How to resolve effectively Data Quality Issues?
Learn how to effectively assess and resolve data quality issues.
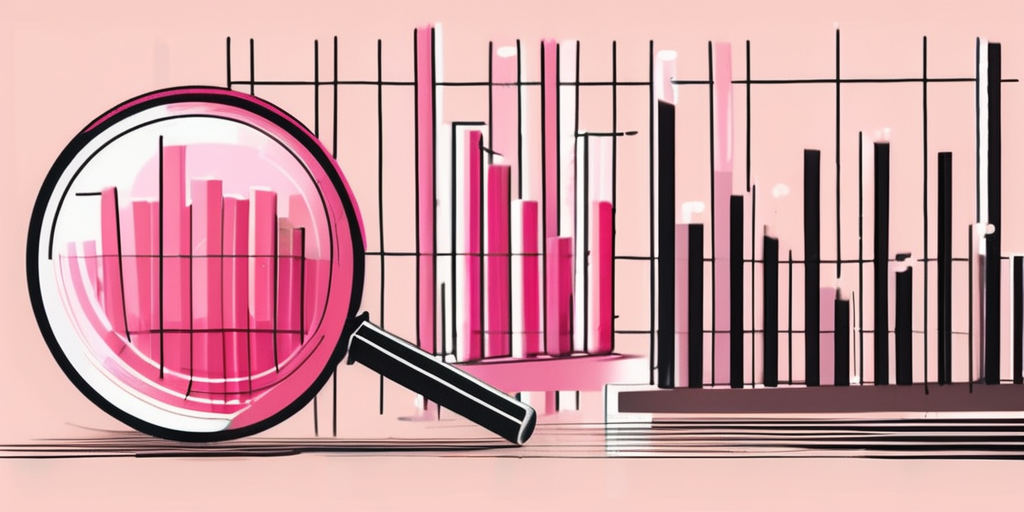
In any organization, the quality of data is of utmost importance. Poor data quality can lead to inaccurate analysis, ineffective decision-making, and ultimately, negative business outcomes. Therefore, it is imperative for businesses to assess and resolve data quality issues effectively. This article explores the steps involved in identifying and rectifying data quality problems to ensure reliable and accurate data.
Understanding Data Quality Issues
Data quality refers to the overall accuracy, completeness, reliability, and consistency of data. It encompasses various factors, including data correctness, timeliness, and relevance. By maintaining high data quality standards, businesses can derive meaningful insights and make informed decisions.
Ensuring data quality is crucial in today's data-driven world, where organizations rely heavily on data to drive strategic initiatives and gain a competitive edge. Poor data quality can lead to costly errors, misleading analyses, and ultimately, poor business outcomes. Therefore, investing in data quality management processes and tools is essential for organizations looking to harness the full potential of their data.
Defining Data Quality
Data quality can be defined as the measure of the fitness of data for a specific purpose. It involves evaluating data based on specific criteria, such as accuracy, precision, consistency, and completeness. By establishing clear data quality standards, organizations can ensure the reliability and usability of their data assets.
Moreover, data quality is not a one-time effort but an ongoing process that requires continuous monitoring, evaluation, and improvement. As data sources and business requirements evolve, organizations must adapt their data quality strategies to maintain high standards and meet changing needs.
Common Data Quality Problems
Data quality issues are prevalent across organizations. Some common problems include inconsistency in data formats, duplicate records, missing values, and outdated information. These issues can arise from various sources, such as data entry errors, system limitations, or inadequate data validation processes.
Addressing data quality problems requires a systematic approach that involves data profiling, cleansing, and enrichment. Organizations can leverage data quality tools and technologies to automate these processes and ensure data accuracy and consistency across their systems.
Furthermore, fostering a data-driven culture within the organization is essential for maintaining data quality standards. By promoting data literacy, training employees on data management best practices, and establishing data governance policies, businesses can instill a mindset of data stewardship and accountability at all levels.
The Importance of High-Quality Data
High-quality data serves as the foundation for accurate analysis and informed decision-making. It significantly impacts various aspects of business operations, including strategic planning, customer satisfaction, and regulatory compliance.
Moreover, high-quality data is crucial for maintaining a competitive edge in today's data-driven business landscape. With the rise of artificial intelligence and machine learning technologies, businesses rely heavily on data to drive innovation, improve operational efficiency, and deliver personalized experiences to customers.
Impact on Business Decisions
Inaccurate or incomplete data can lead to faulty decision-making, resulting in suboptimal outcomes. High-quality data ensures that decisions are based on reliable information, leading to better performance and increased competitiveness. It enables organizations to identify trends, predict future customer behavior, and capitalize on market opportunities.
Furthermore, high-quality data empowers businesses to conduct thorough risk assessments, streamline processes, and allocate resources effectively. By leveraging accurate data, organizations can make proactive decisions that mitigate potential risks and drive sustainable growth.
Role in Customer Satisfaction
Data quality directly affects customer satisfaction levels. Inaccurate customer information can lead to miscommunication, failed marketing campaigns, and a negative brand image. By ensuring data accuracy and completeness, businesses can provide personalized experiences, build trust, and foster long-term customer relationships.
Additionally, high-quality data enables businesses to segment their customer base effectively, target specific demographics with tailored marketing strategies, and anticipate customer needs. This proactive approach not only enhances customer satisfaction but also increases customer loyalty and lifetime value.
Steps to Assess Data Quality
Before resolving data quality issues, it is crucial to assess the extent and impact of the problems. This assessment helps prioritize corrective actions and allocate resources effectively.
Understanding the intricacies of data quality assessment involves delving into various dimensions that contribute to the overall quality of data within an organization. It's not just about identifying errors but also about comprehensively evaluating the reliability and usability of the data in different contexts.
Identifying Data Quality Metrics
Define relevant data quality metrics based on organizational goals and requirements. Metrics can include data accuracy, completeness, consistency, timeliness, and relevance. By quantifying data quality, organizations can measure improvements and track progress over time.
Each data quality metric plays a crucial role in providing a holistic view of the data landscape. Accuracy ensures that the data is correct and free from errors, completeness indicates the presence of all necessary information, consistency highlights uniformity across datasets, timeliness emphasizes the relevance of data within a specific timeframe, and relevance focuses on the significance of data in meeting business objectives.
Implementing Data Quality Audits
Conduct regular data quality audits to systematically evaluate data against defined metrics. These audits can identify inconsistencies, errors, and gaps in data quality, enabling businesses to take appropriate corrective actions. Data quality audits also help identify potential process improvements and strengthen data governance practices.
During data quality audits, it is essential to not only identify issues but also to trace their root causes. This detailed analysis can uncover underlying problems in data collection, processing, or storage, leading to more effective solutions and long-term data quality enhancement strategies.
Techniques to Resolve Data Quality Issues
Once data quality issues are identified, several techniques can be employed to address them effectively.
Ensuring data accuracy and consistency is crucial for any organization that relies on data-driven decision-making. By implementing robust strategies and methodologies, businesses can mitigate the risks associated with poor data quality and enhance the overall reliability of their data assets.
Data Cleansing Methods
Data cleansing involves the identification and correction of inaccurate, incomplete, or duplicated data. Techniques such as standardization, validation, and enrichment are used to ensure data accuracy and consistency. Data cleansing processes can be automated, leveraging algorithms and machine learning, to enhance efficiency and reduce manual efforts.
Standardization involves converting data into a uniform format, making it easier to compare and analyze. Validation techniques help identify anomalies and errors in the data, ensuring that only high-quality data is used for decision-making. Enrichment involves enhancing existing data with additional information from external sources, providing a more comprehensive view of the data.
Data Governance Strategies
Data governance encompasses the policies, processes, and roles that ensure data meets defined quality standards. Establishing robust data governance frameworks helps enforce data quality controls, improve data documentation, and create accountability. By implementing data governance strategies, businesses can prevent data quality issues from arising in the future.
Effective data governance involves defining clear ownership of data, establishing data quality metrics, and implementing data stewardship programs. Data governance frameworks also include data security measures to protect sensitive information and ensure compliance with regulatory requirements. By fostering a culture of data governance within an organization, businesses can proactively address data quality issues and maintain the integrity of their data assets.
Preventing Future Data Quality Issues
While resolving existing data quality issues is crucial, it is equally important to prevent their recurrence in the future. Implementing proactive measures can help maintain a high level of data integrity and reliability.
One key aspect of preventing future data quality issues is to establish a robust data governance framework. This framework outlines the policies, procedures, and standards for managing data across the organization. By clearly defining roles and responsibilities for data management, organizations can ensure accountability and consistency in data quality practices.
Establishing Data Quality Management
Develop a comprehensive data quality management strategy that includes data quality policies, procedures, and responsibilities. This strategy ensures that data quality is a shared responsibility across the organization and establishes clear guidelines for data handling, validation, and maintenance.
Furthermore, organizations should invest in data quality tools and technologies to automate data profiling, cleansing, and monitoring processes. These tools can help streamline data quality management efforts, identify potential issues proactively, and improve overall data accuracy and completeness.
Continuous Monitoring and Improvement
Data quality should be an ongoing process. Implement mechanisms to monitor data quality regularly, detect anomalies, and take corrective actions promptly. By continuously improving data quality practices, organizations can prevent data degradation and maintain high-quality data consistently.
Another effective strategy for preventing future data quality issues is to provide regular training and education to employees on data quality best practices. By raising awareness and building data literacy across the organization, employees can contribute to maintaining data quality standards and identifying potential issues early on.
In conclusion, data quality issues pose significant challenges to organizations, but they can be effectively assessed and resolved. By understanding data quality, recognizing common problems, and implementing appropriate steps, businesses can ensure their data assets are accurate, reliable, and valuable. With high-quality data, organizations can make informed decisions, enhance customer satisfaction, and drive overall business success.
You might also like
Get in Touch to Learn More
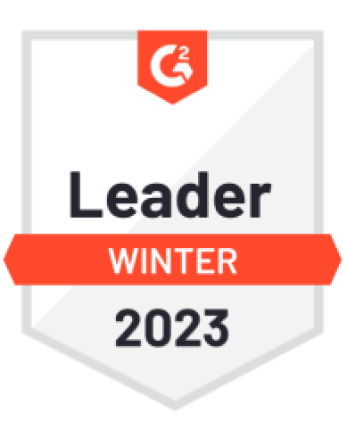
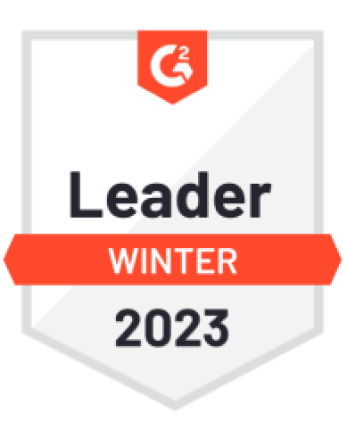

“[I like] The easy to use interface and the speed of finding the relevant assets that you're looking for in your database. I also really enjoy the score given to each table, [which] lets you prioritize the results of your queries by how often certain data is used.” - Michal P., Head of Data