Enterprise Semantic Layer: Building a Company-Wide Data Understanding Framework
Discover how an Enterprise Semantic Layer can revolutionize your organization's data strategy by creating a unified framework for data understanding.

Understanding the Concept of Enterprise Semantic Layer
The Enterprise Semantic Layer serves as an intermediary between complex data sets and end users. This layer abstracts the underlying data structure and provides a user-friendly interface to interact with the data. By implementing an ESL, organizations empower their teams to leverage business intelligence tools more effectively, enabling insights that drive decision-making.
Defining Enterprise Semantic Layer
An Enterprise Semantic Layer is essentially a framework that enables consistent and unified data interpretation across various systems. It translates technical database schemas into business-friendly terms. This process involves mapping the data in a way that reflects the terminology and needs of the business users, making it accessible even to those without technical backgrounds.
Through the Enterprise Semantic Layer, employees can access data without having to possess deep technical knowledge of the underlying databases. It creates a common language between IT and business teams, facilitating better collaboration and understanding.
Importance of a Semantic Layer in Business
In a data-driven world, the ease with which organizations can harness their data for strategic planning and operational efficiency is paramount. The Enterprise Semantic Layer eliminates silos that often exist between departments and fosters a culture of data accessibility. This enhancement in data utilization can significantly boost an organization's agility, innovation, and competitive edge.
Moreover, by standardizing the definitions and metrics used across the enterprise, the Enterprise Semantic Layer reduces confusion and discrepancies within reports. This alignment ensures that the same terms mean the same thing across different departments, ultimately leading to a more coherent business strategy grounded in reliable data.
Additionally, the Enterprise Semantic Layer can play a crucial role in enhancing data governance. By providing a clear framework for data definitions and usage, organizations can better manage compliance with regulations such as GDPR or HIPAA. This governance not only protects sensitive information but also builds trust among stakeholders, who can be assured that data is handled responsibly and ethically.
Furthermore, as organizations increasingly adopt advanced analytics and machine learning, the Enterprise Semantic Layer becomes even more vital. It allows for the seamless integration of these technologies by ensuring that the data fed into algorithms is consistent and accurately reflects business realities. This capability not only improves the quality of insights generated but also accelerates the time to value for data-driven initiatives, making the Enterprise Semantic Layer an indispensable asset in the modern enterprise landscape.
Components of an Enterprise Semantic Layer
Building an effective Enterprise Semantic Layer requires an understanding of its key components, which collectively play a crucial role in ensuring that data is both accessible and usable across the organization.
Data Catalog
A robust data catalog serves as the foundation of the Enterprise Semantic Layer. It is a structured inventory of all the data assets within an organization. A comprehensive data catalog not only provides a detailed description of the datasets but also includes metadata that offers insights into data lineage, ownership, and usage.
By maintaining an up-to-date data catalog, organizations can ensure that users have access to accurate and relevant data. This feature also promotes data discovery, enabling users to quickly find the information they need without dredging through multiple data sources. Furthermore, a well-implemented data catalog can facilitate collaboration among teams by providing a common understanding of data terminology and definitions, thereby reducing misunderstandings and enhancing communication across departments.
Data Governance
Data governance is critical in maintaining the integrity and security of the data within the ESL. It involves establishing policies, processes, and standards to ensure that data is managed consistently and responsibly throughout the organization.
With a strong data governance framework, organizations can enforce data quality standards, regulate access based on user roles, and ensure compliance with legal and regulatory requirements. This governance framework builds trust among users, empowering them to rely on the data provided by the ESL for their decision-making processes. Additionally, effective data governance can help mitigate risks associated with data breaches and misuse, ultimately safeguarding the organization’s reputation and fostering a culture of accountability regarding data management.
Metadata Management
Metadata management entails the organization and management of data about other data. This includes definitions, relationships, and usage instructions for various data elements within the ESL. Effective metadata management ensures that users can understand the context and nuances of the data they are accessing.
By providing detailed metadata, organizations enhance data comprehension, making it easier for users to engage with the data in a meaningful way. This clarity can lead to more informed decision-making and a deeper understanding of data-driven insights. Moreover, metadata management can also support advanced analytics initiatives by enabling data scientists and analysts to quickly identify relevant datasets and understand their interdependencies, thus accelerating the process of deriving actionable insights from complex data landscapes.
Steps to Build a Company-Wide Data Understanding Framework
Constructing an Enterprise Semantic Layer is a strategic process that involves several crucial steps. Each step requires thorough planning and collaboration across departments to ensure a successful implementation.
Identifying Business Needs
The first step in building an Enterprise Semantic Layer is identifying the specific business needs of various stakeholders within the organization. Engaging with diverse teams—including marketing, finance, operations, and IT—enables a comprehensive understanding of the types of data insights they require.
Once the business needs are identified, organizations can prioritize which data sources and metrics are most critical. This step ensures that the ESL is tailored to meet the demands of the business effectively, facilitating a focused and streamlined approach to data utilization. Additionally, it is vital to consider the future growth and evolving needs of the business. By anticipating potential shifts in strategy or market conditions, organizations can create a more resilient framework that adapts over time, ensuring long-term value from the Enterprise Semantic Layer.
Selecting the Right Tools
Choosing the right tools for your Enterprise Semantic Layer is a pivotal step. A variety of tools are available that support data modeling, cataloging, and governance. Selecting tools that align with business needs and existing infrastructure can greatly enhance the efficiency of the Enterprise Semantic Layer.
Organizations should also consider the scalability and ease of use of these tools, as they will significantly influence user adoption across the organization. Involving end users in the selection process can lead to better outcomes and ensure the tools meet their practical needs. Furthermore, it is beneficial to evaluate the integration capabilities of these tools with other systems already in place. This can prevent potential data silos and ensure a seamless flow of information across platforms, ultimately enhancing the overall data ecosystem within the organization.
Implementing the Semantic Layer
The implementation of the Enterprise Semantic Layer involves integrating the chosen tools with existing data sources and establishing workflows for data management. This process should be iterative, with regular feedback loops from stakeholders to ensure alignment with business objectives.
Training and resources should also accompany the implementation phase. Providing support to users will help them transition smoothly to the new system and maximize their ability to leverage the Enterprise Semantic Layer for data-driven decisions. Additionally, fostering a culture of data literacy within the organization is essential. Encouraging ongoing education and workshops can empower employees at all levels to engage with data confidently, enabling them to extract valuable insights and contribute to a more data-driven decision-making process across the enterprise.
Challenges in Building an Enterprise Semantic Layer
While the benefits of an Enterprise Semantic Layer are significant, organizations may encounter challenges during its development and implementation. Recognizing these early on can facilitate smoother transitions.
Data Quality Issues
Data quality is one of the most significant challenges in establishing an Enterprise Semantic Layer. Inaccurate, incomplete, or outdated data can lead to misleading insights, eroding trust in the Enterprise Semantic Layer. Therefore, organizations must prioritize data cleansing and validation before implementing the ESL.
Ongoing data quality monitoring should also be established to maintain the integrity of the data as new information is continuously added. This proactive approach ensures that decision-makers can rely on accurate, high-quality data at all times.
Integration Challenges
Integration challenges arise when attempting to connect disparate data sources, each with its unique structure and technology. Broken or poorly managed integration processes can lead to delays and inconsistencies in data availability.
Utilizing standardized protocols and leveraging tools designed for data integration can mitigate these challenges. Establishing a clear data integration strategy will streamline the connections needed to maintain a robust ESL.
The Role of AI and Machine Learning in Semantic Layers
Artificial Intelligence (AI) and Machine Learning (ML) technologies contribute significantly to enhancing the capabilities of an Enterprise Semantic Layer. Their integration can lead to improved data insights and efficiencies, further enriching the data understanding framework.
Enhancing Data Discovery
AI and ML algorithms can analyze large datasets, identifying patterns and relationships that may not be immediately evident to users. This capability enhances data discovery within the Enterprise Semantic Layer, allowing users to uncover valuable insights more easily.
By employing advanced analytics techniques, businesses can empower users to ask more complex questions and receive more nuanced answers. This transformation elevates the entire data experience, driving deeper engagement with the data assets available.
Improving Data Quality
The application of AI and ML technologies can also enhance data quality management within the ESL. These technologies can automatically flag data anomalies, suggest corrections, and predict potential data quality issues before they arise.
As a result, organizations can maintain a higher standard of data quality, ensuring that the insights generated across the enterprise are grounded in reliable and accurate information.
In conclusion, building an Enterprise Semantic Layer is a strategic endeavor that fosters a comprehensive understanding of data across the entire organization. By leveraging the right components, addressing challenges head-on, and integrating advanced technologies, companies can create a robust framework that drives innovation and data-driven decision-making.
Ready to elevate your organization's data management and analytics capabilities? Look no further than CastorDoc. With its advanced governance, cataloging, and lineage features, combined with a user-friendly AI assistant, CastorDoc is the ideal solution for businesses seeking to implement a powerful Enterprise Semantic Layer. Whether you're a data professional aiming for comprehensive control and visibility or a business user desiring accessible and understandable data, CastorDoc caters to all your needs. Embrace the future of self-service analytics and unlock the full potential of your data. Try CastorDoc today and experience the revolution in data management and decision-making.
You might also like
Get in Touch to Learn More
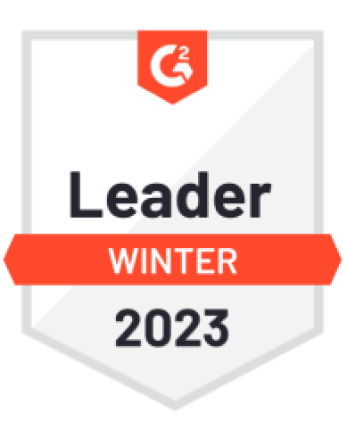
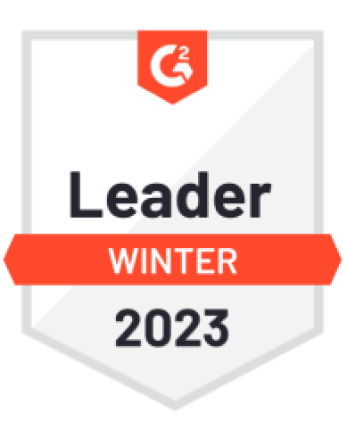

“[I like] The easy to use interface and the speed of finding the relevant assets that you're looking for in your database. I also really enjoy the score given to each table, [which] lets you prioritize the results of your queries by how often certain data is used.” - Michal P., Head of Data