How to Ensure Optimal Data Quality to Enhance Business Performance
Discover effective strategies for maintaining high data quality and boosting business performance.
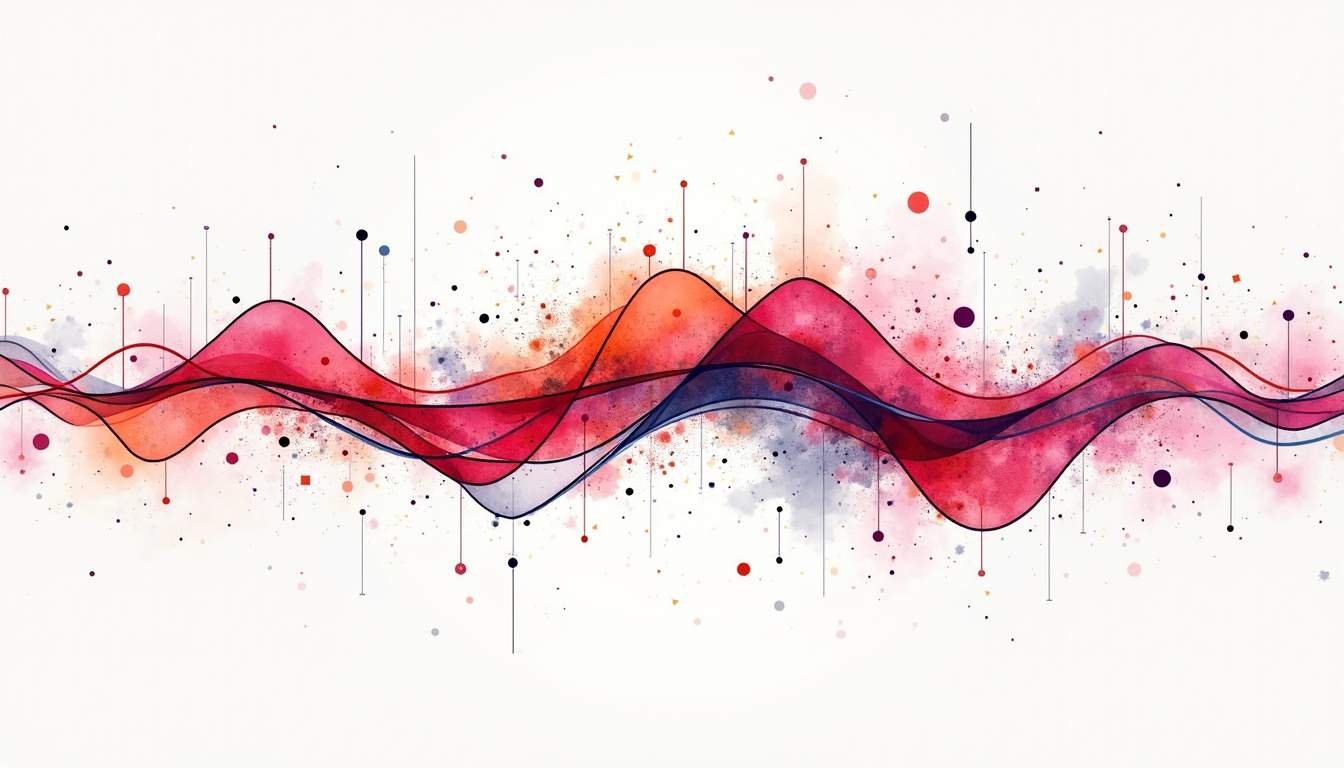
In today's data-driven world, businesses rely heavily on accurate, reliable, and timely data to make informed decisions, enhance operational efficiency, and ultimately drive growth. However, the presence of data quality issues can undermine these efforts, affecting decision-making processes and strategic initiatives. This article explores the importance of data quality and outlines actionable strategies to ensure data integrity, thereby enhancing overall business performance.
Understanding the Importance of Data Quality
Data quality is a crucial aspect of any organization’s information management strategy. High-quality data allows for better decision-making, operational efficiency, and customer satisfaction. Conversely, poor data quality can lead to costly errors, misinformed decisions, and a negative impact on business reputation. As organizations increasingly rely on data-driven strategies, the emphasis on maintaining high standards of data quality becomes even more pronounced. In a world where data is often referred to as the new oil, the integrity and reliability of this data can significantly influence an organization's competitive edge.
Defining Data Quality
Data quality is characterized by several dimensions including accuracy, completeness, consistency, timeliness, uniqueness, and validity. Each of these dimensions plays a vital role in determining the reliability of the data being managed. For instance, accurate data reflects the correct facts and figures, while complete data ensures that no essential information is missing. Understanding these dimensions helps organizations assess their data quality effectively. Furthermore, organizations can implement various data quality frameworks and tools to continuously monitor and improve these dimensions, ensuring that their data remains a valuable asset rather than a liability.
The Role of Data Quality in Business Performance
Quality data directly correlates with business performance. Organizations with high data quality are better equipped to analyze market trends, understand customer behaviors, and make strategic decisions. This, in turn, leads to improved operational efficiency, higher revenue, and increased customer loyalty. Additionally, businesses that prioritize data quality are more likely to comply with regulations and avoid costly penalties associated with data mishandling. Moreover, the integration of advanced analytics and machine learning technologies further amplifies the need for high-quality data, as these tools rely on accurate and reliable datasets to generate meaningful insights. In this context, investing in data quality not only enhances immediate business outcomes but also lays the groundwork for future innovations and growth opportunities.
Strategies for Ensuring Optimal Data Quality
To maintain high data quality, organizations must adopt comprehensive strategies tailored to their specific needs. Here are several essential strategies that can significantly improve data quality.
Implementing Data Governance
Data governance is the foundation for ensuring data quality across an organization. This involves establishing policies, procedures, and standards that dictate how data is managed, processed, and utilized. Assigning data stewards or custodians who are responsible for overseeing data quality initiatives can foster accountability. Data governance also involves documenting data lineage, which helps in tracing data from its origin to its eventual destination, thus ensuring its reliability. Furthermore, a well-defined governance framework promotes a culture of data stewardship within the organization, encouraging employees to take ownership of the data they handle. Regular training and updates on governance policies can keep the team informed about best practices and emerging trends in data management.
Utilizing Data Quality Tools
There are numerous data quality tools available that can help organizations automate the process of monitoring and improving data quality. These tools typically offer functionalities such as data profiling, data cleansing, and duplicate detection. By utilizing these technologies, businesses can effectively identify and rectify data quality issues before they escalate, thereby maintaining data integrity throughout its lifecycle. Additionally, integrating these tools with existing data management systems can streamline workflows and enhance collaboration among teams. Organizations should also consider leveraging machine learning algorithms that can adapt and improve over time, providing increasingly accurate insights into data quality trends and anomalies.
Regular Data Audits and Cleansing
Conducting regular data audits is imperative for identifying inconsistencies and inaccuracies within data sets. Scheduled audits can reveal patterns or trends that indicate underlying data quality issues. Following audits, data cleansing processes should be implemented to correct errors and remove redundant information. This practice not only enhances the quality of the data but also reassures stakeholders of its reliability. Moreover, involving cross-functional teams in the audit process can provide diverse perspectives, ensuring that all potential data quality issues are addressed. Establishing a feedback loop where audit findings are communicated back to data owners can further enhance accountability and foster a proactive approach to data management.
Overcoming Common Data Quality Challenges
Organizations often face various challenges in maintaining data quality. Addressing these challenges requires a proactive approach combined with effective solutions tailored to specific issues. The landscape of data management is constantly evolving, and as organizations grow and adapt, the complexity of their data environments increases. This makes it essential to not only recognize these challenges but also to develop a culture of data stewardship within the organization, where every team member understands the importance of data integrity and quality.
Dealing with Incomplete or Inaccurate Data
Incomplete or inaccurate data can originate from multiple sources, including human errors during data entry or system migration issues. To mitigate these problems, organizations should establish strict data entry protocols, offer training for personnel, and implement validation rules that flag data entry errors in real time. Moreover, regular audits will help identify gaps that need to be addressed. Leveraging technology such as automated data cleansing tools can also significantly enhance the accuracy of data. These tools can identify duplicates, correct formatting issues, and even suggest missing information based on historical patterns, thus reducing the manual workload and enhancing data reliability.
Managing Data Consistency
Data consistency is vital for maintaining a single source of truth. Inconsistencies can occur when data is collected from various sources or systems without appropriate standardization. To ensure consistency, organizations should adopt standardized data formats and nomenclature across all systems. Implementing a centralized data repository also helps maintain uniformity and reduces the likelihood of discrepancies. Furthermore, fostering collaboration between departments can enhance data sharing practices, ensuring that all teams are aligned on data definitions and metrics. Regular training sessions and workshops can reinforce the importance of consistency, encouraging employees to take ownership of their data contributions.
Addressing Data Security and Privacy Concerns
With data security and privacy becoming increasingly significant, organizations must incorporate strategies that protect sensitive information while maintaining data quality. Implementing strict access controls, data encryption, and regular security audits can help safeguard data integrity. Additionally, compliance with regulations such as GDPR or CCPA is essential for protecting personal data and ensuring ethical data management practices. Organizations should also consider adopting a data governance framework that outlines clear policies and procedures for data handling. This framework can include guidelines for data classification, retention, and disposal, ensuring that sensitive information is managed appropriately throughout its lifecycle. Engaging employees in data security training can further enhance awareness and vigilance, creating a culture of accountability around data privacy and protection.
Measuring the Impact of Data Quality on Business Performance
To understand the relationship between data quality and business performance, it is essential to establish key performance indicators (KPIs) that measure the effectiveness of data quality initiatives.
Key Performance Indicators for Data Quality
Key performance indicators serve as valuable metrics for assessing data quality. Organizations can track metrics such as the percentage of data entries free from errors, the frequency of data access issues, and the time taken to resolve data discrepancies. Regularly monitoring these KPIs allows businesses to evaluate the effectiveness of their data quality strategies and make informed decisions moving forward.
The Correlation between Data Quality and Business Success
Studies have shown a strong correlation between high data quality and business success. Organizations that prioritize data quality often experience enhanced operational efficiency, reduced costs associated with data errors, and improved customer satisfaction. Additionally, by leveraging quality data for strategic initiatives, businesses can achieve better market positioning and drive growth.
Future Trends in Data Quality Management
The field of data quality management is constantly evolving. Keeping abreast of future trends is crucial for organizations aiming to enhance their data quality practices.
The Rise of Artificial Intelligence in Data Management
Artificial Intelligence (AI) introduces new opportunities for improving data quality through automation. AI-powered tools can analyze vast datasets, identify anomalies, and suggest corrective actions much faster than manual methods. As AI technology continues to advance, its integration into data governance and quality management systems will further enhance data reliability and efficiency.
The Growing Importance of Real-Time Data Quality Control
In an era where real-time data is essential for quick decision-making, organizations are increasingly focusing on improving real-time data quality control. This practice enables businesses to maintain accurate and up-to-date data throughout their operations. By adopting technologies that facilitate real-time monitoring and cleansing, organizations can respond promptly to data quality issues and ensure that their data remains reliable and actionable.
In conclusion, ensuring optimal data quality is not just a technical requirement but a strategic necessity for enhancing business performance. By understanding its importance, implementing robust strategies, overcoming challenges, measuring impact, and staying updated with future trends, organizations can successfully leverage data as a competitive advantage. The pursuit of data quality will ultimately lead to better decision-making, improved operational efficiency, and sustained business growth.
Ready to elevate your business performance with impeccable data quality? CastorDoc is your ideal partner in this journey. With its advanced governance, cataloging, and lineage capabilities, coupled with a user-friendly AI assistant, CastorDoc is the powerful tool your business needs to enable self-service analytics and ensure data integrity. Whether you're a data professional seeking control and visibility or a business user desiring accessible, understandable data, CastorDoc is designed to meet your needs. Try CastorDoc today and unlock the full potential of your data to drive informed decision-making across your enterprise.
You might also like
Get in Touch to Learn More
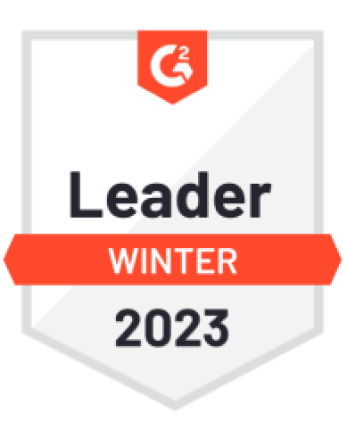
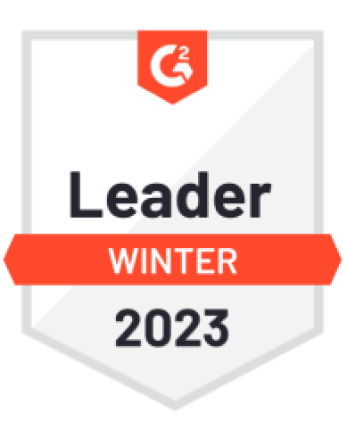

“[I like] The easy to use interface and the speed of finding the relevant assets that you're looking for in your database. I also really enjoy the score given to each table, [which] lets you prioritize the results of your queries by how often certain data is used.” - Michal P., Head of Data