Data Quality and Observability: Key Differences & Relationships!
Explore the intricate relationship between data quality and observability .
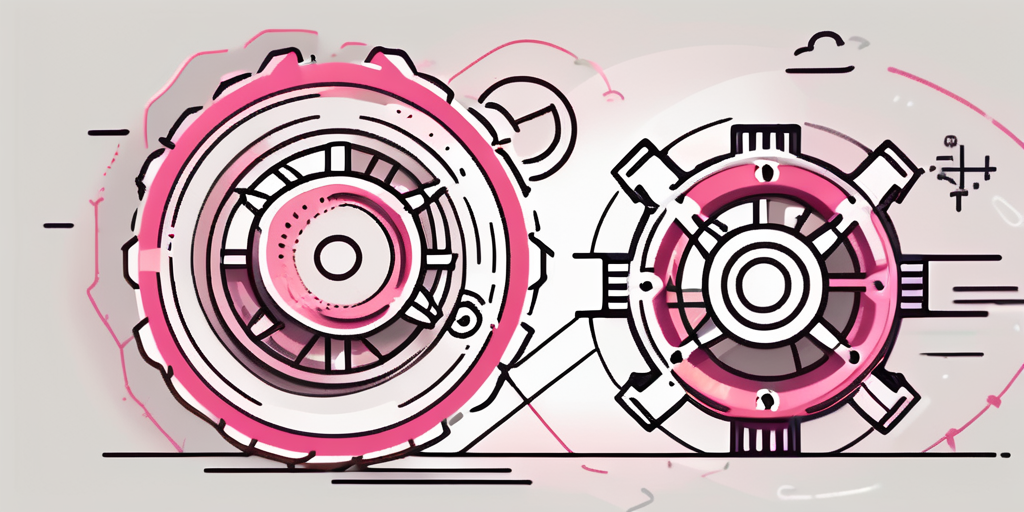
Data quality and observability are two critical aspects of managing and analyzing data in today's digital age. While they share a common goal of ensuring accurate and reliable data, there are distinct differences between them. Understanding these differences and how they relate to each other is essential for organizations to optimize their data management strategies.
Understanding the Basics of Data Quality
Data quality refers to the accuracy, completeness, consistency, and validity of data. In simple terms, it is the ability of data to meet specific requirements and objectives. Reliable data quality is crucial for organizations to make informed decisions, discover insights, and drive business growth.
Ensuring high-quality data involves a combination of processes, technologies, and best practices. Data quality management frameworks often include data profiling, data cleansing, data enrichment, and data governance strategies. These methodologies help organizations maintain clean, reliable data that can be trusted for critical decision-making processes.
Defining Data Quality
Data quality can be defined as the measure of data's fitness for purpose. It encompasses various aspects, including data accuracy, completeness, timeliness, and relevance. High-quality data provides a solid foundation for operational efficiency, improved customer experiences, and strategic planning.
Moreover, data quality is not a one-time task but an ongoing effort that requires continuous monitoring and improvement. Organizations must establish data quality metrics, set benchmarks, and implement data quality tools to ensure that data remains accurate, consistent, and up-to-date.
Importance of High-Quality Data
High-quality data plays a crucial role in numerous applications and business functions. It enables organizations to gain a competitive edge by ensuring reliable analytics, effective decision-making, and mitigating risks. Conversely, poor data quality can lead to incorrect insights, misguided strategies, and compromised operational performance.
Furthermore, high-quality data is essential for regulatory compliance and maintaining customer trust. Organizations that prioritize data quality demonstrate a commitment to integrity and excellence, fostering stronger relationships with stakeholders and enhancing their reputation in the marketplace.
Factors Affecting Data Quality
Data quality is influenced by several factors. These include data collection methods, data entry processes, data integration, data storage, and data validation techniques. In addition, data quality is impacted by factors such as data duplication, inconsistency, incompleteness, and outdated information.
Addressing these factors requires a holistic approach that involves data stewardship, data quality audits, and data quality training for employees. By proactively managing data quality challenges, organizations can harness the full potential of their data assets and drive sustainable business growth.
Delving into Data Observability
Data observability is a relatively newer concept that focuses on monitoring, measuring, and improving the quality of data pipelines and processes. It ensures that data flows smoothly and provides visibility into the health and performance of data infrastructure.
What is Data Observability?
Data observability refers to the ability to gain insights into the behavior and health of data systems. It involves monitoring data pipelines, data transformations, and data sources to ensure data quality, reliability, and performance. By continuously observing data processes, organizations can identify and resolve issues proactively.
The Role of Data Observability in Business
Data observability plays a vital role in enabling organizations to make data-driven decisions with confidence. It helps identify bottlenecks, anomalies, and data quality issues in real-time, allowing for prompt remediation. By improving data observability, businesses can enhance their operational efficiency, reduce downtime, and optimize resource allocation.
Components of Data Observability
Data observability comprises various components that work together to ensure data reliability. These include monitoring data validity, data consistency, data availability, data integrity, and data lineage. Additionally, advanced techniques such as anomaly detection, outlier analysis, and trend analysis are employed to enhance data observability.
Let's delve deeper into the components of data observability to understand how they contribute to the overall data quality and reliability. Monitoring data validity involves verifying that the data being processed adheres to predefined rules and standards. This ensures that only accurate and relevant data is used for analysis and decision-making. Data consistency, on the other hand, focuses on ensuring that data remains consistent across different systems and processes. By detecting and resolving inconsistencies, organizations can avoid data discrepancies and improve the trustworthiness of their insights.
Data availability is another crucial component of data observability. It involves monitoring the accessibility and responsiveness of data sources and ensuring that they are consistently available for analysis. By ensuring data availability, organizations can minimize disruptions and delays in data-driven processes. Data integrity, on the other hand, focuses on maintaining the accuracy, completeness, and reliability of data throughout its lifecycle. By implementing data validation techniques and data quality checks, organizations can ensure that their data remains trustworthy and free from errors.
Lastly, data lineage plays a significant role in data observability. It involves tracking the origin, transformations, and movement of data across different systems and processes. By establishing a clear data lineage, organizations can trace the path of data and identify any potential issues or bottlenecks. This helps in understanding the impact of changes or failures on downstream processes and enables efficient troubleshooting.
Distinguishing Between Data Quality and Observability
While data quality and observability are interconnected, they focus on distinct aspects of data management.
Understanding the nuances between data quality and observability is crucial for organizations striving to harness the full potential of their data assets. Data quality primarily emphasizes the overall accuracy and fitness of data for specific purposes. It delves into the characteristics and attributes of data, such as completeness, reliability, and relevance. Organizations need high-quality data to ensure that their analyses and decision-making processes are based on reliable information.
Key Differences Explained
Data quality primarily emphasizes the overall accuracy and fitness of data for specific purposes. It focuses on the characteristics and attributes of data, such as completeness, reliability, and relevance. On the other hand, data observability focuses on monitoring and maintaining the health and performance of data systems, ensuring that data flows efficiently and reliably.
On the flip side, data observability is concerned with the real-time monitoring and visibility into data systems. It ensures that organizations can track the flow of data, detect anomalies or bottlenecks, and optimize performance. By enhancing data observability, organizations can proactively address issues and ensure that their data infrastructure operates smoothly and efficiently.
How They Complement Each Other
Data quality and observability are interdependent and reinforce each other. High-quality data is a prerequisite for effective data observability. Conversely, by monitoring and maintaining data observability, organizations can identify and rectify data quality issues, ensuring reliable insights and informed decision-making.
When data quality and observability work hand in hand, organizations can unlock the full potential of their data assets. By prioritizing both aspects, businesses can ensure that their data is not only accurate and reliable but also transparent and easily monitored. This comprehensive approach to data management lays a solid foundation for data-driven strategies and sustainable growth.
Enhancing Data Quality for Better Observability
To achieve optimal data management, organizations must adopt best practices for data quality management and leverage data observability techniques.
In addition to implementing best practices for data quality management and leveraging data observability techniques, organizations can further enhance their data quality by investing in advanced data analytics tools. These tools can provide deeper insights into data patterns, trends, and anomalies, allowing organizations to make more informed decisions based on high-quality data.
Best Practices for Data Quality Management
Implementing effective data quality management practices is crucial for maintaining high-quality data. This includes establishing data quality standards, data governance frameworks, data validation mechanisms, and investing in data cleansing and transformation processes. Regular data quality assessments and audits are also essential to identify potential issues and ensure ongoing data quality improvements.
Furthermore, organizations can enhance their data quality management by implementing data quality monitoring tools that provide real-time visibility into data quality metrics. These tools can help organizations track key performance indicators related to data quality, identify areas for improvement, and take proactive measures to address any emerging issues.
Leveraging Data Observability for Quality Improvement
Data observability can be a powerful tool to identify and rectify data quality issues. By continuously monitoring data pipelines, organizations can detect anomalies, data inconsistencies, and data flow disruptions. Proactive measures can then be taken to resolve these issues, ensuring better data quality and observability.
Moreover, organizations can enhance their data observability efforts by implementing machine learning algorithms that can automatically detect patterns and anomalies in data streams. By leveraging machine learning for data observability, organizations can improve their ability to detect and address data quality issues in real time, ensuring a more robust and reliable data infrastructure.
The Future of Data Quality and Observability
The world of data management and analytics is ever-evolving. Keeping up with emerging trends in data quality and observability is essential to stay ahead in the competitive landscape.
Emerging Trends in Data Quality
Emerging technologies, such as machine learning and artificial intelligence, are revolutionizing data quality management. These technologies enable automated data validation, anomaly detection, and predictive analysis, improving data quality and reducing manual efforts.
Predictions for Data Observability
Data observability is expected to become increasingly critical as organizations rely more on data-driven insights. Predictive data observability, enhanced by AI and machine learning algorithms, will enable proactive issue detection and resolution. Real-time monitoring and advanced visualization techniques will also play a significant role in improving data observability.
In summary, data quality and observability are essential pillars of effective data management. While data quality focuses on the accuracy and reliability of data, data observability ensures smooth data flows and maintains the health and performance of data systems. By implementing best practices for data quality management and leveraging data observability techniques, organizations can unlock the true potential of their data and drive business success in an increasingly data-driven world.You might also like
Get in Touch to Learn More
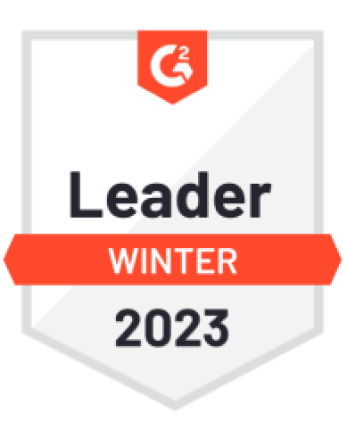
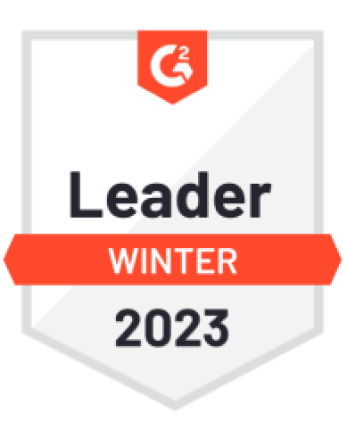

“[I like] The easy to use interface and the speed of finding the relevant assets that you're looking for in your database. I also really enjoy the score given to each table, [which] lets you prioritize the results of your queries by how often certain data is used.” - Michal P., Head of Data