How to Build a Robust Data Quality Integrity Framework?
Discover the essential steps and best practices for creating a comprehensive data quality integrity framework.
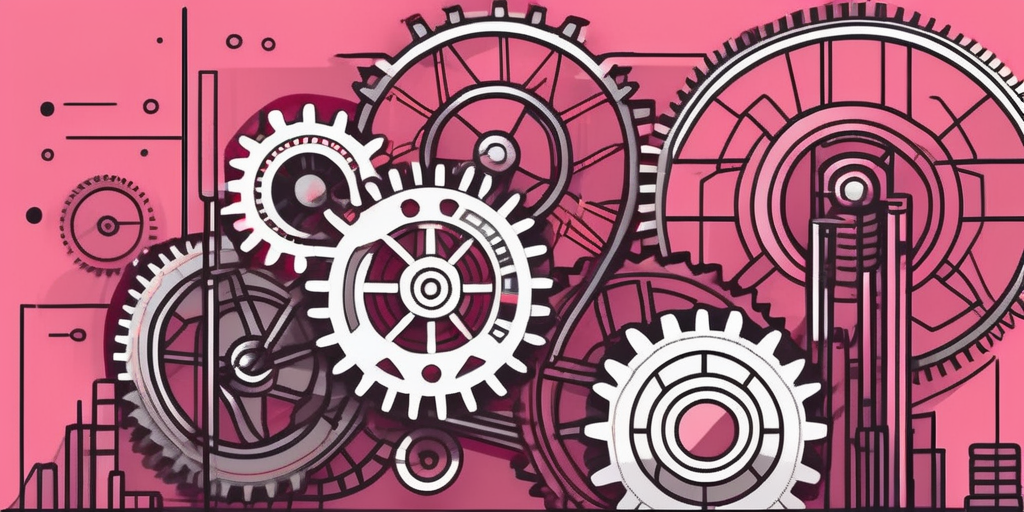
Today, organizations rely heavily on the accuracy and reliability of their data to make informed business decisions. However, data is only valuable if it is of high quality and integrity. Building a robust data quality integrity framework is essential to ensure the consistency, accuracy, and reliability of data within an organization. In this article, we will explore the importance of data quality integrity, the key components of a robust framework, the steps to build it, and the challenges that may arise in the process.
Understanding the Importance of Data Quality Integrity
Data quality integrity refers to the overall trustworthiness and reliability of data. It encompasses various aspects, such as accuracy, completeness, consistency, and timeliness. Organizations that prioritize data quality integrity can make better business decisions, improve operational efficiency, and enhance customer satisfaction.
Defining Data Quality Integrity
Before diving deeper, let's clarify what we mean by data quality integrity. Data quality integrity is the assurance that data is complete, accurate, consistent, and up-to-date throughout its lifecycle. It involves establishing and adhering to data governance principles, implementing robust data quality management processes, and ensuring data security and privacy.
Why is Data Quality Integrity Crucial?
Ensuring data quality integrity is crucial for several reasons. Firstly, high-quality and reliable data empowers organizations to make informed business decisions. By having trustworthy data at their disposal, executives can gain valuable insights, identify trends, and detect potential issues early on.
Moreover, data quality integrity is essential for compliance with regulatory requirements. Organizations operating in industries such as healthcare, finance, or telecommunications must adhere to strict data protection and privacy regulations. Failing to ensure data quality integrity can result in severe penalties and damage to reputation.
Lastly, data quality integrity is vital for building and maintaining customer trust. Inaccurate or inconsistent data can lead to mistakes in customer communications, improper billing, or incorrect product recommendations. By ensuring data quality integrity, organizations can deliver personalized and relevant experiences to their customers, thereby enhancing satisfaction and loyalty.
Furthermore, data quality integrity plays a significant role in data analytics and machine learning. When organizations have reliable and high-quality data, they can train their machine learning models more effectively, leading to more accurate predictions and insights. This, in turn, enables organizations to optimize their operations, improve customer targeting, and drive innovation.
Additionally, data quality integrity is crucial for data integration and data sharing initiatives. In today's interconnected world, organizations often need to exchange data with partners, suppliers, or customers. Ensuring data quality integrity becomes paramount to avoid misinterpretation, miscommunication, or errors in data integration processes.
In conclusion, data quality integrity is a critical aspect of any organization's data management strategy. By prioritizing data quality integrity, organizations can unlock the full potential of their data, make informed decisions, comply with regulations, build customer trust, and drive innovation. It is an ongoing effort that requires continuous monitoring, improvement, and collaboration across different departments and stakeholders.
Key Components of a Robust Data Quality Integrity Framework
Building a robust data quality integrity framework involves considering various key components. Let's explore three essential elements that form the foundation of such a framework.
Data Governance
Data governance is the foundation of any successful data quality integrity framework. It involves establishing policies, procedures, and standards for data management within an organization. A robust data governance framework ensures that data is collected, stored, processed, and shared consistently and securely across the organization.
Effective data governance also includes defining roles and responsibilities for data stewardship, establishing data quality KPIs, and ensuring compliance with regulatory requirements such as GDPR or HIPAA. By implementing a comprehensive data governance framework, organizations can foster a data-driven culture and improve decision-making processes based on accurate and reliable data.
Data Quality Management
Data quality management focuses on ensuring the accuracy, completeness, consistency, and timeliness of data. It involves defining data quality metrics, conducting data profiling and cleansing activities, and implementing data quality controls. By effectively managing data quality, organizations can identify and resolve issues, improving the overall integrity of their data.
Furthermore, data quality management encompasses data lineage tracking, metadata management, and establishing data quality monitoring processes. These practices enable organizations to trace the origins of data, understand data transformations, and proactively detect anomalies or discrepancies that could impact data integrity.
Data Security and Privacy
Data security and privacy are critical components of a data quality integrity framework. Organizations must implement appropriate security measures to protect data from unauthorized access and ensure compliance with privacy regulations. By safeguarding data against security breaches and unauthorized use, organizations can maintain data integrity and protect sensitive information.
In addition to implementing encryption, access controls, and data masking techniques, organizations should conduct regular security audits, penetration testing, and privacy impact assessments. These proactive measures help mitigate security risks, enhance data protection mechanisms, and build trust with customers and stakeholders regarding data privacy practices.
Steps to Building a Data Quality Integrity Framework
Building a robust data quality integrity framework involves a series of well-defined steps. Let's delve into each step to understand the process thoroughly.
Establishing the Data Quality Vision and Goals
The first step in building a data quality integrity framework is to establish a clear vision and set achievable goals. This involves defining the desired state of data quality integrity within the organization and identifying the specific objectives to be accomplished.
For example, a healthcare organization may have a vision of achieving 100% accuracy in patient records and a goal of reducing data errors by 50% within the next year. By clearly defining the vision and goals, organizations can align their efforts and resources towards improving data quality.
Designing the Data Quality Strategy
Once the vision and goals are established, organizations need to design a comprehensive data quality strategy. This strategy should outline the approach, processes, and tools that will be used to achieve the desired data quality integrity. It should also consider data governance principles, data quality management practices, and data security requirements.
For instance, the data quality strategy may involve implementing data profiling techniques to identify data anomalies, establishing data cleansing processes to remove duplicate or inaccurate data, and implementing data validation rules to ensure data consistency.
Implementing the Data Quality Tools and Processes
After designing the strategy, the next step is to implement the data quality tools and processes. This involves selecting and deploying appropriate data quality management tools, establishing data quality monitoring mechanisms, and defining roles and responsibilities within the organization.
For example, organizations may choose to invest in data quality software that provides features such as data profiling, data cleansing, and data validation. They may also establish data quality monitoring mechanisms, such as regular data audits and data quality scorecards, to ensure that data integrity is maintained over time.
Monitoring and Improving the Data Quality Framework
Building a data quality integrity framework is an ongoing process that requires continuous monitoring and improvement. Organizations should regularly assess the performance of the framework, measure data quality metrics, and identify areas for improvement. By monitoring and improving the framework, organizations can adapt to evolving data requirements and ensure the long-term integrity of their data.
For instance, organizations may conduct periodic data quality assessments to measure the accuracy, completeness, and consistency of their data. Based on the results of these assessments, they can identify areas where data quality can be improved and take appropriate actions, such as providing additional training to data stewards or implementing data quality improvement initiatives.
By following these steps and continuously refining their data quality integrity framework, organizations can ensure that their data is reliable, accurate, and trustworthy, enabling them to make informed decisions and drive business success.
Challenges in Building a Data Quality Integrity Framework
While building a data quality integrity framework is crucial, it is not without its challenges. Let's explore some common hurdles that organizations may face in this process.
Data Complexity and Volume
Modern organizations deal with increasingly complex and voluminous data. With the advent of big data and the Internet of Things (IoT), managing and ensuring the quality of extensive datasets can be overwhelming. Organizations must invest in scalable infrastructure, implement advanced analytics techniques, and leverage automation to overcome these challenges.
Lack of Data Governance
The absence of a robust data governance framework can hinder the establishment of a data quality integrity framework. Without clear policies, procedures, and standards, organizations may struggle to ensure consistent data management practices across the organization. It is crucial to prioritize data governance and establish a clear governance structure within the organization.
Technology and Infrastructure Challenges
Implementing an effective data quality integrity framework requires advanced technology infrastructure and tools. Organizations may face challenges in procuring and implementing suitable data quality management tools, integrating disparate data sources, and managing technological complexities. It is essential to assess technology requirements and invest in the right infrastructure to support the framework.
Conclusion
A robust data quality integrity framework is indispensable for organizations seeking to harness the power of data. By understanding the importance of data quality integrity and considering key components such as data governance, data quality management, and data security, organizations can lay a strong foundation for their framework. By following the steps of establishing a vision, designing a strategy, implementing tools, and monitoring the framework, organizations can ensure long-term data integrity. However, it is important to be aware of challenges such as data complexity, lack of governance, and technology limitations, and proactively address them to build a successful framework. By building a robust data quality integrity framework, organizations can unlock the full potential of their data and gain a competitive edge in today's data-driven landscape.
You might also like
Get in Touch to Learn More
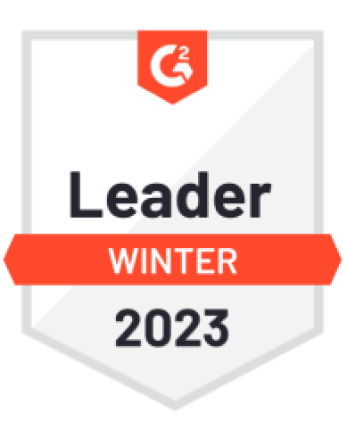
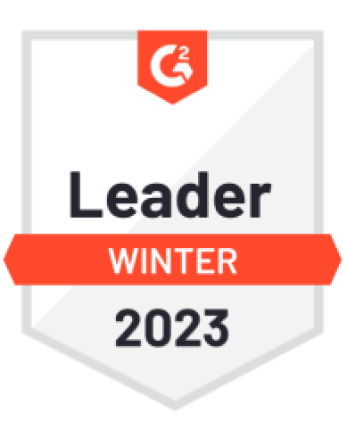

“[I like] The easy to use interface and the speed of finding the relevant assets that you're looking for in your database. I also really enjoy the score given to each table, [which] lets you prioritize the results of your queries by how often certain data is used.” - Michal P., Head of Data