5 Steps to Improve Data Quality in Healthcare
Learn 5 practical steps to enhance data quality in healthcare, ensuring accurate and reliable patient information.
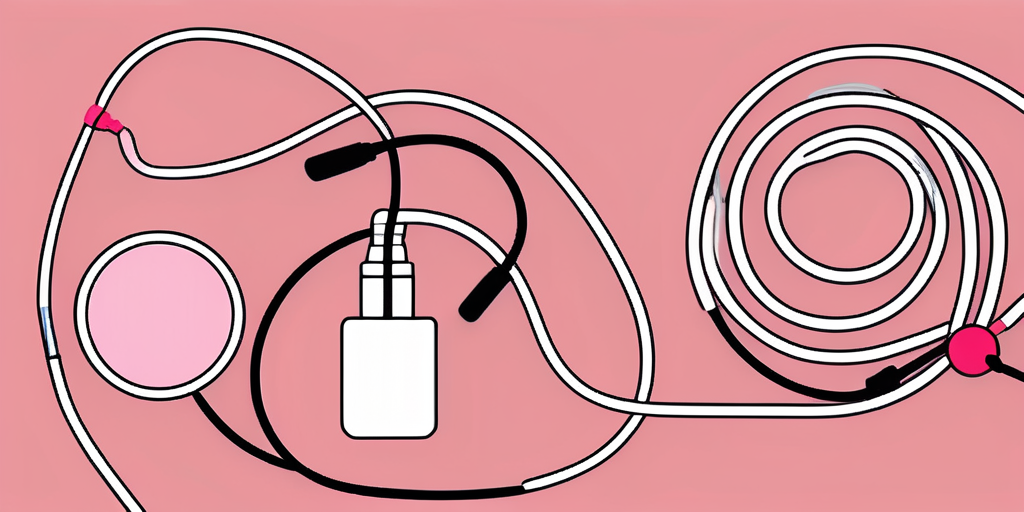
In the field of healthcare, data has become increasingly vital. It fuels decision-making processes, supports research and analysis, and ultimately contributes to the overall quality of patient care. However, data is only as valuable as its quality, and the accuracy and reliability of healthcare data are paramount. This article will outline a comprehensive approach to improving data quality in the healthcare industry, consisting of five key steps.
Understanding the Importance of Data Quality in Healthcare
Data plays a pivotal role in modern healthcare, serving as the foundation for evidence-based medicine and patient-centric care. Accurate and high-quality data ensure that healthcare providers have the necessary information to make informed decisions, improving both patient outcomes and organizational efficiency.
On the other hand, poor data quality can have severe consequences. Inaccurate data can lead to misdiagnoses, inappropriate treatments, and compromised patient safety. It can also hinder research efforts, impede public health initiatives, and result in financial losses for healthcare organizations.
The Role of Data in Modern Healthcare
Data underpins every aspect of healthcare. It enables healthcare providers to track patient demographics, medical histories, and treatment plans. Moreover, it facilitates clinical research, aids in disease surveillance, and supports population health management.
Effective data management promotes seamless care coordination and enables the exchange of health information among different healthcare entities. It empowers patients to actively participate in their own care and ensures interoperability among disparate systems, fostering a comprehensive and integrated healthcare ecosystem.
The Impact of Poor Data Quality
Poor data quality poses substantial challenges to the healthcare industry. Inaccurate or incomplete data can lead to medical errors, such as incorrect diagnoses, wrong medications, or inappropriate treatments. These errors not only harm patients but also contribute to rising healthcare costs due to avoidable hospital readmissions and unnecessary procedures.
Furthermore, poor data quality impedes healthcare analytics, making it difficult to identify trends, assess performance, and measure outcomes accurately. It hampers population health management initiatives by inhibiting the ability to track disease prevalence, plan interventions, and allocate resources effectively.
One specific example of the impact of poor data quality is in the field of clinical research. Research studies rely heavily on accurate and reliable data to draw meaningful conclusions and contribute to medical advancements. However, if the data used in these studies is of poor quality, the results may be skewed or misleading. This can lead to wasted resources, false conclusions, and delays in the development of new treatments or therapies.
Another area where poor data quality can have a significant impact is in healthcare reimbursement. Insurance companies and government payers rely on accurate data to determine reimbursement rates for healthcare services. If the data submitted by healthcare providers is of poor quality, it can lead to underpayment or overpayment, creating financial challenges for both the providers and the payers.
In addition to these challenges, poor data quality can also hinder the implementation of population health management strategies. Population health management aims to improve the health outcomes of a specific group of individuals by analyzing data and implementing targeted interventions. However, if the data used to identify high-risk individuals or track the effectiveness of interventions is of poor quality, it becomes difficult to accurately assess the impact of these strategies and make informed decisions.
Overall, the importance of data quality in healthcare cannot be overstated. Accurate and reliable data is essential for providing high-quality care, conducting meaningful research, and improving population health outcomes. Healthcare organizations must prioritize data quality initiatives and invest in robust data management systems to ensure the integrity and usefulness of the data they collect and analyze.
Step 1: Establishing Data Governance
Data governance is the foundation of a robust data quality management framework. It involves defining policies, processes, and responsibilities to ensure data integrity, security, and privacy. In the healthcare context, data governance aims to establish guidelines for data management and promote accountability throughout the organization.
Defining Data Governance in Healthcare
Data governance in healthcare encompasses a range of activities, including data stewardship, data standards development, and data quality monitoring. It involves creating a governance structure that defines roles and responsibilities, establishes data governance committees, and ensures compliance with regulatory requirements.
With the increasing digitization of healthcare, the volume and complexity of data have grown exponentially. This has made data governance even more crucial in ensuring the accuracy and reliability of patient information. By implementing data governance, healthcare organizations can establish clear data ownership, improve data documentation, and enhance data quality through rigorous data validation and data cleansing procedures.
Key Components of Effective Data Governance
An effective data governance program in healthcare includes several key components. These components include assigning data stewards, who are responsible for data quality and ensuring adherence to data standards. Data governance also involves developing data policies and procedures, establishing data quality metrics, and implementing data auditing and monitoring mechanisms.
Furthermore, data governance is not a one-time effort but an ongoing process. It requires continuous evaluation and improvement to keep up with evolving technology and changing regulatory requirements. Collaboration and communication are critical to successful data governance implementation. Building a culture of data stewardship and creating channels for feedback and continuous improvement are essential for maintaining an effective data governance framework.
Moreover, data governance goes beyond just technical aspects. It also involves engaging stakeholders across the healthcare organization, including clinicians, administrators, and IT professionals. By involving all relevant parties, data governance can address the unique challenges and opportunities present in different healthcare settings.
Step 2: Implementing Data Quality Management Tools
Data quality management tools are essential for assessing, monitoring, and improving the quality of healthcare data. These tools facilitate data profiling, data cleansing, and data enrichment, enhancing the accuracy, consistency, and completeness of healthcare data.
The Need for Data Quality Tools
In a rapidly evolving healthcare environment, the volume and complexity of data continue to grow exponentially. Manual data quality checks and interventions are no longer feasible or efficient. Data quality management tools automate data validation, identify data anomalies, and detect duplicates or inconsistencies, enabling efficient data cleansing processes.
Selecting the Right Tools for Your Organization
When selecting data quality management tools, healthcare organizations must consider their specific data quality requirements, budgetary constraints, and scalability needs. Robust tools should offer features such as data profiling, data parsing, data matching, and data linking capabilities.
Moreover, integration with existing health information systems and interoperability with external data sources are essential to enable seamless data exchange and promote data standardization across the healthcare ecosystem.
Step 3: Standardizing Data Collection Processes
Standardization is crucial to ensure consistency and comparability of healthcare data across different systems, departments, and organizations. Standardized data collection processes facilitate data integration, aggregation, and analysis, supporting evidence-based decision making at all levels of healthcare delivery.
The Importance of Standardization in Data Collection
Data standardization involves adopting uniform data elements, terminologies, and coding systems. This enables healthcare providers to capture and exchange data in a consistent format, reducing ambiguity and minimizing the risk of data errors or misinterpretations.
Standardized data collection processes also enable the sharing of data between healthcare providers, encourage data interoperability, and improve the accuracy and reliability of data analysis and reporting.
Best Practices for Data Collection Standardization
To achieve effective data collection standardization, healthcare organizations should adopt internationally recognized data standards, such as the Logical Observation Identifiers Names and Codes (LOINC) or the Systematized Nomenclature of Medicine - Clinical Terms (SNOMED CT). Furthermore, providing training and education to healthcare professionals on data collection best practices is essential to ensure compliance and data quality.
Regular audits, data validation checks, and ongoing communication with data users are also crucial to address any deviations from data standards and mitigate data quality issues.
Step 4: Training Staff on Data Quality Principles
Ensuring data quality requires the active involvement of healthcare professionals at all levels. Staff training on data quality principles empowers healthcare providers to understand the importance of accurate data and instills a culture that values data integrity and data-driven decision making.
The Role of Staff in Data Quality Management
Healthcare professionals play a vital role in ensuring data quality, as they are directly involved in data collection, documentation, and exchange activities. Staff members need to be aware of the potential consequences of poor data quality and understand their responsibilities in maintaining accurate and consistent data.
By providing comprehensive training on data quality principles, healthcare organizations can equip their staff with the knowledge and skills necessary to promote data accuracy, reliability, and security.
Effective Training Techniques for Data Quality
Training programs should focus on data collection techniques, data entry guidelines, and data coding practices. They should also address the importance of data validation, data cleansing, and the potential risks associated with poor data quality.
Hands-on training, case studies, and real-life examples can help reinforce key concepts and provide practical insights into data quality management. Ongoing education and continuous professional development opportunities are crucial to ensure staff members stay up-to-date with evolving data quality standards and practices.
Conclusion
Improving data quality is a multifaceted endeavor that requires a comprehensive and systematic approach. By following these five steps - understanding the importance of data quality, establishing data governance, implementing data quality management tools, standardizing data collection processes, and training staff on data quality principles - healthcare organizations can enhance the integrity of their data. Ultimately, this will enable better decision making, boost patient outcomes, and drive the transformation of the healthcare industry.
You might also like
Get in Touch to Learn More
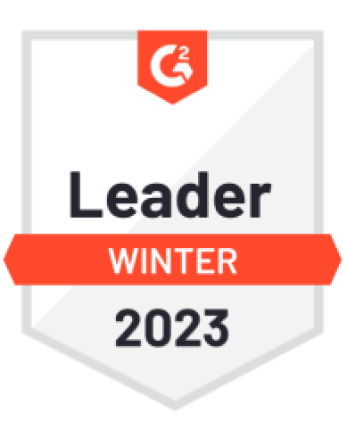
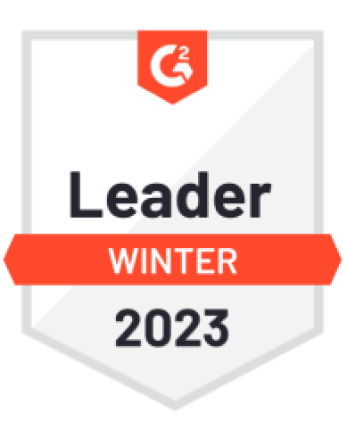

“[I like] The easy to use interface and the speed of finding the relevant assets that you're looking for in your database. I also really enjoy the score given to each table, [which] lets you prioritize the results of your queries by how often certain data is used.” - Michal P., Head of Data