Data Mesh vs. Data Warehouse: How Are They Different?
Discover the key differences between Data Mesh and Data Warehouse.
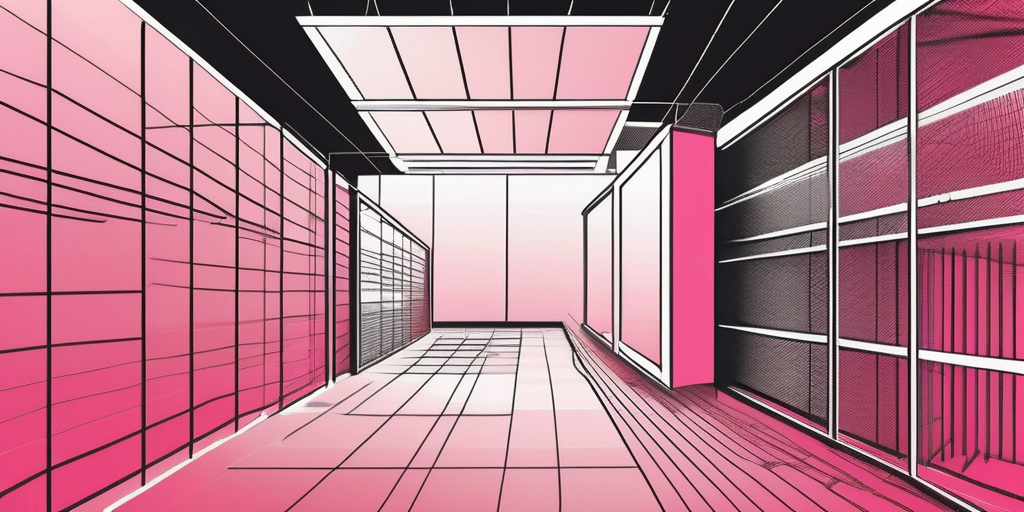
Understanding the Basics of Data Mesh and Data Warehouse
The world of data management has undergone significant transformations in recent years. Two approaches that have gained prominence are Data Mesh and Data Warehouse. To fully comprehend their differences, it is essential to delve into the fundamental concepts of each.
In addition to Data Mesh and Data Warehouse, another emerging concept in the realm of data management is Data Lake. A Data Lake is a vast pool of raw data, stored in its native format until it is needed. Unlike Data Warehouse, which structures data before storing it, Data Lake allows for the storage of unstructured and semi-structured data, providing more flexibility for future analysis and processing.
Defining Data Mesh
Data Mesh is a relatively new paradigm that challenges the traditional centralization of data management. It advocates for a decentralized approach, where data is treated as a product and owned by individual domains or teams within an organization. These teams have the autonomy to manage, govern, and curate their own data products, enabling better collaboration and innovation.
Implementing Data Mesh involves a shift towards domain-oriented decentralized data architecture, where data ownership, access, and quality are prioritized at the domain level. This approach aims to break down data silos, promote cross-functional collaboration, and increase agility in data-driven decision-making processes.
Defining Data Warehouse
On the other hand, a Data Warehouse is a centralized repository of structured, integrated, and historical data that serves as a single source of truth for an organization. It follows a schema-on-write approach, where data is transformed and loaded into a predefined structure to facilitate reporting, analysis, and decision-making processes.
Data Warehouse solutions often involve Extract, Transform, Load (ETL) processes to extract data from various sources, transform it into a consistent format, and load it into the warehouse for analysis. This structured approach ensures data consistency and reliability for business intelligence and reporting purposes.
The Evolution of Data Management
The journey from traditional data management approaches to the emergence of Data Mesh and the coexistence of Data Warehouse has been an evolutionary one.
As technology continues to advance at a rapid pace, the landscape of data management is constantly evolving. Organizations are now exploring innovative solutions to address the challenges posed by the ever-increasing volume and complexity of data.
The Traditional Data Warehouse Approach
Historically, organizations relied heavily on Data Warehouses as the primary means of managing their data. These centralized systems offered a unified view of data, enabling efficient reporting and analysis. However, they often faced challenges with scalability and flexibility, as they struggled to accommodate the increasing volume, variety, and velocity of data.
Despite their limitations, Data Warehouses played a crucial role in laying the foundation for modern data management practices. They provided a structured framework for storing and organizing data, allowing businesses to gain valuable insights and make informed decisions based on historical information.
The Emergence of Data Mesh
In response to the limitations of Data Warehouses, the concept of Data Mesh was introduced. This decentralized approach addresses the scalability and flexibility issues by distributing data ownership and governance to domain-oriented teams. Each team manages their own data infrastructure and collaborates with others using well-defined protocols and standards.
By decentralizing data management responsibilities, Data Mesh enables organizations to adapt more effectively to changing business needs and evolving data requirements. This approach promotes a culture of data ownership and accountability, empowering teams to take ownership of their data assets and drive innovation within their respective domains.
Key Differences Between Data Mesh and Data Warehouse
Architectural Differences
Data Mesh employs a domain-oriented architecture, where data is treated as a product and each domain team is responsible for their data infrastructure. This approach allows for greater agility and autonomy within each domain, as teams can make decisions tailored to their specific needs without impacting the entire system. Additionally, the domain-oriented architecture of Data Mesh promotes a more decentralized data ecosystem, enabling faster innovation and adaptation to changing business requirements.
On the other hand, a Data Warehouse follows a centralized architecture, with a predefined structure and a single repository for data. While this centralized approach can provide a unified view of the organization's data, it can also lead to bottlenecks and dependencies, especially when multiple teams need to access and analyze the data simultaneously.
Data Governance and Ownership
Data ownership and governance are fundamental differentiators between Data Mesh and Data Warehouse. In Data Mesh, each domain team has ownership of their data and is accountable for its quality and governance. This distributed ownership model fosters a sense of responsibility and expertise within each team, leading to better data quality and more efficient decision-making processes. Moreover, the decentralized governance structure of Data Mesh promotes a culture of collaboration and transparency, where data issues can be addressed at the source.
In contrast, a Data Warehouse has a centralized governance structure where data ownership lies with a central team. While this centralized approach can provide clear accountability and consistency in data management practices, it may also lead to delays in decision-making and hinder innovation, as teams have to rely on the central team for data-related tasks and approvals.
Scalability and Flexibility
Scalability and flexibility are areas where Data Mesh shines. With its distributed approach, it can easily scale by adding or removing domain teams as per the organization's changing requirements. This scalability is particularly advantageous in dynamic environments where new data sources need to be integrated quickly, or when existing domains need to be restructured to meet evolving business needs. Additionally, the flexibility of Data Mesh allows each domain team to choose the tools and technologies that best suit their requirements, enabling them to innovate and experiment without being constrained by a centralized infrastructure.
On the other hand, Data Warehouse often struggles with scalability due to its centralized nature. As data volumes and processing requirements grow, the centralized architecture of a Data Warehouse can become a bottleneck, leading to performance issues and increased maintenance costs. Moreover, the rigidity of a Data Warehouse's structure can limit its ability to adapt to changing data sources and analytical needs, making it challenging to keep pace with the organization's evolving requirements.
Pros and Cons of Data Mesh and Data Warehouse
Advantages of Data Mesh
- Data Mesh promotes a culture of collaboration and innovation, facilitating cross-functional teams to work together efficiently.
- It offers scalability by allowing the organization to scale horizontally by adding new domain teams and expanding the data ecosystem.
- Data Mesh grants ownership and accountability to domain teams, leading to improved data quality and governance.
Disadvantages of Data Mesh
- Implementing Data Mesh requires a cultural shift within an organization, which can be challenging to achieve.
- The distributed nature of Data Mesh can lead to data silos if not properly managed.
- Data Mesh might introduce complexities in data integration and interoperability.
Advantages of Data Warehouse
- Data Warehouse provides a unified view of data, ensuring consistency and accuracy for reporting and analysis.
- It offers efficient data governance and security, as all data goes through a centralized process for validation and quality control.
- Data Warehouse is well-suited for organizations with structured and stable data requirements.
Disadvantages of Data Warehouse
- Data Warehouse can be inflexible and challenging to adapt to changing business needs, particularly with respect to handling unstructured or semi-structured data.
- Scalability can be a concern, especially as data volumes continue to grow at an exponential rate.
- Data Warehouse implementation often requires significant upfront investment in hardware, software, and resources.
As organizations grapple with the ever-increasing complexity and magnitude of data, understanding the differences between Data Mesh and Data Warehouse becomes crucial. While both have their own merits and drawbacks, choosing the right approach depends on the organization's specific needs, data maturity level, and long-term goals.
One of the additional advantages of Data Mesh is its ability to foster innovation and experimentation within an organization. By empowering domain teams to take ownership of their data, Data Mesh encourages a culture of exploration and creativity. This can lead to the discovery of new insights and opportunities that might have otherwise gone unnoticed in a traditional data warehouse setup. Furthermore, the collaborative nature of Data Mesh allows for cross-pollination of ideas and expertise, leading to the development of novel solutions and approaches to data challenges.
On the other hand, one of the drawbacks of a Data Warehouse is its potential lack of flexibility in handling unstructured or semi-structured data. While Data Warehouses excel at managing structured data, such as transactional records or customer information, they may struggle with the complexities of unstructured data sources like social media feeds or sensor data. This limitation can hinder organizations that rely heavily on these types of data for their analytics and decision-making processes. Additionally, the rigid structure of a Data Warehouse can make it challenging to adapt to rapidly changing business needs, as any modifications to the data model or schema require significant effort and time.
You might also like
Get in Touch to Learn More
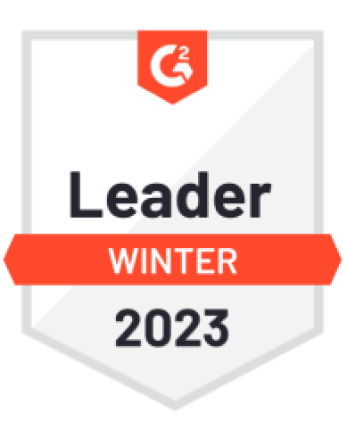
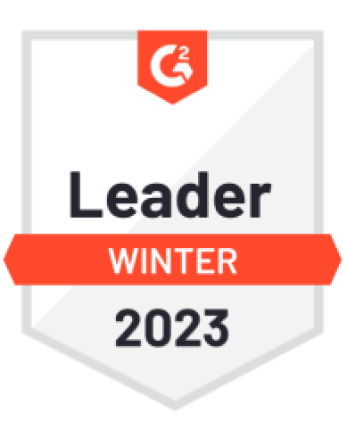

“[I like] The easy to use interface and the speed of finding the relevant assets that you're looking for in your database. I also really enjoy the score given to each table, [which] lets you prioritize the results of your queries by how often certain data is used.” - Michal P., Head of Data