Databook: Uber’s In-house Metadata Catalog Powering Scalable Data Discovery
Discover how Uber's in-house metadata catalog is revolutionizing data discovery and scalability.

Today, managing and extracting value from vast amounts of information is a critical challenge faced by companies across industries. Uber, the global ride-sharing giant, is no exception. With millions of users and a complex data ecosystem, Uber needs a robust and scalable solution for data discovery and management. Enter Databook, Uber's in-house metadata catalog that serves as the linchpin of their data infrastructure.
Understanding the Concept of Metadata Catalog
Before diving into the specifics of Databook, let's first grasp the concept of a metadata catalog. Metadata, simply put, is data about data. It provides essential information such as the origin, characteristics, and relationships of datasets. A metadata catalog acts as a centralized repository where this information is stored and organized, allowing users to easily discover, understand, and utilize data assets.
Metadata catalogs are crucial components of modern data management systems, serving as the backbone for effective data governance and stewardship. They not only store technical details about datasets but also capture business context, ownership information, and usage guidelines. This holistic approach to metadata management ensures that data is not only accessible but also trustworthy and compliant with regulatory requirements.
The Role of Metadata in Data Management
In the realm of data management, metadata plays a pivotal role. It enables efficient data governance, ensures data lineage and traceability, and supports data quality assurance. By providing insights into the context and structure of data, metadata empowers data professionals to make informed decisions, enhances collaboration, and boosts overall data reliability.
Moreover, metadata facilitates data integration and interoperability across different systems and platforms. By standardizing metadata definitions and formats, organizations can break down data silos, improve data sharing, and enable seamless data exchange between disparate applications and databases.
Defining Metadata Catalog
A metadata catalog serves as a comprehensive inventory of an organization's data assets. It encompasses metadata related to various types of data, including structured and unstructured. A robust metadata catalog should be flexible, scalable, and easily searchable, enabling users to quickly find and understand the datasets they need.
Furthermore, metadata catalogs support data discovery and promote data reuse within an organization. By providing detailed descriptions, tags, and relationships between datasets, users can identify relevant data sources, understand their content, and leverage existing data assets for new projects and initiatives. This not only saves time and resources but also encourages a culture of data-driven decision-making and innovation across the organization.
The Birth of Databook at Uber
Recognizing the immense value of metadata in their data-driven business, Uber set out to develop Databook, their in-house metadata catalog. The journey began with a deep analysis of their data ecosystem, identifying pain points and potential areas of improvement.
As Uber delved deeper into the realm of metadata management, they uncovered a treasure trove of insights waiting to be harnessed. The realization dawned that metadata held the key to unlocking hidden patterns, optimizing processes, and driving innovation within the organization.
Identifying the Need for an In-house Metadata Catalog
Uber's data ecosystem had grown rapidly and organically, resulting in data silos and fragmentation. Locating and understanding datasets became challenging, hampering their data-driven decision-making processes. Recognizing the need for a centralized metadata catalog that would enable seamless data discovery, Uber embarked on the development of Databook.
The quest for a solution to the metadata maze led Uber to envision Databook as more than just a catalog; it was to be a beacon of clarity in the sea of data ambiguity. By centralizing metadata management, Uber aimed to empower their teams with the ability to navigate the data landscape with ease and confidence, fostering a culture of informed decision-making and data-driven innovation.
The Development Process of Databook
The development of Databook involved a cross-functional team of data engineers, data scientists, and product managers. The team leveraged state-of-the-art technologies and designed Databook as a scalable, cloud-native solution. Continuous feedback loops and agile methodologies ensured that Databook remained aligned with the evolving needs of Uber's data ecosystem.
Collaboration was at the heart of Databook's development, with each team member bringing their unique expertise to the table. From designing intuitive user interfaces to optimizing backend infrastructure, every aspect of Databook was meticulously crafted to deliver a seamless and enriching experience for Uber's data practitioners. The journey of Databook's creation was not just about building a tool; it was a testament to Uber's commitment to harnessing the power of metadata to drive their data-driven future forward.
Key Features of Databook
Databook offers a plethora of features that empower Uber's data professionals in their quest for scalable data discovery and management. Let's explore some of its key functionalities.
When it comes to data discovery and search, Databook truly shines. It not only enables users to explore Uber's vast array of datasets through intuitive search capabilities but also provides advanced filtering options. Users can narrow down their search results based on data creation date, last modified date, or even data size. This level of granularity in search functionality enhances the user experience and ensures that data professionals can quickly find the exact datasets they need.
Data Discovery and Search
Databook enables users to explore Uber's vast array of datasets through intuitive search capabilities. By leveraging metadata, users can search by dataset name, attributes, or even keywords related to the data. This streamlined approach fosters efficiency and reduces the time spent on manual data exploration.
Moreover, Databook goes beyond traditional search functionality by offering personalized recommendations to users based on their search history and preferences. By leveraging machine learning algorithms, Databook can suggest relevant datasets that users may have overlooked, further enhancing the data discovery process.
Data Lineage and Relationships
Understanding the lineage and relationships between datasets is crucial for data professionals at Uber. Databook provides a clear and visual representation of data lineage, showcasing the path from raw data to derived datasets. Additionally, it reveals the interdependencies between datasets, enabling users to navigate complex data relationships with ease.
Furthermore, Databook allows users to annotate data lineage diagrams with additional information, such as data transformation steps or data source details. This feature not only enhances the documentation of data lineage but also promotes collaboration among data professionals by providing a centralized platform for sharing insights and knowledge about data relationships.
Data Quality and Compliance
Data quality and compliance are paramount in Uber's data-centric operations. Databook incorporates features to ensure data integrity and compliance with regulatory requirements. It allows users to track and assess the quality of datasets, flagging any potential anomalies or data quality issues. This proactive approach to data governance enhances Uber's ability to maintain high data standards.
In addition to data quality monitoring, Databook includes built-in compliance checks that automatically scan datasets for adherence to internal data policies and external regulations. By providing real-time feedback on compliance status, Databook empowers data professionals to take immediate action to rectify any compliance issues, ensuring that Uber's data practices remain transparent and in line with industry standards.
The Impact of Databook on Uber's Data Infrastructure
Since its implementation, Databook has revolutionized Uber's data infrastructure, driving significant improvements in data discovery, management, and governance.
Enhancing Scalability in Data Discovery
Prior to Databook, data discovery at Uber was a time-consuming process that involved manual exploration and data wrangling. With Databook's powerful search capabilities and intuitive interface, data professionals can now effortlessly discover and access the datasets they need, leading to greater efficiency and scalability in data discovery.
Streamlining Data Management Processes
Databook acts as a centralized hub for data assets, streamlining the management and maintenance of datasets. The clear visibility provided by Databook's metadata catalog simplifies the task of data documentation, reducing redundancy and ensuring data consistency. This streamlined approach to data management optimizes resource allocation and promotes collaboration across teams.
Improving Data Quality and Compliance
Databook's data quality assessment features have had a profound impact on Uber's data operations. The ability to track and monitor data quality in real-time enables data professionals to identify and rectify issues promptly. By ensuring data integrity and compliance, Databook strengthens Uber's reputation as a trusted provider of reliable and secure data.
The Future of Databook and Metadata Catalogs
Databook is just the tip of the iceberg when it comes to the potential of metadata catalogs in the realm of Big Data. As technology advances and data-driven decision-making becomes even more integral to businesses, the importance of metadata catalogs will continue to grow.
Potential Enhancements for Databook
Uber's data professionals are constantly exploring ways to enhance Databook to meet the evolving needs of their data ecosystem. Some potential enhancements include the integration of advanced AI algorithms for automated data classification and enrichment. Additionally, enhanced collaboration features and seamless integration with other data management tools are on the roadmap for future development.
The Growing Importance of Metadata Catalogs in Big Data
As organizations grapple with managing and extracting insights from ever-increasing volumes of data, metadata catalogs are becoming a critical component of their data infrastructure. Metadata catalogs empower data professionals to efficiently discover, understand, and utilize data assets, catalyzing data-driven decision-making and organizational growth.
In conclusion, Databook has emerged as a game-changer for Uber's data infrastructure, boosting scalability in data discovery, streamlining data management processes, and improving data quality and compliance. As organizations continue to harness the power of Big Data, the role of metadata catalogs like Databook will only become more significant. By embracing and investing in metadata catalogs, companies can unlock the full potential of their data assets and propel themselves towards a data-driven future.
You might also like
Get in Touch to Learn More
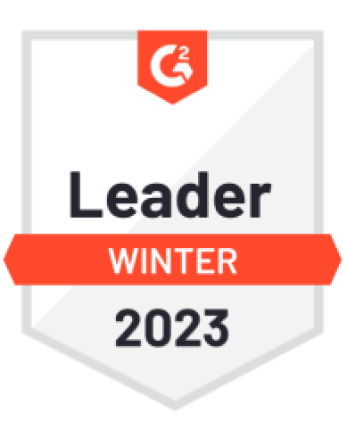
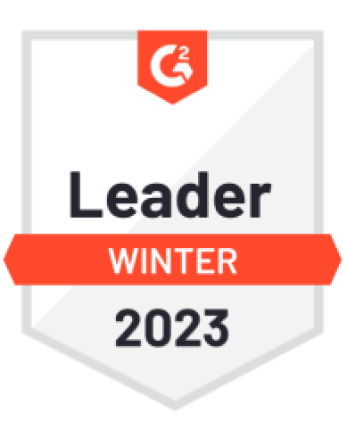

“[I like] The easy to use interface and the speed of finding the relevant assets that you're looking for in your database. I also really enjoy the score given to each table, [which] lets you prioritize the results of your queries by how often certain data is used.” - Michal P., Head of Data