Data Integrity vs Data Validity: Proving They Are Different
Uncover the distinction between data integrity and data validity in this piece
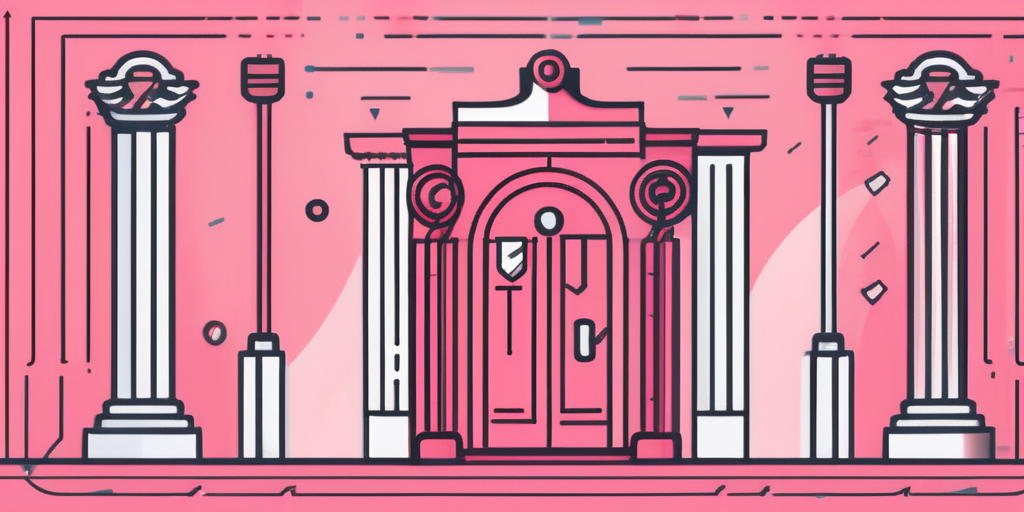
Data integrity and data validity are two fundamental concepts in the field of data management, yet they are often used interchangeably or confused with one another. While both play a crucial role in ensuring the accuracy and reliability of data, it is important to understand that they are distinct concepts with different implications.
Understanding the Basics: Data Integrity and Data Validity
Before delving into the intricacies of data integrity and data validity, let's define each term separately to establish a solid foundation for our discussion.
Data integrity is a critical aspect of data management that goes beyond just accuracy. It encompasses the concept of maintaining the overall quality of data throughout its entire lifecycle. This includes not only ensuring that the data is correct and complete but also safeguarding it from unauthorized access, corruption, or loss. Data integrity is essential for building trust in the data and making informed decisions based on reliable information.
Defining Data Integrity
Data integrity refers to the overall accuracy, consistency, and reliability of data throughout its lifecycle. It involves maintaining the correctness and completeness of data, preventing its corruption or unauthorized alteration, and ensuring its coherence across different data sources and systems.
Ensuring data integrity requires implementing robust data validation processes, data quality checks, and security measures. It involves establishing data governance policies, data validation rules, and data quality standards to maintain the integrity of the data assets. By upholding data integrity, organizations can enhance data security, improve decision-making processes, and build a strong foundation for data-driven initiatives.
Defining Data Validity
On the other hand, data validity focuses on the conformity of data with predefined rules or constraints. Valid data adheres to specific criteria, such as data type, range, format, or business rules. It ensures that data meets the required standards and can be processed and analyzed accurately.
Data validity plays a crucial role in ensuring the reliability and usability of data for various applications. By validating data against predefined criteria, organizations can identify and correct errors, inconsistencies, or discrepancies in the data. This process not only enhances the quality of data but also increases the effectiveness of data analysis and reporting.
The Importance of Data Integrity and Data Validity
Both data integrity and data validity are crucial for organizations that heavily rely on data-driven decision making and information systems. Let's explore the significance of each in more detail.
Ensuring data integrity and data validity goes beyond just maintaining the accuracy of information; it also involves safeguarding the confidentiality and availability of data. Data integrity not only focuses on the accuracy of the data itself but also on protecting it from unauthorized access or cyber threats. Similarly, data validity not only ensures that the data is accurate but also that it is up-to-date and relevant for making informed decisions.
The Role of Data Integrity in Information Systems
Data integrity ensures that data remains consistent and reliable throughout its lifecycle, preventing any unauthorized modifications, deletions, or corruption. In an information system, data integrity ensures that data accurately represents the intended information and supports reliable business operations, analytics, and reporting.
Furthermore, data integrity plays a critical role in maintaining the trust of stakeholders, customers, and partners. By upholding data integrity standards, organizations can demonstrate their commitment to transparency and accountability, which are essential for building strong relationships and credibility in the marketplace.
The Role of Data Validity in Decision Making
Data validity is essential for decision-making processes that heavily rely on accurate and reliable data. Valid data provides the necessary foundation for analysis, forecasting, and planning. Without data validity, decision makers risk basing their decisions on flawed or incomplete information, leading to potentially detrimental outcomes.
Moreover, data validity is closely linked to data quality, which encompasses various aspects such as accuracy, completeness, consistency, and timeliness. By ensuring data validity, organizations can improve the overall quality of their data assets, leading to more effective decision making, improved operational efficiency, and better strategic outcomes.
Distinguishing Between Data Integrity and Data Validity
While data integrity and data validity share the goal of ensuring accurate and reliable data, several key differences set them apart. Let's explore these differences to gain a clearer understanding.
When it comes to data integrity, the focus is on maintaining the overall accuracy, validity, and consistency of data. This involves not only ensuring that the data is correct but also that it is reliable and secure. Data integrity encompasses a broader scope that extends beyond adherence to predefined rules or constraints. It is about the trustworthiness and reliability of the data throughout its lifecycle.
Key Differences in Concepts
Data integrity focuses on maintaining the overall accuracy, validity, and consistency of data. It encompasses a broader scope that extends beyond adherence to predefined rules or constraints. On the other hand, data validity specifically pertains to the conformity of data with specific criteria, rules, or constraints.
On the other hand, data validity is more concerned with the specific criteria, rules, or constraints that data must adhere to. It is about ensuring that the data is correct according to these predefined standards. Data validity is crucial for ensuring that the data is meaningful and can be relied upon for decision-making processes.
Impact on Data Management
Another distinguishing factor is the impact of data integrity and data validity on data management practices. Data integrity measures often involve the implementation of various controls and processes to prevent unauthorized modifications or corruption. Maintaining data integrity requires robust security measures and protocols to safeguard data from unauthorized access or tampering.
Conversely, data validity requires the establishment of validation rules and mechanisms to ensure that the data meets predefined criteria. This involves setting up checks and validations to verify the accuracy and reliability of the data. Data validity plays a crucial role in maintaining data quality and consistency across different systems and processes.
Common Misconceptions About Data Integrity and Data Validity
There are several misconceptions surrounding data integrity and data validity that are worth addressing. By debunking these myths, we can gain a more accurate perspective on these concepts.
Understanding data integrity is crucial in maintaining the quality and reliability of data within an organization. While many believe that data integrity solely focuses on preventing data breaches or unauthorized access, its scope is far broader. Data integrity also encompasses the accuracy, consistency, and reliability of data throughout its lifecycle. This includes ensuring that data is complete, valid, and has not been altered in an unauthorized or unintended manner.
Debunking Myths About Data Integrity
One common myth is that data integrity only concerns the prevention of data breaches or unauthorized access. While security is indeed an important aspect of data integrity, its scope extends beyond confidentiality to encompass overall data accuracy, consistency, and reliability.
Ensuring data integrity involves implementing controls and processes to detect and correct errors, maintain data quality, and preserve the trustworthiness of data. By upholding data integrity, organizations can make informed decisions, improve operational efficiency, and build a foundation of trust with stakeholders.
Debunking Myths About Data Validity
Another misconception is that data validity only involves data type checking or basic format validation. In reality, data validity encompasses a wider range of criteria, including business rules, constraints, and context-specific requirements, ensuring that data is fit for its intended purpose.
Valid data is not only accurate in terms of its format but also aligns with the rules and standards set by the organization. This includes verifying that data meets predefined criteria, such as range checks, uniqueness constraints, and referential integrity. By validating data against these criteria, organizations can ensure that their data is reliable, consistent, and suitable for analysis and decision-making.
Ensuring Data Integrity and Data Validity
To maintain data integrity and data validity, organizations must implement strategies and techniques that address each concept appropriately.
Data integrity is crucial for organizations to ensure that their data remains accurate, consistent, and reliable throughout its lifecycle. Without proper measures in place, data integrity can be compromised, leading to errors, inconsistencies, and potential security breaches. By implementing robust strategies and techniques, organizations can safeguard their data and maintain its integrity.
Strategies for Maintaining Data Integrity
Implementing access controls and user permissions, regular data backups, cryptographic measures, and audit trails are some strategies that organizations can employ to ensure data integrity. These measures help protect data from unauthorized modifications, ensure its accuracy, and enable the detection of any data tampering attempts. Access controls limit who can view or modify data, while audit trails provide a detailed record of data access and changes, aiding in accountability and traceability.
Regular data backups are essential for data integrity as they allow organizations to restore data to a previous state in case of corruption or loss. Cryptographic measures such as encryption help secure data both at rest and in transit, adding an extra layer of protection against unauthorized access or tampering.
Techniques for Ensuring Data Validity
To ensure data validity, organizations should establish and enforce validation rules, conduct data quality assessments, and implement data validation processes. Regularly monitoring data for adherence to predefined criteria will help identify any inconsistencies or errors, allowing for corrective actions to be taken before they impact decision making or business operations. Data quality assessments involve evaluating the accuracy, completeness, and consistency of data, ensuring that it meets the required standards for usability and reliability.
By implementing data validation processes, organizations can automate the verification of data against predefined rules or standards, reducing the risk of errors and ensuring data accuracy. Data validation helps maintain data consistency and reliability, enabling organizations to make informed decisions based on trustworthy information.
Conclusion
In conclusion, data integrity and data validity are distinct but interdependent concepts in the realm of data management. While data integrity ensures overall data accuracy, consistency, and reliability throughout its lifecycle, data validity focuses on adherence to predefined criteria or standards. By understanding their differences and implementing appropriate strategies, organizations can leverage the power of accurate and reliable data to drive informed decision making and achieve their business objectives.
You might also like
Get in Touch to Learn More
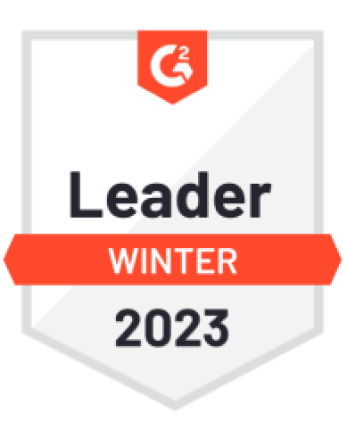
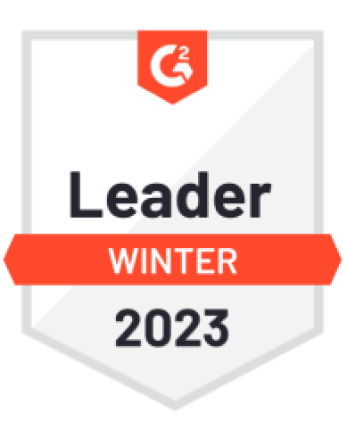

“[I like] The easy to use interface and the speed of finding the relevant assets that you're looking for in your database. I also really enjoy the score given to each table, [which] lets you prioritize the results of your queries by how often certain data is used.” - Michal P., Head of Data