How to launch a Data Cleaning Project?
Discover the essential steps to successfully launch a data cleaning project.

Understanding the Importance of Data Cleaning
Data cleaning is a critical process that ensures the reliability and accuracy of the data that organizations rely on for decision-making. Businesses are increasingly recognizing that the integrity of their data directly impacts their performance and strategic initiatives. Accurate data is not merely a luxury but a necessity for effective analytics and informed decision-making.
Organizations that emphasize data cleaning are better positioned to adapt to changing market conditions, improve operational efficiency, and enhance customer experiences. Clean data provides a robust foundation for analyses, leading to actionable insights that can drive growth and optimization across various departments. Furthermore, as organizations increasingly leverage advanced technologies such as artificial intelligence and machine learning, the quality of the input data becomes even more critical. Poor-quality data can lead to flawed models and misguided strategies, ultimately hindering innovation and competitive advantage.
Defining Data Cleaning
Data cleaning, often referred to as data cleansing or data scrubbing, involves identifying and correcting errors, inconsistencies, and inaccuracies within datasets. This process encompasses several activities, including removing duplicates, correcting misspellings, standardizing formats, and validating data against external sources.
The goal of data cleaning is to ensure that datasets are not only accurate but also relevant and complete for the intended analyses. Facilities without thorough data cleaning processes may find themselves making decisions based on flawed information, potentially leading to costly mistakes. Moreover, the rise of big data has made the cleaning process more complex, as organizations now grapple with vast amounts of information from diverse sources. This complexity necessitates sophisticated tools and methodologies to effectively manage and clean data, ensuring that it remains a valuable asset rather than a liability.
Benefits of a Clean Data Set
A clean dataset enhances an organization’s ability to gain critical insights. The benefits include improved decision-making, reduced operational costs, and greater compliance with regulations. Moreover, a clean dataset can lead to increased customer satisfaction by enabling personalized experiences based on accurate data.
Additionally, businesses that maintain clean data are likely to experience fewer errors in processes reliant on that data, whether they pertain to inventory management, financial reporting, or customer relationship management. Ultimately, investing in data cleaning can yield significant returns by enhancing overall business performance. Furthermore, clean data fosters a culture of trust within the organization, as employees can rely on the information at hand to make informed decisions. This trust not only boosts morale but also encourages collaboration across departments, as teams are more willing to share insights and strategies when they are confident in the underlying data. As a result, organizations can cultivate a more agile and responsive approach to market demands and customer needs.
Preparing for Your Data Cleaning Project
Preparing for a data cleaning project involves several preliminary steps that set the foundation for a successful outcome. This phase not only encompasses understanding your data but also involves planning and stakeholder engagement to ensure the project aligns with organizational goals.
Engaging stakeholders early can facilitate a smoother process, guaranteeing you have the necessary support and resources for undertaking your data cleaning project. Clarity in objectives is key to ensuring that the project delivers the required value. Moreover, involving stakeholders from various departments can provide diverse perspectives that enrich the project, highlighting potential issues that may not have been immediately apparent.
Identifying Your Data Sources
The first step in preparation is to identify all relevant data sources. Understanding where your data resides—whether in databases, spreadsheets, or cloud-based platforms—is crucial. Accurate identification helps in assessing the types and volumes of data that require cleaning, guiding your subsequent actions effectively.
Collaborating with data owners and IT teams can provide deeper insights into the data sources. This collaboration ensures that you capture all necessary information, including legacy systems which may house critical data. Furthermore, acknowledging where data originates can assist in anticipating potential challenges in the cleaning process. It is also beneficial to conduct a preliminary assessment of data quality at this stage, allowing you to gauge the extent of the issues you may face, such as inconsistencies, missing values, or outdated information.
Setting Clear Objectives
Defining clear objectives serves as the roadmap for your data cleaning project. Objectives should be specific, measurable, achievable, relevant, and time-bound (SMART). For example, an objective might be to reduce duplicate records by 80% within two months.
Setting milestones allows for periodic review of progress and adjustments of strategies as needed. These objectives will also guide your team’s efforts, ensuring that everyone is aligned with the project's goals and timelines, ultimately facilitating a more structured and organized cleaning process. Additionally, it can be advantageous to document the rationale behind each objective, as this not only provides context for the team but also serves as a reference point for future projects, helping to build a culture of data quality awareness within the organization.
Key Steps in a Data Cleaning Project
Executing a data cleaning project involves several key steps that collectively facilitate comprehensive data remediation. Each step is integral to achieving the ultimate goal of pristine data, and attention to detail at each stage can maximize the effectiveness of your efforts.
To ensure consistency and quality, it's essential to follow a systematic approach, allowing for measured adjustments based on findings throughout the process.
Data Auditing
The initial step in the execution phase is data auditing. This involves thoroughly evaluating the datasets to identify issues such as duplicates, errors, and formatting inconsistencies. Auditing provides a clear picture of existing data quality levels and pinpoint areas requiring attention.
Information gleaned from the audit phase can also guide the prioritization of cleaning activities. By understanding the severity and prevalence of data quality issues, you can allocate your resources more effectively for higher impact cleaning efforts.
Workflow Specification
Defining the workflow and processes to be followed during the data cleaning project is crucial. This includes outlining roles and responsibilities, determining the sequence of cleaning activities, and specifying the tools and techniques to be employed.
Establishing a clear workflow minimizes confusion and enhances collaboration among team members, ultimately leading to increased efficiency. Documenting the workflow also facilitates training and onboarding of new team members as they can refer to established processes when engaged in data maintenance efforts.
Data Cleaning Process
The heart of a data cleaning project lies in the actual cleaning process. This step involves applying the defined techniques to rectify the identified issues. The process may include removing duplicate records, filling in missing values, standardizing formats, and conducting validations against reliable data sources.
As you work through the cleaning process, it's essential to track changes meticulously. Maintaining a log of modifications made helps in auditing future adjustments and aids in the validation of data quality improvements. This logging process also serves as a reference for continual improvement initiatives in data management practices.
Tools and Techniques for Data Cleaning
In modern data cleaning, leveraging the right tools and techniques can significantly enhance the efficiency and effectiveness of the cleaning process. The selection of tools should align with your project's objectives and the nature of the data being cleaned.
Establishing a toolkit will streamline processes and ensure that your team is well-equipped to tackle the various challenges that arise during data cleaning.
Manual Data Cleaning vs Automated Data Cleaning
When considering data cleaning methodologies, organizations often weigh the pros and cons of manual versus automated processes. Manual data cleaning may be necessary for smaller datasets or when human judgment is critical to resolving complex inconsistencies.
In contrast, automated data cleaning can significantly expedite the cleaning process, especially for larger datasets. Automated tools can efficiently flag errors, remove duplicates, and standardize formats, saving time and reducing the likelihood of human error. Finding the right balance between manual oversight and automation can yield optimal results.
Popular Data Cleaning Tools
There is a multitude of data cleaning tools available, each offering unique functionalities tailored to specific data management needs. Some commonly utilized tools in the field include OpenRefine, Talend, and Microsoft Excel’s data cleaning features.
Choosing the right tools involves assessing the specific requirements of your data cleaning project, including the complexity of the data, the volume of data, and the expertise of your team. Proper tool selection can significantly enhance the cleaning process, leading to better outcomes and more reliable data insights.
Ensuring the Quality of Cleaned Data
Once your data cleaning project is complete, the focus shifts to ensuring that the cleaned data maintains high quality over time. Data quality assurance should be an ongoing priority and incorporated into regular data management practices.
Establishing processes for regular audits and updates can help identify and rectify new issues as they arise, ensuring the longevity and utility of the cleaned data.
Validation Techniques for Cleaned Data
Validation techniques are critical for confirming the effectiveness of your data cleaning efforts. This may involve cross-referencing the cleaned data with external sources, conducting statistical analyses to identify anomalies, or employing data profiling metrics to gauge quality.
Regular validation not only affirms the reliability of the cleaned data but also instills confidence among stakeholders relying on this data for crucial business decisions.
Maintaining Data Quality Over Time
Finally, sustained efforts are required to maintain data quality over time. Implementing a data governance framework can help ensure that data quality guidelines are continuously followed and updated as needed.
Training staff on best practices for data entry and management can also mitigate many issues that lead to data decay. Regular monitoring and feedback loops can create a culture of data stewardship, ensuring ongoing commitment to data quality across the organization.
Ready to elevate your data cleaning project to the next level? CastorDoc is here to transform the way you manage and utilize your data. With our advanced governance, cataloging, and lineage capabilities, coupled with a user-friendly AI assistant, CastorDoc is the ultimate tool for businesses seeking to enable self-service analytics and maintain impeccable data quality. Don't let data challenges hinder your organization's potential. Try CastorDoc today and experience the power of efficient data management and insightful analytics at your fingertips.
You might also like
Get in Touch to Learn More
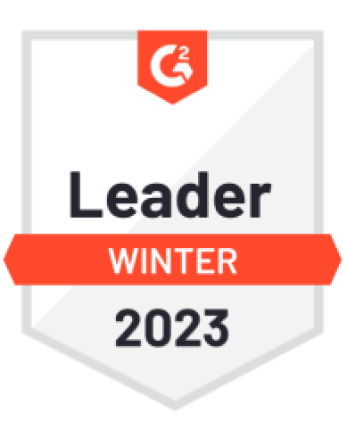
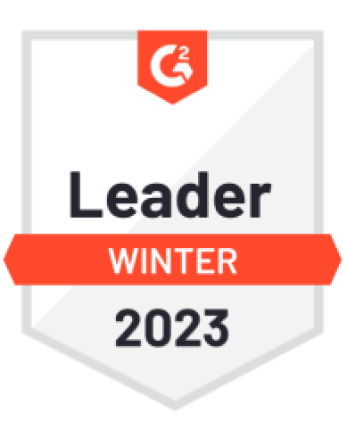

“[I like] The easy to use interface and the speed of finding the relevant assets that you're looking for in your database. I also really enjoy the score given to each table, [which] lets you prioritize the results of your queries by how often certain data is used.” - Michal P., Head of Data