How to Fix Your Data Quality Issues: 6 Proven Solutions!
Learn how to tackle your data quality issues with these 6 proven solutions! From data cleansing to implementing quality control measures, this article will guide you through the steps to ensure accurate and reliable data for your business.

Maintaining high-quality data is essential for businesses to make informed decisions and provide excellent customer service. However, many organizations struggle with data quality issues, which can have far-reaching consequences. In this article, we will explore the importance of data quality and present six proven solutions to help you fix your data quality issues effectively.
Understanding Data Quality Issues
Data quality refers to the accuracy, consistency, completeness, and relevance of data. High-quality data is vital for reliable analysis and decision-making, as it forms the basis of strategic business initiatives. Unfortunately, organizations often encounter common data quality problems that hinder their ability to achieve optimal results.
Ensuring data quality is an ongoing process that requires a combination of technology, processes, and people. Organizations must implement data governance frameworks to define policies, procedures, and responsibilities for managing data quality. Data quality tools can help automate processes such as data profiling, cleansing, and monitoring to maintain high standards.
The Importance of High-Quality Data
High-quality data is crucial for organizations to gain insights into customer behavior, identify trends, and make informed business decisions. With accurate and reliable data, companies can develop targeted marketing campaigns, personalize customer experiences, and optimize operational processes.
Moreover, high-quality data enhances regulatory compliance efforts by ensuring that organizations have accurate and up-to-date information for reporting purposes. It also fosters trust among stakeholders, including customers, partners, and investors, who rely on data-driven insights to engage with the organization.
Common Data Quality Problems
Several common data quality problems plague organizations. These include duplicate records, inconsistent data formats, outdated information, and missing values. These issues can stem from various sources, including human error, incomplete data entry, and system integration problems.
Addressing data quality problems requires a multi-faceted approach that involves data profiling to identify issues, data cleansing to correct errors, and data enrichment to fill in missing information. Organizations must also establish data quality metrics and KPIs to monitor the effectiveness of their data quality initiatives and continuously improve their data management processes.
The Impact of Poor Data Quality
Poor data quality can have severe consequences for businesses, impacting various areas of operations and decision-making processes.
One significant consequence of poor data quality is the potential for increased operational costs. When data is inaccurate or incomplete, businesses may need to invest additional resources in correcting errors, conducting manual data validation, and addressing customer complaints. These added expenses can strain budgets and hinder overall efficiency.
Effects on Business Decision Making
When data quality is compromised, decision-makers may rely on inaccurate or incomplete information, leading to flawed decisions. This can result in wasted resources, missed opportunities, and decreased competitiveness. It is critical to address data quality issues to ensure that important decisions are based on reliable data.
Furthermore, poor data quality can also erode trust in the decision-making process. Stakeholders may become skeptical of the data-driven insights presented to them, leading to a lack of confidence in the organization's strategic direction. Building and maintaining trust through accurate and reliable data is essential for fostering a culture of informed decision-making.
Consequences for Customer Relationships
Poor data quality can also have a detrimental impact on customer relationships. Inaccurate customer information can lead to ineffective communication, wrong marketing targeting, and decreased customer satisfaction. It is essential to maintain accurate customer data to provide personalized experiences and foster strong relationships.
In addition to customer relationships, poor data quality can also harm regulatory compliance efforts. Inaccurate data can lead to violations of data protection laws, privacy regulations, and industry standards. Non-compliance can result in hefty fines, legal repercussions, and reputational damage. Ensuring data accuracy and integrity is crucial for meeting regulatory requirements and safeguarding the organization's reputation.
Solution 1: Implementing Data Governance
Data governance is a comprehensive approach to managing and controlling an organization's data assets. By implementing data governance practices, companies can establish policies, procedures, and guidelines for data management throughout the organization.
One crucial aspect of data governance is the establishment of data stewards within the organization. These individuals are responsible for overseeing the implementation of data governance policies and ensuring that data is managed effectively and in compliance with regulations. Data stewards play a key role in promoting a data-driven culture within the organization.
Defining Data Governance
Data governance involves the creation of a governance framework, which defines roles, responsibilities, and processes for managing data. This framework ensures accountability, assigns ownership, and establishes clear guidelines for data collection, storage, and usage.
Another important component of data governance is data quality management. This involves implementing processes and tools to monitor and improve the quality of data within the organization. By ensuring that data is accurate, consistent, and reliable, companies can make more informed decisions and drive business growth.
Benefits of Data Governance
Implementing data governance provides several benefits. It ensures data consistency and accuracy across the organization, improves data security and privacy, enhances regulatory compliance, and enables better decision-making based on reliable data.
Furthermore, data governance can help organizations build trust with their customers and stakeholders. By demonstrating a commitment to data integrity and privacy, companies can enhance their reputation and foster stronger relationships with key stakeholders. This trust can lead to increased customer loyalty and support sustainable business growth in the long term.
Solution 2: Regular Data Audits
Conducting regular data audits is an effective way to identify and rectify data quality issues. Data audits involve a comprehensive review of data assets to assess their quality, integrity, and adherence to established standards.
Regular data audits are like health check-ups for an organization's data ecosystem. Just as routine check-ups help individuals maintain their well-being, data audits ensure that an organization's data remains healthy and reliable. These audits not only help in identifying and resolving existing data issues but also in preventing potential problems that could arise in the future.
The Role of Data Audits
Data audits play a crucial role in identifying data inconsistencies, duplicates, and other quality issues. By conducting audits regularly, organizations can proactively address data quality problems, ensure data accuracy, and maintain reliable insights.
Moreover, data audits are instrumental in fostering a data-driven culture within an organization. By emphasizing the importance of data quality and integrity, audits encourage employees to value accurate information and make informed decisions based on reliable data.
How to Conduct a Data Audit
Conducting a data audit involves several steps. First, define the objectives of the audit and identify the data attributes to assess. Next, collect and analyze the data, comparing it against established data quality standards. Finally, document the findings and develop an action plan to address any identified issues.
It is essential to involve stakeholders from various departments in the data audit process to ensure a comprehensive evaluation. By engaging individuals who work closely with the data on a daily basis, organizations can gain valuable insights and perspectives that contribute to a more thorough audit and effective resolution of data quality issues.
Solution 3: Data Cleansing Techniques
Data cleansing, also known as data scrubbing or data cleaning, is the process of identifying and correcting or removing inaccuracies, inconsistencies, and duplicates from a database or dataset.
What is Data Cleansing?
Data cleansing techniques involve various methods, such as data validation, standardization, normalization, and deduplication. These techniques help ensure data accuracy, completeness, and consistency.
Data validation is a crucial step in the data cleansing process. It involves checking data for accuracy and reliability, ensuring that it meets specific criteria or standards. Standardization, on the other hand, focuses on converting data into a consistent format for easier analysis and comparison. Normalization is the process of organizing data in a database to reduce redundancy and improve data integrity. Deduplication identifies and eliminates duplicate records, streamlining the database and improving overall data quality.
Effective Data Cleansing Strategies
When implementing data cleansing, several strategies can be employed, depending on the nature and extent of data quality issues. These strategies may include automated cleansing tools, manual data validation processes, and establishing data quality metrics to measure ongoing improvements.
Automated data cleansing tools can help organizations streamline the process by automatically detecting and correcting errors in large datasets. Manual data validation processes involve human intervention to review and correct data inconsistencies that automated tools may have missed. Establishing data quality metrics allows organizations to track the effectiveness of their data cleansing efforts over time, ensuring continuous improvement in data quality.
By applying the above-mentioned proven solutions, organizations can effectively address their data quality issues and unlock the true potential of their data. Understanding the importance of high-quality data, the impact of poor data quality, and implementing data governance, regular data audits, and data cleansing techniques will pave the way for better decision-making, improved customer relationships, and increased overall business success.
You might also like
Get in Touch to Learn More
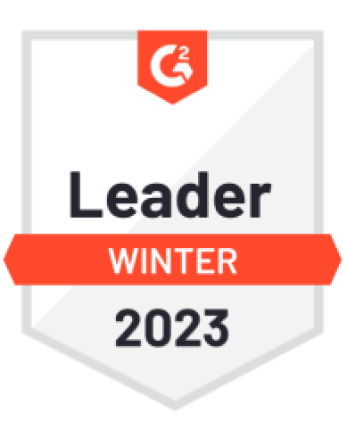
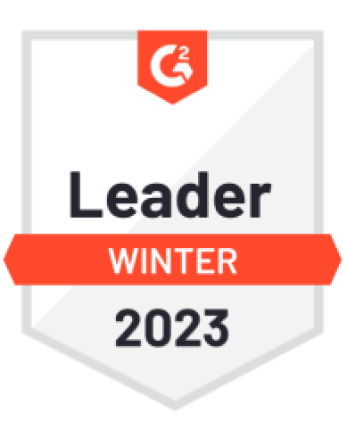

“[I like] The easy to use interface and the speed of finding the relevant assets that you're looking for in your database. I also really enjoy the score given to each table, [which] lets you prioritize the results of your queries by how often certain data is used.” - Michal P., Head of Data