Data Lake & Data Governance: Unifying Disparate Data Sources for Business Success
Discover how data lakes and effective data governance strategies can bring together disparate data sources to drive business success.
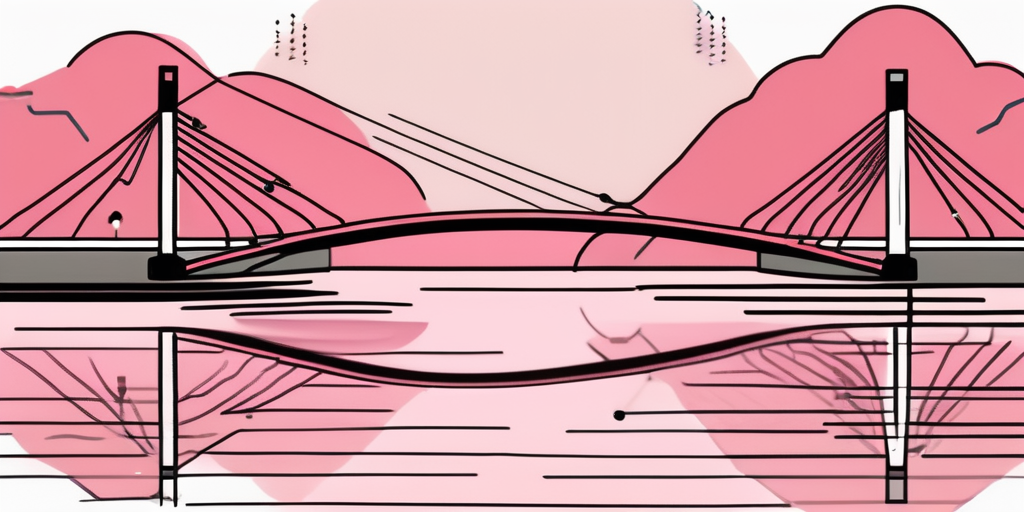
Businesses are increasingly relying on data to make informed decisions and gain a competitive edge. However, the challenge lies in the fact that data is often stored in disparate sources, making it difficult to integrate and derive meaningful insights. This is where the concept of a data lake and data governance comes into play – providing organizations with a unified approach to managing and utilizing their data assets.
Understanding the Concept of Data Lake
Data lake is a centralized repository that allows for the storage of structured, semi-structured, and unstructured data in its raw form. Unlike traditional data warehouses, which require data to be structured and pre-defined, a data lake enables organizations to capture and store vast amounts of data from various sources without the need for upfront schema design. This flexibility and scalability make data lakes an ideal solution for managing diverse datasets.
Furthermore, a data lake is not limited to a specific technology or storage format. It can encompass a wide range of data sources, including transactional databases, log files, social media feeds, and sensor streams. This allows businesses to retain their data in its original format, eliminating the risk of data loss or modification during the ingestion process.
Defining Data Lake
In simpler terms, a data lake can be thought of as a large, centralized reservoir that stores vast amounts of raw data from different sources. It serves as a single source of truth, providing a comprehensive view of the organization's data assets.
Importance of Data Lake in Business
The significance of a data lake for businesses cannot be overstated. By consolidating disparate data sources into a centralized repository, organizations can gain a holistic view of their data, enabling them to identify patterns, trends, and correlations that may not be apparent when data is siloed. This comprehensive understanding of data empowers businesses to make data-driven decisions, enhance operational efficiency, and uncover new opportunities for growth.
Moreover, a data lake fosters data democratization within an organization. By providing a single location for data storage, it enables various teams and departments to access and leverage the same dataset. This eliminates data silos and encourages collaboration, ensuring that insights derived from data are shared across the organization.
Additionally, the flexibility of a data lake allows organizations to adapt to changing data requirements and technologies. As new data sources emerge or existing ones evolve, the data lake can easily accommodate them without the need for significant restructuring. This agility enables businesses to stay ahead in a rapidly evolving data landscape.
Furthermore, data lakes offer advanced analytics capabilities. By integrating data lakes with powerful analytics tools and technologies, organizations can perform complex analyses, such as predictive modeling, machine learning, and real-time data processing. These advanced analytics capabilities unlock valuable insights and enable businesses to gain a competitive edge in their respective industries.
The Role of Data Governance in Data Management
Data governance is a vital aspect of managing data effectively within an organization. It encompasses the policies, processes, and technologies that ensure data quality, data integrity, and data security throughout its lifecycle. Data governance provides a framework for defining data ownership, establishing data standards, and enforcing data management best practices.
What is Data Governance?
Data governance involves the establishment of formalized processes and controls to ensure that data is accurate, consistent, and compliant with regulatory requirements. It encompasses activities such as data quality monitoring, metadata management, data classification, and access control.
The Connection Between Data Governance and Business Success
Effective data governance is directly linked to business success. By implementing robust data governance practices, organizations can maintain data integrity and consistency, thereby enhancing the accuracy and reliability of their insights. This enables them to make well-informed decisions based on trusted data, driving business growth and profitability.
Furthermore, data governance plays a pivotal role in mitigating data-related risks and ensuring compliance with industry regulations, such as GDPR and CCPA. By establishing data policies and procedures, organizations can safeguard sensitive data, protect customer privacy, and avoid costly penalties.
The Intersection of Data Lake and Data Governance
While data lakes and data governance serve distinct purposes, they are closely intertwined and can greatly benefit from each other's capabilities.
How Data Lake and Data Governance Complement Each Other
A data lake provides a centralized repository that can support the implementation of data governance practices. By consolidating disparate data sources in a data lake, organizations can apply data governance policies consistently across all datasets. This ensures that data quality standards, data access controls, and data management practices are enforced uniformly.
Conversely, data governance enhances the effectiveness of a data lake by providing the necessary controls and processes to ensure data integrity and compliance. Data governance mechanisms, such as data lineage tracking, data cataloging, and data stewardship, enable organizations to govern data ingestion, data transformation, and data consumption within the data lake.
Challenges in Integrating Data Lake and Data Governance
Despite the evident benefits of integrating data lakes and data governance, there are challenges that organizations need to overcome. One such challenge is the complexity of managing metadata within a data lake. Metadata management becomes crucial to understand the structure, context, and lineage of data within a data lake. Implementing robust metadata management practices, such as metadata tagging and metadata cataloging, can help organizations address this challenge.
Another challenge is ensuring data quality and data lineage across diverse data sources. Organizations need to establish data quality controls and data lineage tracking mechanisms to maintain the accuracy and reliability of data within the data lake. Furthermore, defining clear data governance policies and responsibilities is essential to ensure that data stewards and data custodians are accountable for data quality and compliance.
Strategies for Unifying Disparate Data Sources
Unifying disparate data sources requires a systematic and well-defined approach. Here are some strategies that organizations can employ:
Identifying and Organizing Disparate Data Sources
The first step towards unifying disparate data sources is to identify the various data sources that exist within the organization. This includes databases, file systems, cloud-based applications, and external data feeds. Once identified, these data sources can be organized based on their relevance, quality, and intended usage.
Data profiling techniques, such as data discovery and data lineage analysis, can aid in determining the characteristics and dependencies of the different data sources. This helps in establishing a clear understanding of the data landscape and identifying any gaps or inconsistencies in the available data.
Techniques for Unifying Disparate Data
Once the data sources have been identified and organized, the next step is to develop techniques for unifying the disparate data. This involves data integration, data transformation, and data cleansing processes.
Data integration involves combining data from different sources into a coherent and consistent format. This can be achieved through techniques such as Extract, Transform, Load (ETL) or data virtualization. ETL processes extract data from source systems, transform it into a standardized format, and load it into the target data lake.
Data transformation involves modifying the structure, format, or content of the data to ensure consistency and compatibility. This may include tasks such as data normalization, data aggregation, or data enrichment.
Data cleansing refers to the process of identifying and correcting or removing errors, inconsistencies, or duplicates within the data. This ensures that the data is accurate and reliable for analysis and decision-making purposes.
Leveraging Data Lake and Data Governance for Business Success
By unifying disparate data sources through a data lake and implementing robust data governance practices, organizations can reap several benefits:
Benefits of a Unified Data System
A unified data system enables organizations to gain a holistic, 360-degree view of their data. This comprehensive understanding of data allows businesses to identify hidden patterns, unearth critical insights, and make informed decisions. It also facilitates collaboration and knowledge sharing across teams, breaking down data silos and fostering innovation.
Furthermore, a unified data system enhances data quality, ensuring that data is accurate, consistent, and up-to-date. Data governance practices help maintain data integrity, enforce data standards, and track data lineage, making the data more trustworthy and reliable.
Future Trends in Data Lake and Data Governance
The evolution of technology and the expanding data landscape bring forth new trends and advancements in data lake and data governance practices.
One such trend is the integration of artificial intelligence (AI) and machine learning (ML) techniques within data lakes and data governance frameworks. AI and ML can automate various data management tasks, such as data profiling, data classification, and data lineage tracking, enhancing the efficiency and effectiveness of data governance processes.
Another emerging trend is the adoption of cloud-based data lakes and data governance solutions. With the proliferation of cloud computing, organizations are increasingly leveraging the scalability and flexibility offered by cloud platforms to store and manage their data assets. Cloud-based data lakes and data governance solutions enable seamless integration of diverse data sources and provide secure access to critical business information.
In conclusion, the combination of a data lake and data governance offers organizations a powerful mechanism to unify their disparate data sources for business success. By implementing a comprehensive data management strategy that incorporates these two concepts, businesses can unlock the full potential of their data assets, gain actionable insights, and stay ahead in today's data-driven world.
You might also like
Get in Touch to Learn More
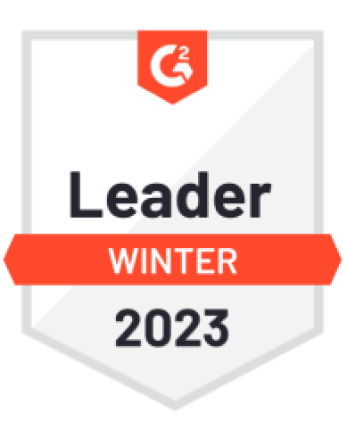
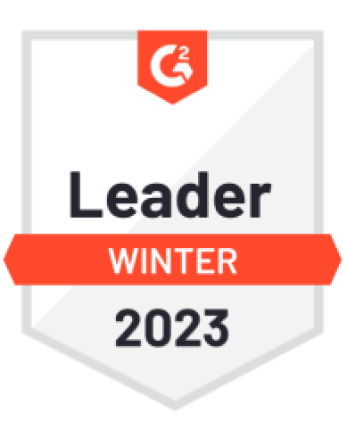

“[I like] The easy to use interface and the speed of finding the relevant assets that you're looking for in your database. I also really enjoy the score given to each table, [which] lets you prioritize the results of your queries by how often certain data is used.” - Michal P., Head of Data