Data Classification: Definition, Types, Examples, Tools & More!
Explore the world of data classification! Learn the definition, various types, real-life examples, top tools, and more to master this essential aspect of data management..

Data classification is a critical aspect of data management that involves categorizing data based on certain attributes or characteristics. By organizing data into different categories, data classification enables better data management, security, and accessibility. In this article, we will delve into the world of data classification, exploring its importance, key concepts, types, tools, and the process involved.
Understanding Data Classification
Data classification is a fundamental process that allows organizations to identify and prioritize their data, ensuring that it is handled appropriately. By understanding the underlying principles of data classification, businesses can effectively manage their data, mitigate risks, and comply with regulatory requirements.
The Importance of Data Classification
Data classification plays a vital role in data management as it enables organizations to assign appropriate protective measures to various types of data. By classifying data, businesses can identify sensitive information that requires additional security measures, ensuring that it remains confidential and protected from unauthorized access.
Moreover, data classification provides organizations with a comprehensive understanding of their data landscape. It allows them to gain insights into the types of data they possess, the potential risks associated with each type, and the necessary steps to mitigate those risks. This knowledge empowers businesses to make informed decisions about data handling and protection.
Furthermore, data classification assists in data governance by facilitating better data organization, simplifying data retrieval, and enhancing data quality. By applying consistent classification techniques, organizations can streamline their data management processes and improve overall operational efficiency.
Key Concepts in Data Classification
When embarking on data classification, there are several key concepts that organizations should be familiar with:
- Data Sensitivity: Data sensitivity refers to the level of confidentiality or criticality associated with specific data. Understanding data sensitivity is crucial in determining the appropriate classification level for different types of data. It involves evaluating factors such as the potential impact of data exposure, legal requirements, and industry standards.
- Data Usage: Data usage encompasses the purpose for which data is collected, processed, and utilized. By classifying data based on its usage, organizations can identify data that requires specific handling instructions or access restrictions. For example, personal customer information may be classified differently from marketing data, as they serve different purposes and have distinct privacy considerations.
- Data Context: Data context involves considering the context in which data is collected and used. Understanding data context helps organizations determine the significance and relevance of data, aiding in its classification process. Factors such as the source of data, its relationship to other data sets, and the intended recipients of the data can all influence its classification. This contextual understanding ensures that data is classified accurately and appropriately.
By grasping these key concepts and implementing robust data classification practices, organizations can effectively manage their data assets, protect sensitive information, and meet regulatory requirements. Data classification is not just a technical process; it is a strategic approach that empowers businesses to make informed decisions about data handling and protection.
Defining Data Classification
At its core, data classification is the process of categorizing data into distinct groups based on predetermined criteria. By applying basic principles of data classification, organizations can effectively organize their data and establish a robust data management framework.
Basic Principles of Data Classification
Effective data classification adheres to several principles:
- Consistency: Data classification should be consistent throughout the organization, ensuring that data is classified uniformly.
- Relevance: Classification criteria should align with the organization's goals, regulatory requirements, and business needs.
- Scalability: The classification system should be scalable to accommodate future growth and changes in data volumes and types.
- Clarity: The classification criteria and categories should be clearly defined and easily understood by all stakeholders.
The Role of Data Classification in Data Management
Data classification serves as the foundation for effective data management. It enables organizations to implement appropriate data retention, access controls, and security measures. By classifying data, businesses can identify data that requires special handling, ensuring compliance with regulatory mandates and minimizing the risk of data breaches.
Furthermore, data classification supports efficient data retrieval, allowing organizations to locate and retrieve pertinent information promptly. It also aids in identifying redundant or obsolete data, facilitating data cleanup initiatives and optimizing storage utilization.
However, data classification goes beyond these immediate benefits. It also plays a crucial role in data analytics and decision-making processes. By classifying data, organizations can gain insights into patterns, trends, and correlations that can inform strategic planning and operational improvements.
For example, a retail company that classifies customer data based on purchasing behavior can identify customer segments with specific preferences. This information can then be used to tailor marketing campaigns, personalize product recommendations, and optimize inventory management. By understanding the different customer segments, the company can enhance customer satisfaction and drive revenue growth.
In addition, data classification contributes to data governance efforts. It helps establish clear ownership and accountability for data assets, ensuring that the right individuals or teams are responsible for managing and protecting specific data categories. This promotes data stewardship and fosters a culture of data responsibility within the organization.
Moreover, data classification supports data sharing and collaboration. When data is classified and labeled appropriately, it becomes easier to determine which data can be shared internally or externally, and under what conditions. This facilitates collaboration between departments, business partners, and even with customers, enabling organizations to leverage their data assets more effectively.
In summary, data classification is a fundamental process that enables organizations to organize, manage, and derive value from their data. By adhering to the basic principles of data classification and recognizing its broader implications, businesses can unlock the full potential of their data and gain a competitive edge in today's data-driven world.
Different Types of Data Classification
Data classification can be categorized into various types based on different criteria. Two common types of data classification include:
Classification Based on Data Sensitivity
Data can be classified based on its sensitivity level, ranging from public to highly confidential. By assigning sensitivity labels, organizations can implement appropriate access controls, encryption, and data protection measures.
For instance, public data may include information available to the general public, such as marketing materials or press releases. This type of data is typically considered safe for open distribution and does not require stringent security measures. On the other end of the spectrum, highly confidential data may encompass intellectual property, financial records, or strategic business plans. This category of data demands the highest level of protection to prevent unauthorized access or leakage.
Classification Based on Data Usage
Data can also be classified based on its intended use or purpose. This type of classification helps organizations identify data that requires specific handling instructions or regulatory compliance.
For example, data may be classified as research data, customer data, or financial data, each requiring distinct access controls and security measures. Research data may involve experimental results, scientific findings, or academic studies that need to be safeguarded to maintain integrity and confidentiality. Customer data, including personal information, purchase history, and communication records, must adhere to strict data protection regulations to ensure consumer privacy and trust. Financial data, such as budget reports, transaction details, and payroll information, necessitates robust security measures to prevent fraud, unauthorized alterations, or data breaches.
Tools for Effective Data Classification
Several tools can assist organizations in the data classification process, ensuring accuracy, consistency, and efficiency.
Automated Data Classification Tools
Automated data classification tools employ machine learning algorithms to analyze data attributes and apply predefined classification rules. These tools streamline the classification process, enabling organizations to handle large volumes of data more effectively.
Examples of automated data classification tools include IBM InfoSphere Classification Module and Microsoft Azure Information Protection.
Manual Data Classification Tools
In certain scenarios, manual data classification may be necessary, particularly when dealing with unstructured data or unique classification requirements. Manual data classification tools allow users to manually assign classification labels and metadata to individual data items.
Examples of manual data classification tools include Microsoft Office Classification Labels and Titus Classification Suite.
The Process of Data Classification
The process of data classification typically involves several steps:
Steps Involved in Data Classification
- Identify Classification Requirements: Determine the classification criteria based on the organization's goals, regulatory requirements, and data sensitivity.
- Inventory Data: Identify and inventory all data within the organization, classifying each data item based on the predefined criteria.
- Assign Classification Labels: Assign classification labels or tags to each data item, indicating its classification level or category.
- Implement Access Controls: Implement appropriate access controls and security measures based on the data classification, ensuring that only authorized users can access sensitive data.
- Maintain and Review: Regularly review and update data classifications as the organization's needs, regulations, and data landscape evolve.
Challenges in Data Classification
Data classification can present certain challenges, including:
- Data Complexity: Classifying complex or unstructured data can be challenging as it may require additional manual effort.
- Data Volume: Handling large volumes of data can be time-consuming and resource-intensive.
- Consistency: Ensuring consistent classification criteria and labels across the organization can be a daunting task.
- Changing Data Landscape: As data types and regulatory requirements evolve, maintaining accurate and up-to-date classifications can be a continuous challenge.
In conclusion, data classification is a crucial aspect of data management that helps organizations effectively organize, protect, and utilize their data. By understanding the importance, key concepts, types, tools, and process of data classification, businesses can ensure better data governance, security, and compliance. Implementing data classification practices enables organizations to maximize the value of their data while minimizing risks and optimizing operational efficiency.
You might also like
Get in Touch to Learn More
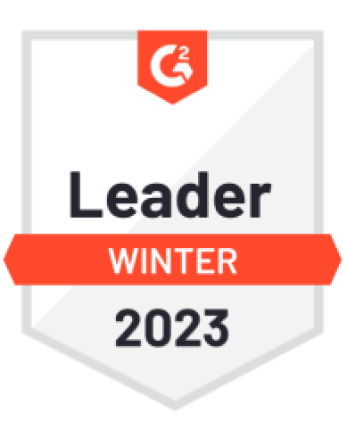
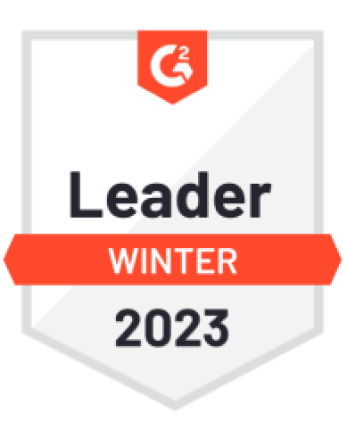

“[I like] The easy to use interface and the speed of finding the relevant assets that you're looking for in your database. I also really enjoy the score given to each table, [which] lets you prioritize the results of your queries by how often certain data is used.” - Michal P., Head of Data