Data Mesh Vs. Data Lake: Differences & Use Cases For 2024
Explore the evolving landscape of data management with this comprehensive guide to the differences and use cases of Data Mesh and Data Lake in 2024.
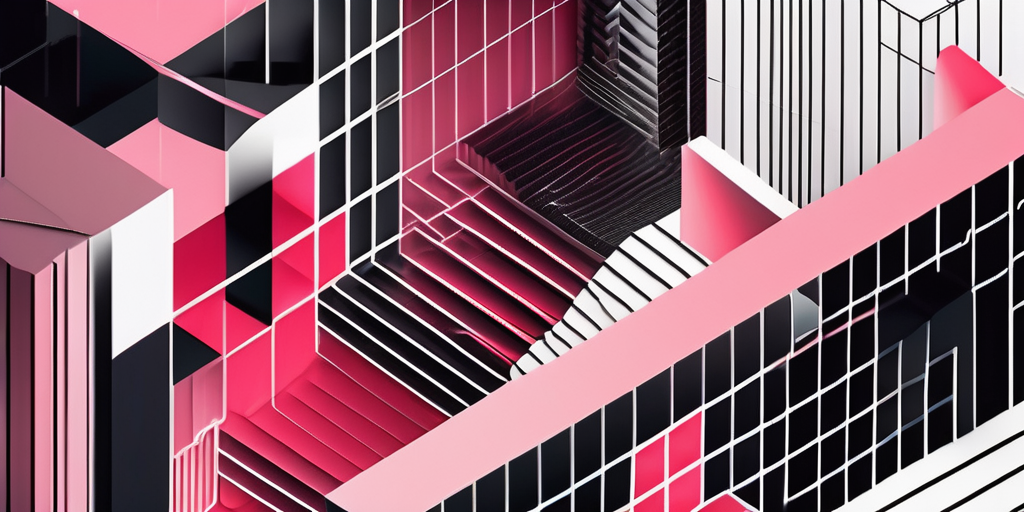
In the world of big data, businesses are constantly seeking innovative approaches to manage and leverage their data assets efficiently. Two prominent concepts that have gained traction in recent years are Data Mesh and Data Lake. While they both serve as repositories for data storage and analysis, they differ in several crucial aspects. This article uncovers the basics of Data Mesh and Data Lake, explores their key differences, discusses their evolution in data architecture, offers insights into choosing between them, and predicts their future trends for 2024.
Understanding the Basics: Data Mesh and Data Lake
What is a Data Mesh?
Data Mesh is an emerging data management paradigm that focuses on decentralization and domain-oriented ownership. Unlike traditional monolithic data architectures, Data Mesh promotes a distributed approach, where data is treated as a product and each domain or team takes responsibility for their data. This enables greater autonomy, agility, and accountability within the organization.
A Data Mesh implementation consists of a network of domain-oriented data platforms or mesh nodes, each responsible for collecting, storing, processing, and sharing data within its domain. These mesh nodes are equipped with self-service capabilities, allowing domain experts to manage and control their data effectively.
Let's dive deeper into the concept of a Data Mesh. Imagine a large organization with multiple business units, each with its own unique data needs and requirements. In a traditional data architecture, all the data would be centralized, making it difficult for individual teams to access and manage their data efficiently. However, with a Data Mesh, each business unit has its own dedicated mesh node, acting as a data hub for that specific domain.
For example, let's consider a retail company that has separate departments for inventory management, sales analytics, and customer insights. Each department can have its own mesh node, responsible for collecting and processing the relevant data. This decentralized approach allows each team to have full control over their data, enabling them to make data-driven decisions quickly and effectively.
Defining Data Lake
Data Lake, on the other hand, is a centralized repository that stores vast amounts of raw and unprocessed data in its native format. It serves as a single source of truth for an organization, pooling data from various sources and serving as an efficient data warehouse. The data lake allows for both structured and unstructured data to coexist, providing a comprehensive and holistic view for data analysis and exploration.
A Data Lake architecture encompasses a storage layer, such as Hadoop Distributed File System (HDFS), that can accommodate huge volumes of data, as well as a processing layer that enables data transformation, indexing, and querying. The flexibility and scalability of Data Lake make it an ideal solution for organizations dealing with increasingly diverse and complex data sets.
Now, let's take a closer look at how a Data Lake operates. Imagine a scenario where a healthcare organization wants to analyze patient data from various sources, such as electronic health records, wearable devices, and medical research databases. In a traditional data warehouse setup, integrating and processing such diverse data would be a daunting task. However, with a Data Lake, all the raw data can be ingested into the lake without any prior transformation or schema enforcement.
Once the data is in the Data Lake, it can be processed and transformed as needed, allowing data scientists and analysts to explore the data in its raw form or apply specific transformations for their analysis. This flexibility empowers organizations to derive valuable insights from their data, uncovering hidden patterns and trends that can drive innovation and decision-making.
Key Differences Between Data Mesh and Data Lake
Data Structure and Organization
In Data Mesh, data is organized and structured around autonomous domains or teams. Each team maintains its data products, including the schemas, storage, and access mechanisms, based on the specific requirements and expertise of their domain. This decentralized approach facilitates faster development cycles, increased data quality, and seamless collaboration between teams.
In contrast, Data Lake follows a centralized approach. It stores data in its raw format, without imposing any predefined structure or organization. This flexibility allows data to be ingested and stored quickly, but it requires additional efforts to ensure proper governance, quality, and discoverability of data across the organization.
Scalability and Flexibility
Data Mesh provides scalable and flexible data management capabilities by distributing the data workload across multiple domains or teams. This decentralized nature allows each domain to independently scale its data infrastructure, adapt to evolving requirements, and make changes without affecting other domains. This ensures agility and resilience in managing data at scale.
On the other hand, Data Lake offers immense scalability, making it suitable for handling vast volumes of data. It can integrate seamlessly with existing data processing tools and technologies, enabling organizations to leverage their existing infrastructure investments. However, without proper governance and management practices, Data Lake scalability can lead to data chaos and inconsistency.
Data Governance and Security
Data Mesh emphasizes localized data ownership, enabling better data governance and security. Each mesh node or domain is responsible for defining and enforcing data policies, access controls, and privacy measures specific to their domain. This approach ensures that data complies with regulatory requirements and privacy regulations, reducing the risk of unauthorized access or data breaches.
Contrarily, Data Lake poses challenges in data governance and security due to its centralized nature. As data from various sources flows into the lake, ensuring data quality, privacy, and compliance becomes crucial. Without proper data governance practices, Data Lake may become a repository of untrusted and unverified data, compromising regulatory compliance and increasing the risk of data breaches.
The Evolution of Data Architecture: From Data Lakes to Data Mesh
The Limitations of Traditional Data Lakes
While Data Lakes have served organizations well in managing large quantities of data, they have shown limitations in certain areas. The centralized nature of Data Lakes often leads to bottlenecks in data access and processing, especially when dealing with diverse data types or when multiple teams require simultaneous access to the same data.
Data Lakes also present challenges in data governance and discoverability. As data accumulates in a centralized repository, it becomes increasingly difficult to maintain data quality, ensure proper access controls, and discover relevant data for analysis.
The Emergence and Advantages of Data Mesh
Recognizing the limitations of traditional Data Lakes, the concept of Data Mesh has emerged as a compelling alternative. Data Mesh decentralizes data management, empowering domain experts and teams to take ownership and accountability for their data. This approach brings numerous benefits, including increased autonomy, faster innovation, improved data quality, and enhanced collaboration between domains.
Data Mesh enables organizations to scale their data capabilities without compromising agility or introducing dependencies across teams. It provides a framework for designing and building data products as self-contained entities, ensuring that the data is accurate, reliable, and governed within each domain.
Choosing Between Data Mesh and Data Lake
Factors to Consider
When selecting between Data Mesh and Data Lake, it is crucial to consider various factors specific to your organization. These include the size and structure of your organization, the level of data governance required, the complexity and diversity of your data sources, and the skills and expertise of your teams.
Organizations with multiple autonomous teams and a need for decentralized decision-making may find Data Mesh more suitable. On the other hand, organizations dealing with massive volumes of data and requiring a centralized repository may opt for Data Lake.
Suitability for Different Business Sizes and Types
Both Data Mesh and Data Lake can benefit organizations of all sizes, but their suitability varies based on the nature of the business. Start-ups and small businesses with limited resources may find Data Mesh more advantageous, as it allows them to start small, iterate quickly, and scale as they grow. Data Mesh also supports rapid experimentation and innovation, enabling businesses to stay competitive in a dynamic market.
On the other hand, large enterprises with extensive data infrastructure and existing data lakes may prefer sticking to Data Lake architectures, especially if they have already established data governance and security practices. Data Lakes provide a consolidated view of data across the organization, supporting enterprise-wide analytics initiatives and facilitating cross-team collaborations.
Future Trends: Data Mesh and Data Lake in 2024
Predicted Developments in Data Mesh
As organizations continue to adopt and refine their Data Mesh strategies, several developments are expected to shape the future of Data Mesh. Advanced tooling and frameworks will emerge to support domain teams in managing their data products effectively. Data Mesh will further integrate with cloud-native technologies, enabling seamless scalability, portability, and interoperability of data across domains. The community around Data Mesh will grow, fostering collaboration, sharing best practices, and evolving standards that promote interoperability and compatibility between different Data Mesh implementations.
Anticipated Changes in Data Lake Use
Data Lake architectures will continue to evolve to address the challenges faced by organizations. Improved data governance and security practices will be prioritized to ensure the trustworthiness and compliance of data stored in Data Lakes. Advances in metadata management and data cataloging will enhance data discoverability and enable efficient data exploration. Data Lakes will evolve to incorporate more data processing capabilities, including real-time analytics and machine learning, to support the growing demands for insights and automation.
In conclusion, Data Mesh and Data Lake are two distinct approaches to data management, each with its own strengths and use cases. Data Mesh empowers autonomous teams to take ownership of their data, fostering agility and accountability, while Data Lake provides a centralized repository for scalable data storage and analysis. Choosing between Data Mesh and Data Lake requires careful consideration of organizational factors and requirements. As we move further into 2024, both Data Mesh and Data Lake will continue to evolve, responding to the changing needs and demands of organizations in the era of big data.
You might also like
Get in Touch to Learn More
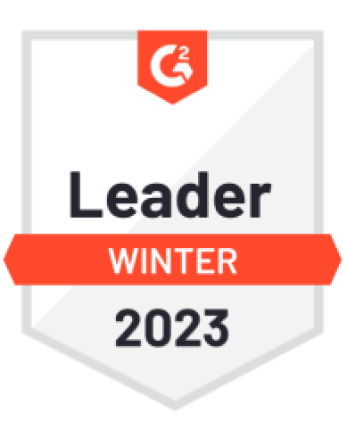
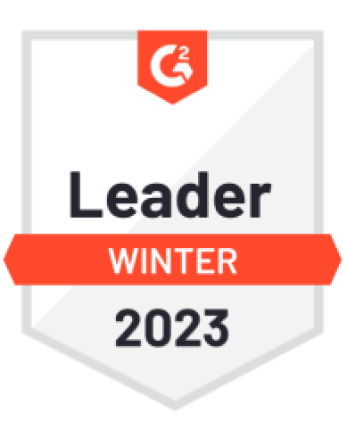

“[I like] The easy to use interface and the speed of finding the relevant assets that you're looking for in your database. I also really enjoy the score given to each table, [which] lets you prioritize the results of your queries by how often certain data is used.” - Michal P., Head of Data