Data Governance Maturity Models and How to Measure It?
Explore the intricacies of data governance maturity models and discover effective strategies for measuring your organization's data management capabilities.
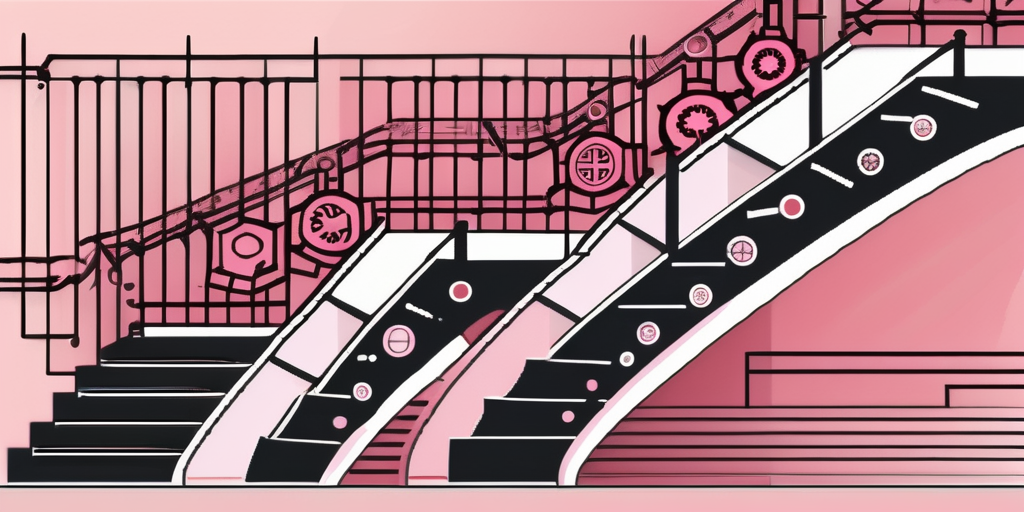
Data governance is a crucial aspect of managing data effectively within organizations. It encompasses the policies, procedures, roles, and standards that ensure that data is accurate, available, and secure. To enhance data governance practices, professionals often turn to maturity models as frameworks for assessment and improvement. This article delves into the understanding and measurement of data governance maturity models.
Understanding Data Governance Maturity Models
Data governance maturity models provide organizations with a structured approach to evaluate their current data governance capabilities and framework. By assessing where they stand, organizations can develop focused strategies for improvement. Maturity models offer insights into various dimensions such as policy creation, data quality, compliance, and stakeholder engagement.
Defining Data Governance
Data governance refers to the overall management of the availability, usability, integrity, and security of the data employed in an enterprise. This management framework encompasses a set of processes and responsibilities that ensure data accuracy, consistency, and privacy throughout its lifecycle.
Organizations define data governance in various ways, but common elements include the establishment of clear ownership, accountability, and quality control mechanisms for data. By defining these roles and processes, organizations can ensure that they can derive value from their data assets. Furthermore, a well-structured data governance framework not only protects sensitive information but also fosters trust among stakeholders, thereby enhancing collaboration across departments. This trust is crucial as it encourages employees to engage with data more effectively, leading to innovative solutions and improved business outcomes.
The Importance of Data Governance Maturity
The maturity of an organization's data governance reflects its capability to manage data effectively. A higher maturity level indicates a comprehensive, proactive approach to governing data, which leads to enhanced decision-making, regulatory compliance, and operational efficiency.
Data governance maturity is important because it aligns data management practices with business goals and facilitates a culture of accountability. By measuring maturity, organizations can identify gaps, prioritize initiatives, and allocate resources more efficiently. Moreover, as organizations navigate an increasingly complex data landscape, maturity models serve as a valuable tool for benchmarking against industry standards and best practices. This benchmarking process not only highlights areas for improvement but also inspires organizations to adopt innovative data governance strategies that can drive competitive advantage.
Key Components of Data Governance Maturity Models
Key components of data governance maturity models often include the following:
- Policies and Standards: Establishing clear guidelines for data management practices.
- Data Ownership: Assigning ownership and accountability for data handling.
- Data Quality Management: Implementing processes for monitoring and improving data quality.
- Compliance and Risk Management: Ensuring adherence to legal and regulatory standards.
- Training and Awareness: Enhancing user knowledge about data governance processes.
- Technology and Tools: Leveraging software solutions for data governance automation.
These components contribute to a robust data governance framework, providing a roadmap for organizations aspiring to improve their maturity levels. Additionally, organizations may find it beneficial to incorporate metrics and performance indicators that can quantitatively assess the effectiveness of their data governance initiatives. By regularly reviewing these metrics, organizations can not only track progress over time but also adjust their strategies in response to evolving business needs and technological advancements. This dynamic approach ensures that data governance remains a vital part of the organizational strategy, adapting to changes in the data landscape and maintaining alignment with overall business objectives.
Different Types of Data Governance Maturity Models
Several data governance maturity models exist, each offering unique perspectives and frameworks for assessment. These models serve as essential tools for organizations striving to enhance their data governance practices, enabling them to identify gaps and areas for improvement in their data management strategies.
Capability Maturity Model Integration (CMMI)
The Capability Maturity Model Integration (CMMI) is a process improvement approach that focuses on enhancing the maturity of organizational processes. It provides a framework for organizations to assess their data governance practices against five maturity levels—from initial to optimized. This structured approach helps organizations understand where they currently stand and what steps they need to take to progress.
CMMI encourages organizations to refine their processes through continuous improvement, providing a structured pathway to elevate data governance maturity. By following this model, organizations can ensure their data practices are consistent and aligned with industry best practices. Furthermore, CMMI promotes a culture of accountability and transparency, which is crucial for fostering trust in data-driven decision-making across the organization.
IBM's Data Governance Maturity Model
IBM has developed a specific maturity model tailored to data governance, identifying levels in terms of processes, policies, and technology. This model emphasizes key areas such as data stewardship, data quality, and regulatory compliance. By clearly defining these components, organizations can better understand the critical elements that contribute to effective data governance.
IBM's framework assists organizations in moving from a reactive to a proactive approach to data governance. It encourages leveraging technology to facilitate governance processes and enhance data visibility across the enterprise. Additionally, this model highlights the importance of integrating data governance with overall business strategy, ensuring that data initiatives align with organizational goals and objectives, ultimately leading to improved business outcomes.
Stanford Data Governance Maturity Model
The Stanford Data Governance Maturity Model provides a comprehensive framework for evaluating the maturity of data governance within an organization. This model includes stages ranging from ad-hoc practices to full integration of governance within business processes. Each stage offers specific criteria that organizations can use to measure their progress and identify areas for development.
Stanford's model emphasizes organizational culture, the importance of data ownership, and the engagement of stakeholders. It aims to foster a shared responsibility for data management, promoting collaboration and accountability among all data users. By cultivating a culture that values data as a strategic asset, organizations can enhance their overall data governance capabilities, leading to better data-driven insights and decision-making processes. Furthermore, the model encourages ongoing training and education for employees, ensuring that everyone understands their role in maintaining data integrity and compliance.
Steps to Measure Data Governance Maturity
Measuring data governance maturity involves systematic steps to accurately assess the current state and identify areas for improvement.
Identifying the Right Metrics
To evaluate data governance maturity, organizations must first identify pertinent metrics. These metrics should reflect both qualitative and quantitative aspects of governance practices.
- Data Quality Scores: Metrics that assess the accuracy, completeness, and consistency of data.
- Compliance Rate: The percentage of adherence to data governance policies and regulations.
- Stakeholder Engagement: Measures of stakeholder involvement and accountability in data management.
Choosing the right metrics is essential for obtaining a holistic view of the organization's data governance state. Additionally, it is beneficial to incorporate industry benchmarks to provide context for the metrics. By comparing performance against similar organizations, businesses can gain insights into best practices and identify opportunities for competitive advantage. Furthermore, organizations should consider the evolving nature of data governance and ensure that their metrics are adaptable to changes in technology, regulations, and business objectives.
Conducting a Data Governance Audit
A data governance audit involves a thorough examination of current governance practices against the chosen maturity model. This audit helps in identifying strengths and weaknesses in existing processes.
During the audit, organizations should assess documentation, interview stakeholders, and evaluate data management practices. This assessment will yield a clear picture of the current maturity level and highlight areas that require focused improvement efforts. It is also important to engage cross-functional teams during the audit process, as diverse perspectives can uncover hidden issues and foster a culture of collaboration. By involving various departments, organizations can ensure that the audit captures the full spectrum of data governance practices, from data creation to data retirement.
Interpreting the Results
Once an audit has been completed, the next step is to interpret the results. This involves analyzing the findings to determine which aspects of data governance are performing well and where gaps exist.
Organizations should use these insights to create a prioritized action plan that addresses identified weaknesses while leveraging existing strengths. The ultimate goal is to elevate the overall maturity of data governance practices. Additionally, it is crucial to communicate these findings effectively to all stakeholders involved. Transparency in the results can foster a sense of ownership and accountability, motivating teams to actively participate in the improvement initiatives. Regularly revisiting and updating the action plan based on feedback and changing circumstances will also ensure that the organization remains agile and responsive to the dynamic landscape of data governance.
Improving Your Data Governance Maturity
Improving data governance maturity requires a structured approach and commitment from all levels of the organization.
Best Practices for Data Governance
Organizations looking to enhance their data governance maturity should consider adopting best practices, which include:
- Creating a Data Governance Council: Establish a dedicated team responsible for overseeing data governance efforts.
- Developing Clear Policies: Formulate comprehensive policies that clarify data handling procedures and responsibilities.
- Fostering a Data-Driven Culture: Encourage a culture where data is prioritized and utilized in decision-making.
Implementing these practices can lay the foundation for a more mature data governance framework.
Overcoming Common Challenges
Organizations frequently encounter challenges when striving to improve data governance maturity. Common obstacles include resistance to change, inadequate resources, and lack of awareness among staff.
To overcome these challenges, organizations should focus on fostering communication, providing adequate training, and ensuring leadership support for data governance initiatives. Addressing these issues head-on will enhance the likelihood of successful improvements in maturity.
The Role of Technology in Data Governance Maturity
Technology plays a significant role in advancing data governance maturity. Tools that automate data management processes and enhance data visibility can greatly facilitate adherence to governance policies.
Data cataloging and metadata management tools, for instance, enable organizations to understand their data landscape better, thus improving governance practices. Embracing technology not only streamlines governance processes but also aids in achieving compliance and enhancing data quality.
In conclusion, understanding and measuring data governance maturity is essential for organizations striving to capitalize on their data assets effectively. By adopting structured maturity models and implementing the associated best practices, organizations can ensure that their data governance frameworks are not only effective but also adaptable to the ever-evolving data landscape.
As you seek to elevate your organization's data governance maturity, consider the innovative solution that CastorDoc offers. With its advanced governance capabilities, intuitive AI assistant, and user-friendly platform, CastorDoc is designed to streamline your data management processes and empower your team with self-service analytics. Whether you're looking to enhance data quality, ensure compliance, or enable effective data utilization, CastorDoc provides the comprehensive tools necessary to transform your data governance framework. Embrace the future of data management and try CastorDoc today to unlock the full potential of your data assets and drive informed decision-making across your enterprise.
You might also like
Get in Touch to Learn More
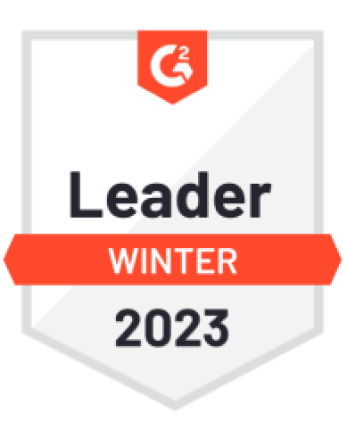
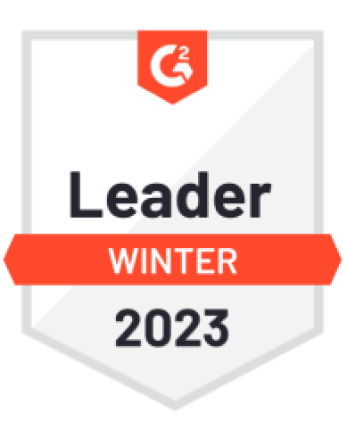

“[I like] The easy to use interface and the speed of finding the relevant assets that you're looking for in your database. I also really enjoy the score given to each table, [which] lets you prioritize the results of your queries by how often certain data is used.” - Michal P., Head of Data