security Measures
Data security is a critical aspect of any data catalog. It is essential to implement appropriate security measures to protect sensitive and confidential information. This includes defining access controls, encryption mechanisms, and data anonymization techniques. A robust data catalog should adhere to data privacy regulations and ensure that data is shared only with authorized personnel.
Choosing the Right Data Catalog Tool
Choosing the right data catalog tool is a crucial decision for any data-driven organization. Consider the following factors when evaluating different data catalog tools:
Evaluating Different Data Catalog Tools
Before making a decision, evaluate multiple data catalog tools available in the market. Consider factors such as scalability, ease of use, integration capabilities, and vendor support. Assess how well each tool aligns with your organization's specific needs and requirements.
Considerations for Selecting a Data Catalog Tool
When selecting a data catalog tool, it is important to consider factors such as data governance capabilities, support for different data formats, scalability, and extensibility. Additionally, evaluate the tool's ability to integrate with other data platforms and tools within your organization's data ecosystem.
Best Practices for Maintaining a Data Catalog
Maintaining a data catalog is an ongoing process that requires regular attention and updates. Here are some best practices to ensure the effectiveness and usability of your data catalog:
Regular Data Catalog Updates
Data is continuously evolving, and it is essential to keep your data catalog up to date. Regularly add new datasets, update metadata, and remove obsolete or redundant data. This ensures that data scientists have access to the most current and accurate information for their analyses.
Ensuring Data Catalog Usability
A data catalog should be user-friendly and intuitive for data scientists to navigate and search. Ensure that the catalog provides powerful search capabilities, filtering options, and an easy-to-understand interface. Consider incorporating user feedback and incorporating usability enhancements to optimize the data catalog's effectiveness.
Promoting Data Catalog Adoption Among Teams
To maximize the value of a data catalog, it is important to promote its adoption across teams. Educate and train data scientists on the benefits and features of the data catalog. Encourage collaboration and knowledge sharing by providing guidelines and incentives for data scientists to contribute to the catalog's growth and maintenance.
Implementing a robust data catalog is a vital step for data scientists to effectively manage, analyze, and derive insights from the vast amounts of data available today. By understanding the importance of a data catalog, choosing the right tool, and following best practices for maintenance, data scientists can harness their analytical potential and make better-informed decisions for their organizations.
Ready to elevate your data management and analytics capabilities? CastorDoc is here to transform the way you interact with your data. With its advanced governance, cataloging, and lineage features, combined with a user-friendly AI assistant, CastorDoc stands out as a powerful tool for enabling self-service analytics. Embrace the ease of natural language interactions and gain complete control over your data governance lifecycle. Don't miss the opportunity to streamline your data processes and empower your decision-making. Try CastorDoc today and unlock the full potential of your data assets.
You might also like
Get in Touch to Learn More
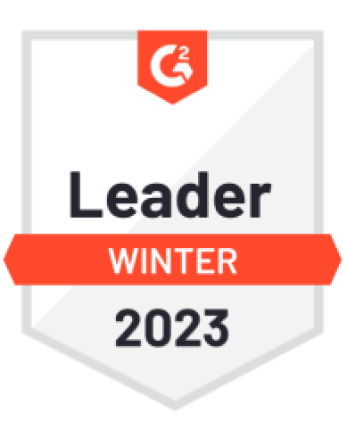
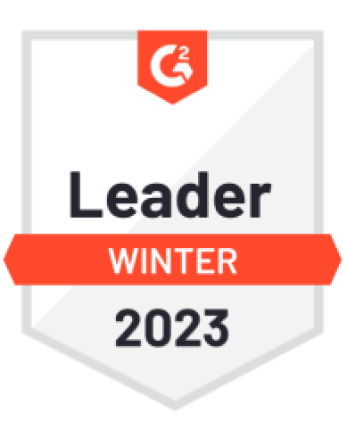

“[I like] The easy to use interface and the speed of finding the relevant assets that you're looking for in your database. I also really enjoy the score given to each table, [which] lets you prioritize the results of your queries by how often certain data is used.” - Michal P., Head of Data