Data Tagging For Snowflake
Discover the power of data tagging for Snowflake.
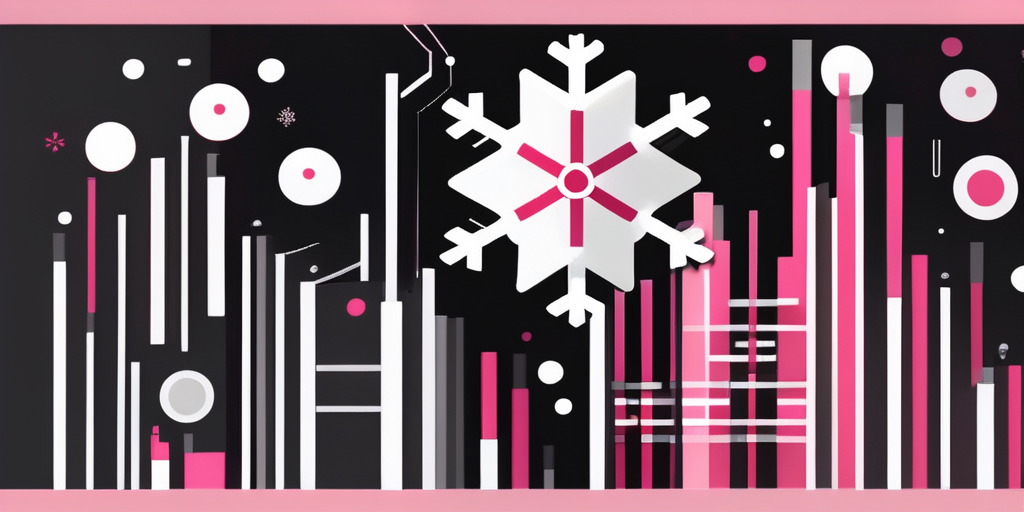
The effective management and organization of data is crucial for organizations seeking to stay competitive. This is where data tagging comes into play. In this article, we will explore the fundamentals of data tagging and how it intersects with Snowflake, a leading cloud data platform. We will delve into the importance of data tagging, highlight the key features of Snowflake, and discuss the benefits of implementing data tagging in Snowflake. Additionally, we will cover the steps to implement data tagging and provide best practices to overcome the challenges associated with it.
Understanding the Basics of Data Tagging
What is Data Tagging?
Data tagging refers to the process of attaching labels or metadata to data sets, allowing for easy categorization and organization. These tags provide valuable context and enable efficient data management, making it easier to locate, understand, and utilize data assets. By assigning specific attributes or characteristics to data, organizations can streamline data governance, enhance data quality, and ensure regulatory compliance.
One key aspect of data tagging is the ability to create custom tags tailored to the unique needs of an organization. These custom tags can include information such as project-specific identifiers, data source details, or even user-defined categories. By leveraging custom tags, organizations can create a more granular and detailed system of data classification, leading to more precise search results and improved data utilization.
Importance of Data Tagging
Data tagging plays a vital role in data management, enabling organizations to reap several benefits. Firstly, it promotes data discoverability and accessibility. By organizing data using tags, users can quickly search for and retrieve relevant information, saving time and effort. Additionally, data tagging enhances data governance by facilitating compliance with regulations and data privacy laws. It helps organizations proactively monitor data usage and ensure that sensitive information is appropriately handled. Finally, data tagging enables better collaboration and enhances data-driven decision-making by providing a clear understanding of the data context for all stakeholders.
Another significant advantage of data tagging is its impact on data analytics and machine learning initiatives. By consistently tagging data with relevant metadata, organizations can improve the accuracy and efficiency of their analytical models. This metadata enrichment enables data scientists to better understand the characteristics and relationships within the data, leading to more precise insights and predictions. Furthermore, data tagging can support automated processes such as data classification and anomaly detection, empowering organizations to leverage advanced analytics techniques for strategic decision-making.
Introduction to Snowflake
Overview of Snowflake
Snowflake is a cloud data platform that revolutionizes the way organizations store, analyze, and share data. Built from the ground up for the cloud, Snowflake offers unmatched flexibility, scalability, and performance. Its architecture separates compute and storage, allowing for independent scaling and efficient resource utilization. Snowflake's cloud-native design makes it ideal for handling large-scale data workloads and enables seamless collaboration across teams and partners.
One of the key aspects that sets Snowflake apart is its automatic scaling capabilities. As data needs fluctuate, Snowflake can automatically adjust compute resources to meet demand, ensuring optimal performance and cost-efficiency. This dynamic scaling feature eliminates the need for manual intervention, allowing organizations to focus on deriving insights from their data rather than managing infrastructure.
Key Features of Snowflake
Snowflake boasts a rich set of features that empower organizations to harness the power of their data. Its unique multi-cluster shared data architecture enables users to access and analyze data concurrently without impacting performance. Snowflake's built-in support for semi-structured data types, such as JSON and XML, allows for the integration of unstructured data sources seamlessly. Moreover, Snowflake's comprehensive security measures, including end-to-end encryption and granular access controls, ensure the confidentiality and integrity of data.
Another standout feature of Snowflake is its data sharing capabilities. Organizations can securely share live data with internal teams or external partners without the need for complex data movement or duplication. This streamlined sharing process not only enhances collaboration but also accelerates decision-making by providing real-time access to up-to-date information. Snowflake's data sharing functionality is a game-changer for organizations looking to break down data silos and foster a culture of data-driven decision-making.
The Intersection of Data Tagging and Snowflake
Why Use Data Tagging in Snowflake?
Snowflake's data tagging capabilities complement its cutting-edge features, making it an ideal platform for implementing data tagging. By utilizing Snowflake's tagging capabilities, organizations can enhance data discoverability within their Snowflake environments. The ability to assign metadata to tables, columns, and other objects in Snowflake allows users to easily navigate and comprehend data assets, paving the way for improved data governance and data-driven decision-making.
The Role of Data Tagging in Snowflake
Data tagging in Snowflake serves as a powerful tool to classify and organize data objects. Tags can be used to highlight the sensitivity, purpose, ownership, or any other relevant attribute of data within Snowflake. By leveraging the tagging feature, organizations can establish data categorization standards, track data lineage, and implement access controls based on specific tag attributes. This ensures that data is appropriately managed, secured, and used in compliance with organizational policies.
Moreover, data tagging in Snowflake contributes to fostering a culture of data transparency and accountability within organizations. By assigning tags to data assets, stakeholders across different departments can easily identify the source, context, and quality of the data they are working with. This transparency not only promotes collaboration and knowledge sharing but also helps in building trust in the data-driven insights derived from Snowflake.
Enhancing Data Governance with Snowflake Data Tagging
When it comes to data governance, Snowflake's data tagging feature plays a crucial role in ensuring regulatory compliance and data integrity. Organizations can use tags to indicate data classification levels, such as public, internal, confidential, or restricted, enabling them to enforce access controls and data protection measures effectively. Additionally, data tagging facilitates the auditing process by providing a clear trail of how data is used, transformed, and shared within Snowflake, aiding in compliance audits and regulatory reporting requirements.
Implementing Data Tagging in Snowflake
Implementing data tagging in Snowflake involves a straightforward set of steps. Firstly, organizations need to define a tagging strategy, mapping out the relevant attributes to be used as tags. Next, tags can be assigned to Snowflake objects such as databases, schemas, tables, and columns using Snowflake's SQL commands and metadata management capabilities. Organizations should also establish processes to maintain and update tags regularly to keep pace with evolving business requirements.
Expanding on the process of defining a tagging strategy, organizations can consider involving stakeholders from different departments to gather diverse perspectives on what attributes should be tagged. This collaborative approach can lead to a more comprehensive tagging strategy that reflects the needs of various teams within the organization. Additionally, conducting a thorough analysis of existing data structures and workflows can help identify key areas where tagging can add the most value.
Best Practices for Data Tagging in Snowflake
To ensure the success of data tagging in Snowflake, it is crucial to follow some best practices. Firstly, organizations should define a clear and consistent tagging taxonomy to maintain uniformity across data assets. Creating a comprehensive tagging policy that aligns with the organization's data governance framework is also essential. Regular audits should be conducted to validate the accuracy and relevancy of tags. Finally, promoting awareness and providing training to users about the importance and proper usage of data tags will drive successful adoption.
Building on the idea of regular audits, organizations can leverage automated tools within Snowflake to schedule periodic tag reviews and validations. These tools can help identify any inconsistencies or missing tags, ensuring that the tagging system remains robust and up to date. Moreover, establishing a feedback loop where users can provide input on the effectiveness of the current tagging system can drive continuous improvement and refinement of the tagging strategy.
Challenges and Solutions in Data Tagging for Snowflake
Common Challenges in Data Tagging
While data tagging offers numerous benefits, organizations often face challenges when implementing and managing it. Lack of standardized tagging practices, inconsistent data classification, and resistance to change are common hurdles. Organizations may also encounter difficulties in integrating existing data classification systems with Snowflake's tagging capabilities. Furthermore, maintaining the accuracy and consistency of tags across a growing volume of data can be a daunting task.
One specific challenge that organizations face is the lack of awareness and understanding about the importance of data tagging. Many employees may not fully grasp the significance of tagging data and how it can improve data management and analysis. This lack of awareness can lead to inconsistent and incomplete tagging practices, making it difficult to derive meaningful insights from the data.
Effective Solutions for Data Tagging Challenges
To overcome the challenges associated with data tagging, organizations should adopt effective solutions. Establishing clear guidelines and governance policies for data tagging will help ensure consistency and accuracy in tagging practices. By providing employees with comprehensive training and education on the importance of data tagging, organizations can foster a culture of data awareness and encourage employees to actively participate in the tagging process.
In addition to education and governance, integrating automated tools and processes to classify and tag data can significantly streamline the tagging process and reduce human error. These tools can automatically scan and analyze data, applying appropriate tags based on predefined rules and criteria. This not only saves time and effort but also improves the accuracy and consistency of tagging practices.
Furthermore, regular monitoring and periodic audits of data tags help maintain data integrity and resolve any discrepancies promptly. By conducting regular reviews of tagged data, organizations can identify and rectify any inconsistencies or inaccuracies, ensuring that the tags remain up to date and reliable.
Expanding on the benefits of data tagging, organizations can leverage tagged data to enhance data governance and compliance efforts. By implementing robust data tagging practices, organizations can easily identify and track sensitive or regulated data, ensuring that it is handled and protected appropriately. This can help organizations meet regulatory requirements and maintain data privacy and security standards.
In conclusion, data tagging in Snowflake empowers organizations to effectively manage and utilize their data assets. By understanding the basics of data tagging and exploring its intersection with Snowflake, organizations can create a data-driven environment that enhances data governance, promotes collaboration, and enables informed decision-making. With the right implementation strategies and best practices, organizations can overcome the challenges associated with data tagging and unlock the full potential of Snowflake's cloud data platform.
Ready to elevate your data tagging strategy within Snowflake and harness the full power of your data? Look no further than CastorDoc. With its advanced governance, cataloging, and lineage capabilities, paired with a user-friendly AI assistant, CastorDoc is the ultimate tool for enabling self-service analytics. Whether you're a data professional seeking to streamline data governance or a business user aiming to make data more accessible, CastorDoc's conversational interface and natural language processing will transform the way you interact with data. Don't miss the opportunity to revolutionize your data management and drive informed decision-making. Try CastorDoc today and unlock the potential of your data assets.
You might also like
Get in Touch to Learn More
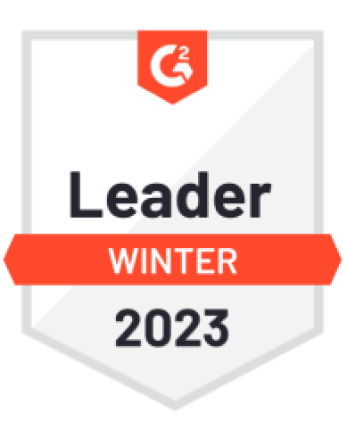
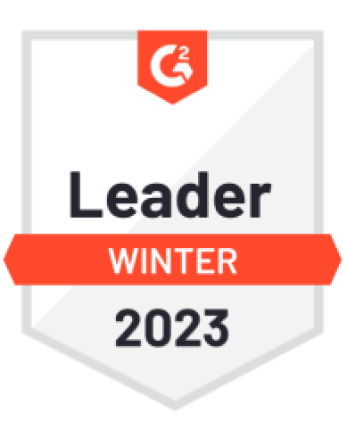

“[I like] The easy to use interface and the speed of finding the relevant assets that you're looking for in your database. I also really enjoy the score given to each table, [which] lets you prioritize the results of your queries by how often certain data is used.” - Michal P., Head of Data