Data Vs Metadata: 4 Key Differences, Examples & Common challenges
Discover the essential disparities between data and metadata in this piece
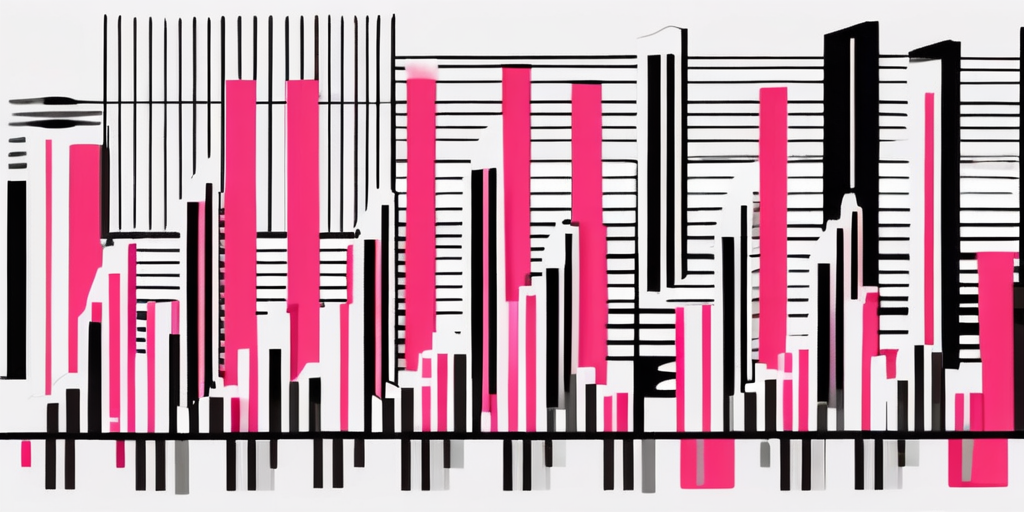
Organizations are inundated with an overwhelming amount of information. To navigate this data landscape effectively, it is crucial to understand the differences between two fundamental concepts: data and metadata. While the terms may sound similar, they serve distinct purposes and have unique characteristics. In this article, we will delve into the basics of data and metadata, explore their key differences, examine their roles in different industries, and address the common challenges associated with managing them.
Understanding the Basics: Data and Metadata
Defining Data: An Overview
Data refers to raw facts, observations, or measurements that represent real-world entities. It can take various forms, such as text, numbers, images, audio, and video. Data is the foundation upon which information and knowledge are built. It can be captured, stored, processed, and analyzed to derive insights and make informed decisions.
When we delve deeper into the realm of data, we uncover its significance in various fields such as business, science, healthcare, and technology. In the business world, data plays a crucial role in market analysis, customer segmentation, and trend forecasting. In scientific research, data is essential for experimentation, hypothesis testing, and drawing conclusions. Healthcare professionals rely on data for patient diagnosis, treatment planning, and medical research. Moreover, the rapid advancements in technology have led to the generation of massive amounts of data, giving rise to big data analytics and artificial intelligence applications.
Defining Metadata: An Overview
Metadata, on the other hand, refers to data about data. It provides context and details about the characteristics, attributes, and properties of the underlying data. Metadata helps in understanding the meaning, structure, and quality of data. It facilitates data discovery, integration, interpretation, and management.
Metadata serves as the backbone of data organization and governance. By describing the content, context, and structure of data, metadata enables efficient data retrieval, sharing, and reuse. In addition, metadata standards and frameworks ensure consistency and interoperability across different systems and platforms. For example, in the realm of digital libraries, metadata schemas like Dublin Core and MARC (Machine-Readable Cataloging) are used to categorize and index resources for easy access and retrieval. Furthermore, metadata plays a crucial role in data security and privacy by defining access controls, data lineage, and provenance information.
The Four Key Differences Between Data and Metadata
Difference 1: Purpose and Function
The primary purpose of data is to represent and capture information. It is the tangible representation of real-world entities or events. For example, in the context of a retail business, data can include customer names, purchase history, and product details. This data is crucial for analyzing customer behavior, identifying trends, and making informed business decisions.
On the other hand, metadata serves as a means to describe, organize, and manage data. It enhances data understanding, accessibility, and interoperability by providing additional context and structure. Metadata can include information about the source of the data, its format, creation date, and any relevant relationships with other data. It acts as a guide for users, helping them navigate and interpret the underlying data.
Difference 2: Structure and Composition
Data typically exhibits a diverse range of structures and compositions depending on its type and format. For example, structured data is organized in predefined formats such as databases, spreadsheets, or XML files. This structured format allows for efficient storage, retrieval, and analysis of data. Unstructured data, on the other hand, lacks a predefined structure and can include text documents, images, videos, and social media posts. Analyzing unstructured data requires advanced techniques such as natural language processing and image recognition.
In contrast, metadata often follows predefined schemas or standards, enabling consistency and interoperability across different systems and applications. These schemas define the attributes and properties that metadata should contain. For instance, the Dublin Core Metadata Initiative provides a widely used set of metadata elements for describing digital resources. Adhering to such standards ensures that metadata can be understood and utilized by different systems, facilitating data integration and exchange.
Difference 3: Usage and Application
Data is utilized for various purposes, including analysis, reporting, decision-making, and automation. It forms the basis for business intelligence, machine learning, and artificial intelligence. For example, data analysis can help businesses identify patterns and trends, enabling them to optimize their operations and improve customer satisfaction. Machine learning algorithms rely on vast amounts of data to train models and make predictions.
Conversely, metadata primarily aids in the management, governance, and understanding of data. It enables efficient data retrieval, categorization, and navigation. Metadata can include information about the data's origin, ownership, and usage rights. This allows organizations to enforce data governance policies, ensuring compliance with regulations and protecting sensitive information. Metadata also helps users discover relevant data by providing search capabilities and facilitating data integration across different systems.
Difference 4: Accessibility and Control
Data is often accessible to end-users and applications for manipulation and processing. It can be stored in databases, file systems, or cloud-based repositories. Access controls can be implemented to ensure that only authorized users can view or modify the data. Additionally, data can be replicated and distributed across multiple locations for redundancy and availability.
In contrast, metadata is typically hidden or managed within systems or repositories. It enables administrators and data stewards to control access, update, and maintain the integrity of data. For example, metadata can include information about data ownership, data quality, and data lineage. This allows organizations to establish data governance practices and ensure that metadata remains accurate and up-to-date. By controlling access to metadata, organizations can enforce data privacy policies and protect sensitive information.
The Role of Data and Metadata in Different Industries
The Impact of Data and Metadata in Healthcare
In the healthcare industry, data plays a pivotal role in patient care, diagnosis, treatment, and research. Electronic health records, medical imaging data, and clinical trial data are just a few examples of healthcare data. Metadata helps in standardizing medical terminologies, ensuring compliance with regulations, and improving health outcomes through data integration and interoperability.
Furthermore, data and metadata in healthcare are also instrumental in advancing precision medicine initiatives. By analyzing genetic data, treatment outcomes, and patient demographics, healthcare providers can tailor personalized treatment plans for individuals based on their unique genetic makeup and medical history. This targeted approach not only enhances patient outcomes but also contributes to the overall efficiency of healthcare delivery systems.
The Role of Data and Metadata in E-commerce
In the realm of e-commerce, data is essential for understanding customer behavior, personalizing shopping experiences, and optimizing supply chain operations. Product catalogs, customer reviews, and transactional data are prime examples of e-commerce data. Metadata assists in categorizing products, managing inventory, analyzing sales patterns, and enhancing search capabilities on e-commerce platforms.
Moreover, data and metadata in e-commerce are increasingly being leveraged for dynamic pricing strategies. By analyzing market trends, competitor pricing, and customer demand patterns in real-time, e-commerce businesses can adjust their pricing strategies to maximize profitability and maintain a competitive edge in the market. This data-driven approach not only benefits businesses but also enhances the overall shopping experience for consumers.
Data and Metadata in the Field of Education
Data and metadata are transforming the landscape of education by improving teaching methods, student performance analysis, and curriculum development. Student assessment data, learning analytics, and educational content repositories are key educational data sources. Metadata helps in aligning learning objectives, tracking learner progress, and fostering collaboration among educators.
Furthermore, data and metadata in education are also revolutionizing personalized learning experiences. By analyzing student engagement metrics, learning preferences, and academic performance data, educators can tailor instructional content and teaching strategies to meet the individual needs of students. This data-driven approach not only enhances student learning outcomes but also promotes a more inclusive and adaptive educational environment.
Common Challenges in Managing Data and Metadata
Challenge 1: Data Quality and Consistency
Ensuring data quality and consistency is a persistent challenge for organizations. Data may be incomplete, outdated, or inaccurate, leading to erroneous insights and decisions. Metadata can help address this challenge by providing information about data provenance, lineage, and quality constraints.
For example, let's consider a scenario where a retail company is analyzing customer data to identify trends and preferences. If the data is inconsistent or contains errors, the company may make incorrect assumptions about customer behavior, leading to ineffective marketing strategies. By leveraging metadata that includes details about data sources, data quality checks, and data transformation processes, the company can ensure that the insights derived from the data are reliable and accurate.
Challenge 2: Metadata Standardization
Metadata often comes from various sources and may lack standardization. Inconsistent metadata formats, definitions, and terminology can hinder data integration and interoperability. Establishing and adhering to metadata standards and best practices can mitigate this challenge and promote seamless data exchange and collaboration.
Consider a scenario where two departments within an organization are working on a joint project that requires sharing and integrating data. If each department uses different metadata formats and definitions, it can lead to confusion and misinterpretation of the data. By implementing standardized metadata practices, such as using common data dictionaries and agreed-upon terminology, the departments can ensure that the data integration process is smooth and accurate, enabling effective collaboration and decision-making.
Challenge 3: Data Security and Privacy
With the proliferation of data breaches and privacy concerns, protecting sensitive data has become a critical challenge. Organizations must adhere to privacy regulations, encrypt data, and implement access controls. Metadata plays a vital role in managing permissions, classifying data sensitivity levels, and enforcing data governance policies.
Let's take the example of a healthcare organization that handles patients' medical records. Ensuring the privacy and security of this sensitive data is of utmost importance. By leveraging metadata, the organization can classify the data based on its sensitivity level, such as personal identifiable information (PII) or protected health information (PHI). This classification enables the organization to implement appropriate access controls and encryption measures, ensuring that only authorized individuals can access the data, thus safeguarding patient privacy.
Challenge 4: Metadata Management and Governance
Managing metadata throughout its lifecycle is a complex task. Metadata needs to be captured, stored, organized, and updated systematically. Metadata governance ensures that metadata is accurate, trustworthy, and aligned with business objectives. Implementing metadata management frameworks and tools can streamline this process.
Consider a large financial institution that deals with vast amounts of financial data. To effectively manage this data, the institution needs to have a robust metadata management system in place. This system should capture metadata about data sources, data transformations, and business rules. By implementing a metadata management framework, the institution can ensure that the metadata is up to date, accurate, and aligned with regulatory requirements. This, in turn, enables the institution to make informed decisions based on reliable and trustworthy data.
In conclusion, data and metadata are integral components of the modern information landscape. Understanding their differences, significance, and challenges is crucial for organizations aiming to harness the power of data-driven insights. By effectively managing data and metadata, organizations can unlock tremendous value, drive innovation, and gain a competitive edge in today's data-driven world.
You might also like
Get in Touch to Learn More
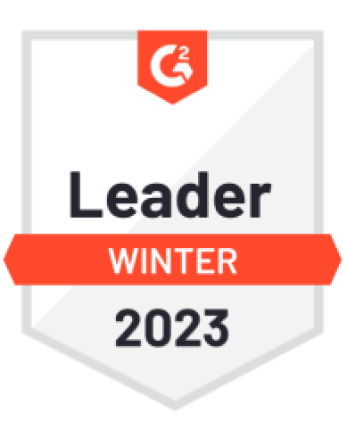
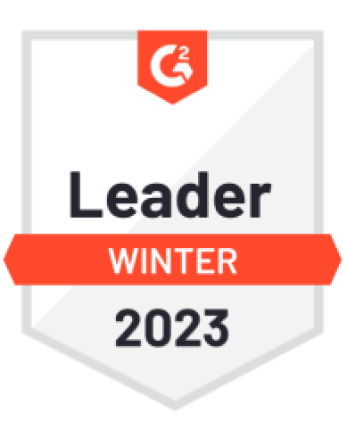

“[I like] The easy to use interface and the speed of finding the relevant assets that you're looking for in your database. I also really enjoy the score given to each table, [which] lets you prioritize the results of your queries by how often certain data is used.” - Michal P., Head of Data