Data Mesh Architecture: Why It Matters to You in 2024?
Discover the future of data architecture with our in-depth article on Data Mesh.
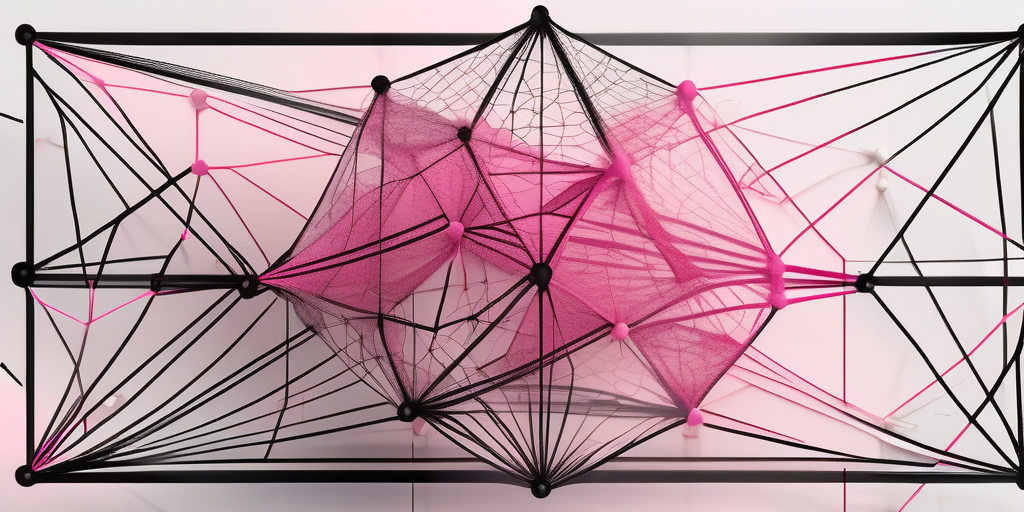
In the world of data architecture, new trends and approaches emerge to address the ever-increasing complexity and scale of data. One such approach that has gained significant attention is data mesh architecture. This article aims to provide a comprehensive understanding of data mesh architecture and why it matters to you in 2024.
Understanding Data Mesh Architecture
Data mesh architecture is a paradigm shift in how organizations handle their data infrastructure and governance. It advocates for decentralized ownership and responsibility over data, enabling better scalability, agility, and data democratization. By adopting data mesh architecture, organizations can break free from the limitations of traditional data architecture and effectively deal with the avalanche of data generated in today's digital age.
The Basics of Data Mesh
At its core, data mesh revolves around the idea of treating data as a product. Instead of relying on centralized data teams, data mesh decentralizes ownership and empowers domain-oriented data teams, known as data products teams, to take ownership of data pipelines, quality, and governance within their domain. This approach promotes a more collaborative and agile environment for data management.
Imagine a scenario where a large e-commerce company is trying to analyze customer behavior to improve their recommendation engine. In a traditional data architecture, the centralized data team would be responsible for collecting, processing, and analyzing all the data. However, with data mesh architecture, the responsibility is distributed among different data product teams. For example, there might be a data product team focused on customer behavior data, another team focused on product data, and so on. Each team takes ownership of their specific domain and is responsible for ensuring the quality and accuracy of the data they manage.
Key Components of Data Mesh Architecture
Data mesh architecture consists of several key components that work together to enable its benefits. Firstly, it emphasizes the use of domain-oriented data products, which are self-contained and encapsulate all aspects of data management. These data products are developed and maintained by dedicated data product teams, allowing for better control and accountability.
Let's take the example of the customer behavior data product team mentioned earlier. This team would be responsible for not only collecting and processing the customer behavior data but also ensuring its quality, defining data schemas, and providing clear documentation for other teams to consume the data. By having dedicated teams for each domain, organizations can ensure that data is managed effectively and that there is a clear understanding of data ownership and responsibilities.
Secondly, data mesh architecture relies on federated data governance to ensure consistency and adherence to policies across different data products. By promoting shared standards and best practices, organizations can achieve harmonious data management without compromising the autonomy of individual data product teams.
Imagine a scenario where different data product teams within an organization are working on similar data sets, such as customer demographics. With federated data governance, there would be a set of agreed-upon standards and policies that all teams follow when working with this data. This ensures consistency in data quality, security, and privacy, while still allowing each team to have the flexibility to manage their specific data needs.
Lastly, data mesh utilizes a decentralized data infrastructure to support the scalability and flexibility needed in today's data-driven world. This infrastructure leverages modern technologies and platforms, such as data lakes, data warehouses, and cloud services, to enable efficient data processing, storage, and access.
With a decentralized data infrastructure, organizations can scale their data processing capabilities based on their specific needs. For example, during peak times, such as Black Friday for an e-commerce company, the infrastructure can automatically scale up to handle the increased data load, ensuring smooth operations. Additionally, by leveraging cloud services, organizations can take advantage of the flexibility and cost-effectiveness offered by the cloud, allowing them to focus on their core business rather than managing complex infrastructure.
The Evolution of Data Architecture
To understand the significance of data mesh architecture, it's crucial to examine the limitations of traditional data architecture and the need for a new approach.
In today's data-driven world, the evolution of data architecture has been a continuous journey marked by significant shifts in paradigms and methodologies. As organizations grapple with the ever-increasing volume, variety, and velocity of data, the need for innovative solutions has become more pressing than ever before.
Traditional Data Architecture
In traditional data architecture, data is treated as a byproduct of applications and processes. Centralized data teams are responsible for managing and governing data, leading to bottlenecks and inefficiencies. Data silos are prevalent, making it challenging to access and integrate data across different business units or domains. As a result, organizations struggle to unlock the full potential of their data.
The traditional approach to data architecture, while effective in its time, has become outdated in the face of modern data challenges. The rigid structures and hierarchical processes of traditional data architecture are ill-equipped to handle the dynamic and complex nature of today's data landscape.
The Shift to Data Mesh Architecture
The rise of data mesh architecture is a response to the shortcomings of traditional data architecture. By decentralizing data ownership and focusing on data products, organizations can foster a data-driven culture and unleash the power of data. Data mesh enables the agility and scalability required to effectively manage vast amounts of data, supporting organizations in making data-informed decisions in real-time.
Embracing data mesh architecture represents a paradigm shift towards a more democratized and collaborative approach to data management. By empowering cross-functional teams to take ownership of data domains, organizations can break down silos and promote a culture of data transparency and accountability.
The Importance of Data Mesh in 2024
Addressing Data Complexity
In 2024, data complexity will continue to grow exponentially. With the proliferation of IoT devices, machine learning models, and the increasing digitalization of business processes, organizations will be inundated with data from various sources. Data mesh architecture provides a framework to handle this complexity by distributing data ownership and empowering domain experts to manage their data effectively.
Furthermore, as data continues to be generated at an unprecedented rate, traditional centralized data architectures struggle to keep up. Data mesh offers a decentralized approach that allows organizations to scale their data infrastructure horizontally, enabling them to adapt to the ever-increasing volume and variety of data. This scalability is essential for organizations looking to future-proof their data management strategies and stay competitive in the rapidly evolving digital landscape.
Enhancing Data Accessibility
Data accessibility is crucial for organizations to gain actionable insights and drive innovation. By adopting data mesh architecture, organizations can break down data silos and facilitate seamless data sharing and collaboration across different teams and domains. This democratization of data promotes better decision-making and empowers individuals to leverage data for problem-solving and innovation.
Moreover, data mesh architecture fosters a culture of data transparency and accountability within organizations. By clearly defining data ownership and responsibilities at the domain level, data mesh encourages a sense of ownership and stewardship among employees. This not only improves data quality and reliability but also enhances trust in the data-driven decision-making process, ultimately leading to more informed and strategic business outcomes.
Benefits of Implementing Data Mesh Architecture
Improved Data Governance
Data mesh architecture promotes a federated governance model, where accountability and responsibility are distributed among data product teams. Each team has a deep understanding of their domain's data, enabling them to implement governance practices tailored to their specific needs. This decentralized approach fosters a culture of data ownership and accountability, leading to better data quality and compliance.
Furthermore, the implementation of data mesh architecture can also enhance data security measures within an organization. With data governance distributed across various teams, the risk of a single point of failure or vulnerability is significantly reduced. This approach ensures that data access controls, encryption standards, and compliance protocols are consistently enforced at a granular level, strengthening the overall security posture.
Scalability and Flexibility
One of the most significant advantages of data mesh architecture is its ability to scale and adapt to changing business requirements. By decentralizing data management, organizations can respond quickly to new data sources, technologies, and analytics needs. Data product teams can independently scale their data infrastructure and adopt new tools and technologies, facilitating innovation and experimentation.
In addition to scalability, the flexibility offered by data mesh architecture enables organizations to leverage diverse data sources and types without significant rearchitecting. This agility in data integration allows for seamless incorporation of structured and unstructured data, real-time streaming data, and legacy systems, providing a holistic view for analytics and decision-making processes. The adaptability of data mesh architecture empowers teams to explore new data-driven initiatives and pivot strategies swiftly in response to market dynamics.
Challenges in Adopting Data Mesh Architecture
Overcoming Organizational Hurdles
Implementing data mesh architecture requires a significant shift in organizational culture and mindset. It challenges existing hierarchies and power structures, necessitating buy-in from leadership and fostering a data-driven culture throughout the organization. Overcoming resistance to change and aligning stakeholders with the benefits of data mesh architecture are crucial for successful implementation.
Technical Challenges and Solutions
Technical challenges may arise when transitioning to data mesh architecture. Integration between data products, ensuring data quality across different domains, and selecting appropriate technologies and platforms can pose significant hurdles. However, leveraging modern data integration tools, establishing data mesh principles, and fostering collaboration between data product teams can help overcome these challenges.
In conclusion, data mesh architecture represents a paradigm shift in data management, offering organizations a decentralized and scalable approach to handling data. By embracing data mesh architecture, organizations can address the complexities of data in 2024 and unlock the full potential of their data assets, leading to improved decision-making, innovation, and competitive advantage. Embrace the future of data architecture, embrace data mesh.
You might also like
Get in Touch to Learn More
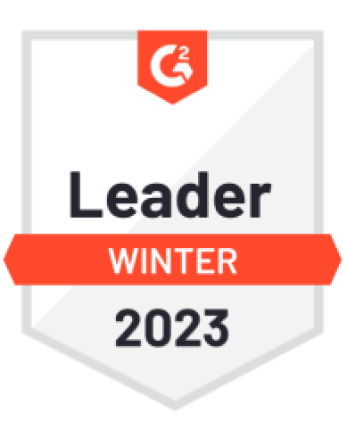
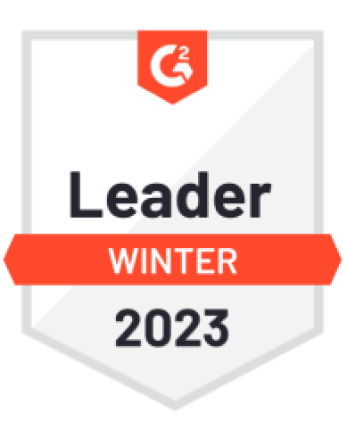

“[I like] The easy to use interface and the speed of finding the relevant assets that you're looking for in your database. I also really enjoy the score given to each table, [which] lets you prioritize the results of your queries by how often certain data is used.” - Michal P., Head of Data